Step-DPO: Step-wise Preference Optimization for Long-chain Reasoning of LLMs
Xin Lai, Zhuotao Tian, Yukang Chen, Senqiao Yang, Xiangru Peng, Jiaya Jia
2024-06-28
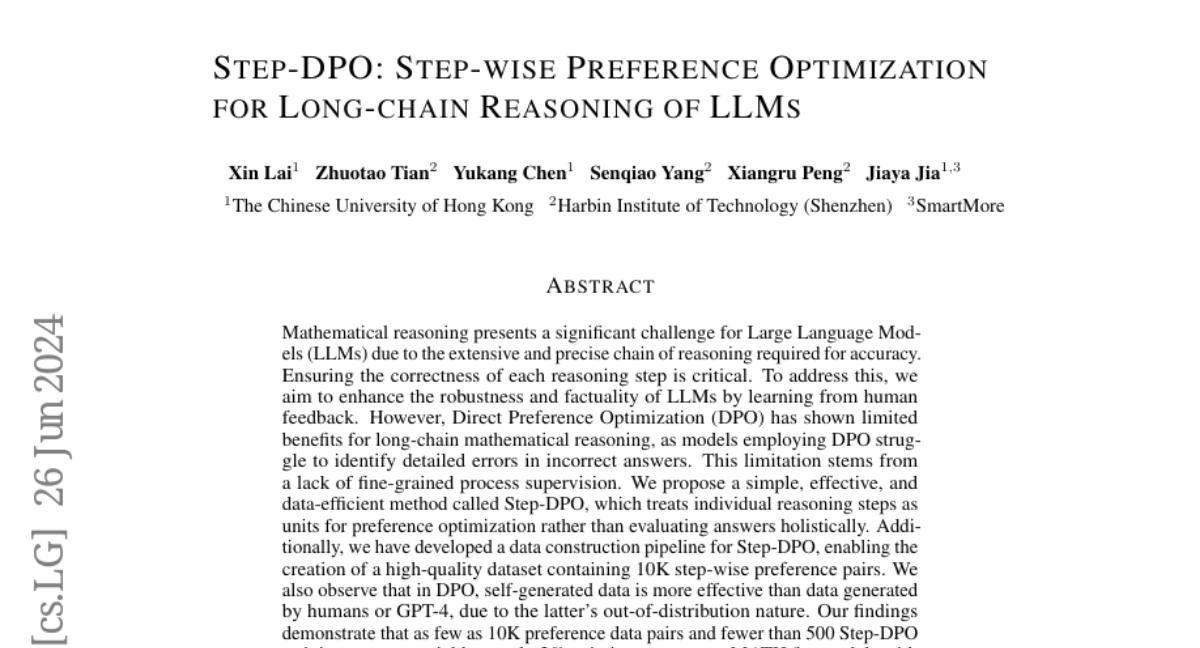
Summary
This paper talks about Step-DPO, a new method designed to improve how large language models (LLMs) handle mathematical reasoning. It focuses on optimizing individual reasoning steps to enhance the accuracy of these models when solving complex math problems.
What's the problem?
Mathematical reasoning is challenging for LLMs because it requires a series of precise steps to arrive at the correct answer. Existing methods, like Direct Preference Optimization (DPO), have not been very effective for long-chain reasoning tasks because they struggle to identify specific errors in the reasoning process. This is mainly due to a lack of detailed guidance on each step of the reasoning, which makes it hard for the models to learn from their mistakes.
What's the solution?
To address this issue, the authors introduced Step-DPO, which treats each individual reasoning step as a separate unit for optimization rather than looking at the entire answer as a whole. This approach allows for more focused learning from human feedback. They also created a data construction pipeline that generates a high-quality dataset with 10,000 pairs of step-wise preferences, helping the model learn more effectively. Their experiments showed that using this method can improve accuracy by nearly 3% on math tasks with fewer than 500 training steps.
Why it matters?
This research is important because it provides a more effective way for LLMs to learn and improve their mathematical reasoning skills. By focusing on individual steps in the reasoning process, Step-DPO can help these models become more accurate and reliable in solving complex math problems. This advancement could lead to better performance in educational tools, tutoring systems, and any applications that rely on accurate mathematical reasoning.
Abstract
Mathematical reasoning presents a significant challenge for Large Language Models (LLMs) due to the extensive and precise chain of reasoning required for accuracy. Ensuring the correctness of each reasoning step is critical. To address this, we aim to enhance the robustness and factuality of LLMs by learning from human feedback. However, Direct Preference Optimization (DPO) has shown limited benefits for long-chain mathematical reasoning, as models employing DPO struggle to identify detailed errors in incorrect answers. This limitation stems from a lack of fine-grained process supervision. We propose a simple, effective, and data-efficient method called Step-DPO, which treats individual reasoning steps as units for preference optimization rather than evaluating answers holistically. Additionally, we have developed a data construction pipeline for Step-DPO, enabling the creation of a high-quality dataset containing 10K step-wise preference pairs. We also observe that in DPO, self-generated data is more effective than data generated by humans or GPT-4, due to the latter's out-of-distribution nature. Our findings demonstrate that as few as 10K preference data pairs and fewer than 500 Step-DPO training steps can yield a nearly 3% gain in accuracy on MATH for models with over 70B parameters. Notably, Step-DPO, when applied to Qwen2-72B-Instruct, achieves scores of 70.8% and 94.0% on the test sets of MATH and GSM8K, respectively, surpassing a series of closed-source models, including GPT-4-1106, Claude-3-Opus, and Gemini-1.5-Pro. Our code, data, and models are available at https://github.com/dvlab-research/Step-DPO.