StoryMaker: Towards Holistic Consistent Characters in Text-to-image Generation
Zhengguang Zhou, Jing Li, Huaxia Li, Nemo Chen, Xu Tang
2024-09-20
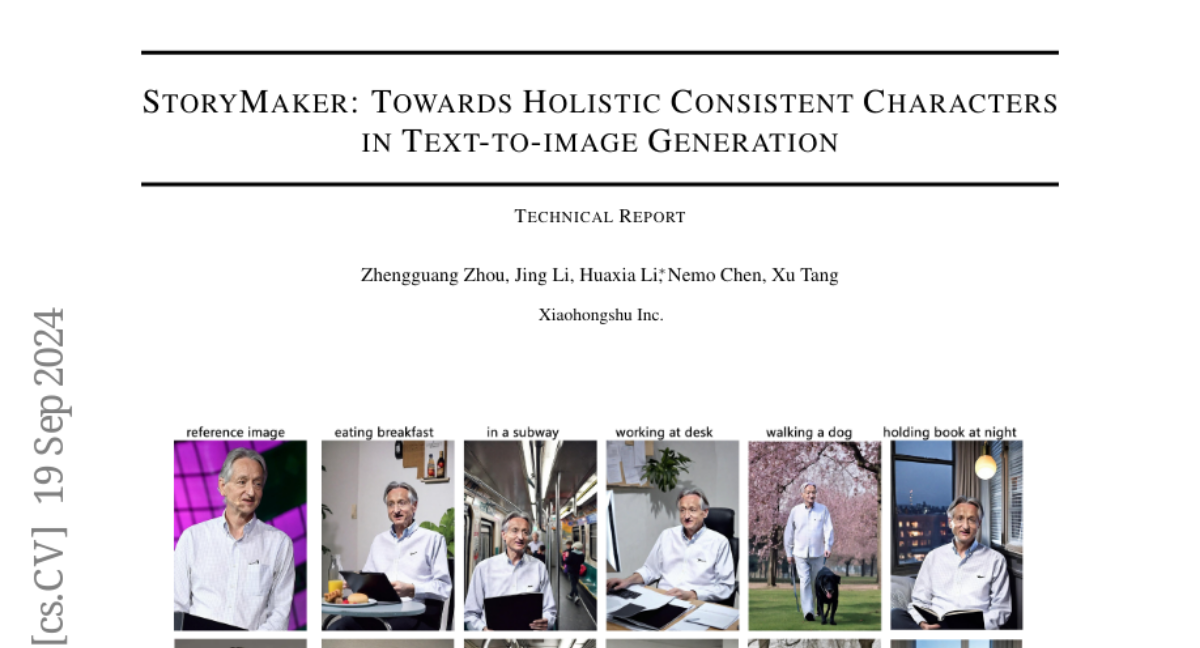
Summary
This paper presents StoryMaker, a new approach to generating images from text that ensures characters remain consistent in their appearance across multiple images, including their faces, clothing, hairstyles, and bodies.
What's the problem?
Current methods for generating images often focus on keeping faces consistent but struggle to maintain other important details like clothing and hairstyles when creating scenes with multiple characters. This lack of overall consistency can make it hard to tell a coherent story through a series of images.
What's the solution?
StoryMaker addresses this issue by using advanced techniques to ensure that all aspects of a character's appearance are consistent. It combines facial identity information with cropped images of the characters, using a special method called the Positional-aware Perceiver Resampler (PPR) to create distinct features for each character. Additionally, it employs a technique to separate the attention given to characters from the background, preventing them from blending together. The model is also trained to handle different poses while maintaining character consistency. Overall, StoryMaker enhances the quality of generated images and helps create a cohesive narrative.
Why it matters?
This research is important because it improves how AI can generate images for storytelling, comics, and other visual content where character consistency is crucial. By ensuring that characters look the same across different scenes, StoryMaker opens up new possibilities for creating engaging and coherent visual narratives.
Abstract
Tuning-free personalized image generation methods have achieved significant success in maintaining facial consistency, i.e., identities, even with multiple characters. However, the lack of holistic consistency in scenes with multiple characters hampers these methods' ability to create a cohesive narrative. In this paper, we introduce StoryMaker, a personalization solution that preserves not only facial consistency but also clothing, hairstyles, and body consistency, thus facilitating the creation of a story through a series of images. StoryMaker incorporates conditions based on face identities and cropped character images, which include clothing, hairstyles, and bodies. Specifically, we integrate the facial identity information with the cropped character images using the Positional-aware Perceiver Resampler (PPR) to obtain distinct character features. To prevent intermingling of multiple characters and the background, we separately constrain the cross-attention impact regions of different characters and the background using MSE loss with segmentation masks. Additionally, we train the generation network conditioned on poses to promote decoupling from poses. A LoRA is also employed to enhance fidelity and quality. Experiments underscore the effectiveness of our approach. StoryMaker supports numerous applications and is compatible with other societal plug-ins. Our source codes and model weights are available at https://github.com/RedAIGC/StoryMaker.