Stark: Social Long-Term Multi-Modal Conversation with Persona Commonsense Knowledge
Young-Jun Lee, Dokyong Lee, Junyoung Youn, Kyeongjin Oh, Byungsoo Ko, Jonghwan Hyeon, Ho-Jin Choi
2024-07-08
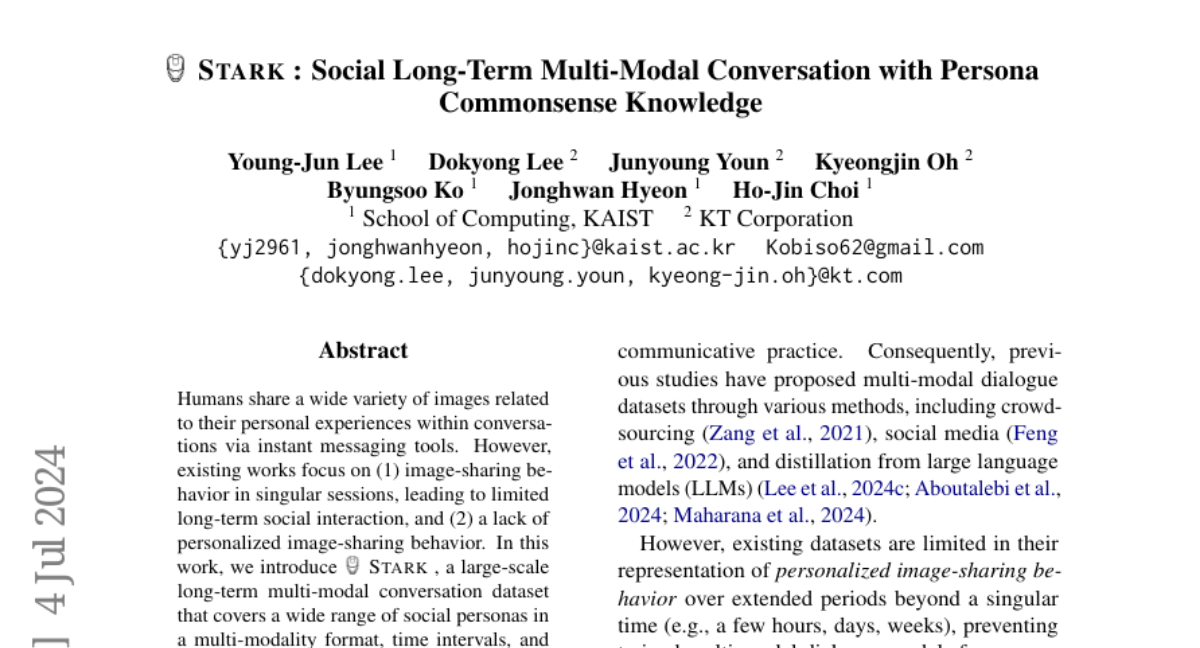
Summary
This paper talks about Stark, a new dataset designed to improve how AI systems understand and engage in long-term conversations that involve sharing images. It focuses on capturing personal experiences and social interactions over time.
What's the problem?
The main problem is that current research on multi-modal conversations (which include both text and images) often looks at short, single interactions. This means they miss out on how people share images and communicate over longer periods. Additionally, existing datasets do not account for the personalization of image-sharing, which is important for making conversations feel more relatable and engaging.
What's the solution?
To address these issues, the authors created Stark, a large-scale dataset that includes a variety of social personas and captures long-term interactions in conversations. They developed a new framework called Mcu (Multi-modal Contextualization Unit) that automatically generates dialogue using ChatGPT and an image aligner that helps select relevant images. This allows the dataset to reflect real-life conversations more accurately by including diverse time intervals and personalized image-sharing moments.
Why it matters?
This research is important because it enhances the ability of AI systems to engage in more realistic and meaningful conversations. By focusing on long-term interactions and personalization, Stark can help improve applications like chatbots, virtual assistants, and other AI-driven communication tools, making them more effective in understanding human behavior and preferences.
Abstract
Humans share a wide variety of images related to their personal experiences within conversations via instant messaging tools. However, existing works focus on (1) image-sharing behavior in singular sessions, leading to limited long-term social interaction, and (2) a lack of personalized image-sharing behavior. In this work, we introduce Stark, a large-scale long-term multi-modal conversation dataset that covers a wide range of social personas in a multi-modality format, time intervals, and images. To construct Stark automatically, we propose a novel multi-modal contextualization framework, Mcu, that generates long-term multi-modal dialogue distilled from ChatGPT and our proposed Plan-and-Execute image aligner. Using our Stark, we train a multi-modal conversation model, Ultron 7B, which demonstrates impressive visual imagination ability. Furthermore, we demonstrate the effectiveness of our dataset in human evaluation. We make our source code and dataset publicly available.