Story-Adapter: A Training-free Iterative Framework for Long Story Visualization
Jiawei Mao, Xiaoke Huang, Yunfei Xie, Yuanqi Chang, Mude Hui, Bingjie Xu, Yuyin Zhou
2024-10-10
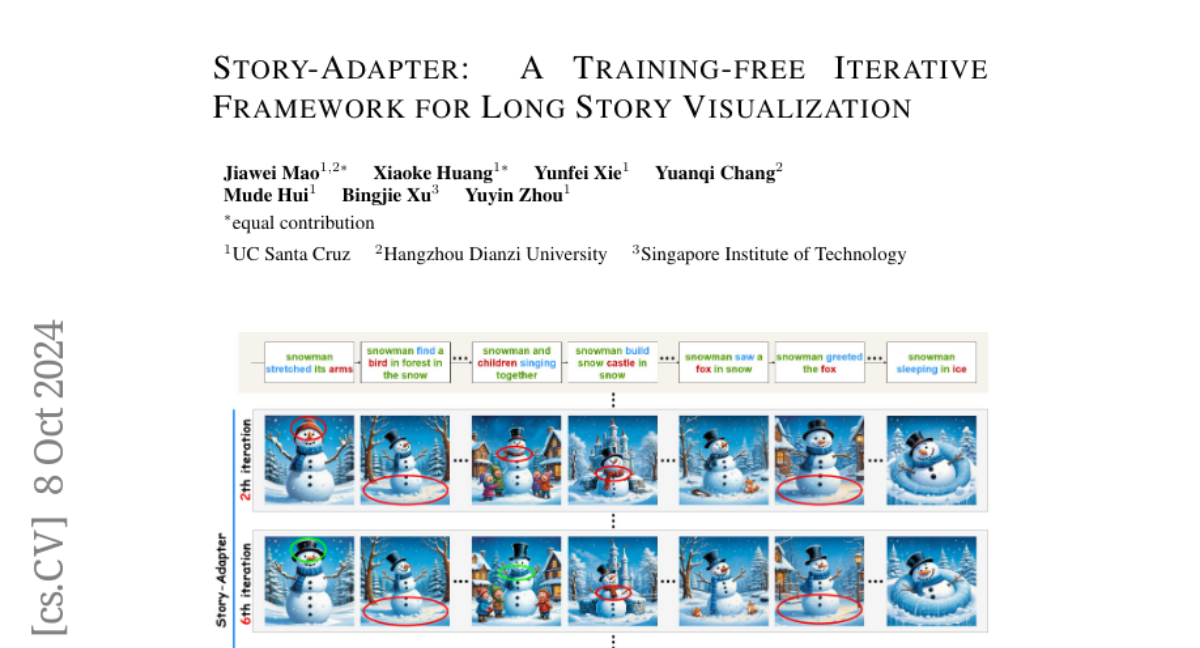
Summary
This paper presents Story-Adapter, a new framework designed to create visual representations of long stories without needing extensive training for the models involved.
What's the problem?
Generating images that accurately reflect long narratives can be challenging. Existing methods often struggle to maintain consistency across many images and can be computationally expensive, especially when creating up to 100 frames for a single story.
What's the solution?
The authors propose Story-Adapter, which allows for an iterative process where each generated image is refined based on the text prompt and previous images. This framework includes a special module that helps keep the story's meaning consistent while reducing the computational load. By using this method, the model can gradually improve the quality of the images it generates, resulting in better visual storytelling.
Why it matters?
This research is important because it simplifies the process of creating coherent visual narratives from text. By making it easier and more efficient to visualize long stories, Story-Adapter can be useful in various fields such as filmmaking, education, and content creation, allowing more people to tell their stories visually.
Abstract
Story visualization, the task of generating coherent images based on a narrative, has seen significant advancements with the emergence of text-to-image models, particularly diffusion models. However, maintaining semantic consistency, generating high-quality fine-grained interactions, and ensuring computational feasibility remain challenging, especially in long story visualization (i.e., up to 100 frames). In this work, we propose a training-free and computationally efficient framework, termed Story-Adapter, to enhance the generative capability of long stories. Specifically, we propose an iterative paradigm to refine each generated image, leveraging both the text prompt and all generated images from the previous iteration. Central to our framework is a training-free global reference cross-attention module, which aggregates all generated images from the previous iteration to preserve semantic consistency across the entire story, while minimizing computational costs with global embeddings. This iterative process progressively optimizes image generation by repeatedly incorporating text constraints, resulting in more precise and fine-grained interactions. Extensive experiments validate the superiority of Story-Adapter in improving both semantic consistency and generative capability for fine-grained interactions, particularly in long story scenarios. The project page and associated code can be accessed via https://jwmao1.github.io/storyadapter .