StyleStudio: Text-Driven Style Transfer with Selective Control of Style Elements
Mingkun Lei, Xue Song, Beier Zhu, Hao Wang, Chi Zhang
2024-12-12
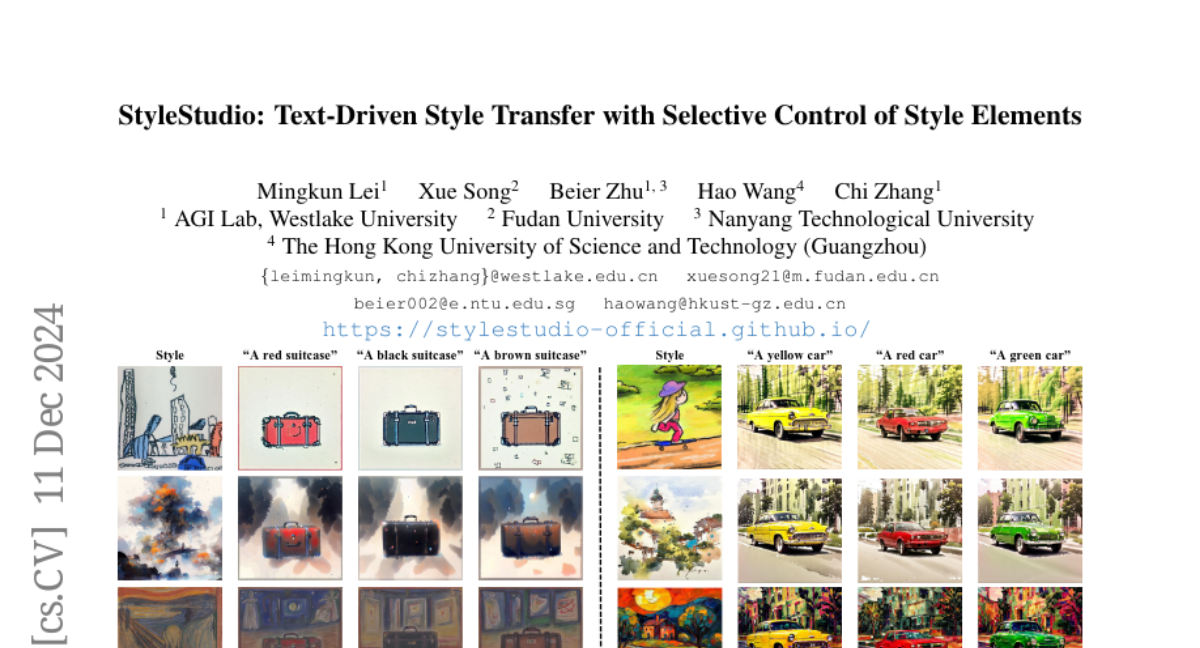
Summary
This paper presents StyleStudio, a method for transferring the style of one image to another based on a text description. It improves how styles are applied to images while allowing users to control specific style elements more effectively.
What's the problem?
While recent advancements in technology have made it easier to combine styles from reference images with content described in text, there are still challenges. These include models getting too attached to the reference styles, limited control over which style elements are used, and difficulties in making sure the final image matches the text prompt accurately.
What's the solution?
To tackle these issues, the authors propose three main strategies. First, they introduce a new technique called Adaptive Instance Normalization (AdaIN) that better combines style and text features. Second, they create a method called Style-based Classifier-Free Guidance (SCFG) that allows users to selectively control which style elements to emphasize, reducing unwanted influences. Lastly, they use a teacher model during the early stages of video generation to maintain stability and reduce errors in the final output. These improvements lead to better quality in style transfer and alignment with the text prompts.
Why it matters?
This research is important because it enhances the ability to create visually appealing images that accurately reflect both the desired style and content. By allowing for more precise control over stylistic elements, StyleStudio can benefit artists, designers, and anyone interested in creating customized images with ease.
Abstract
Text-driven style transfer aims to merge the style of a reference image with content described by a text prompt. Recent advancements in text-to-image models have improved the nuance of style transformations, yet significant challenges remain, particularly with overfitting to reference styles, limiting stylistic control, and misaligning with textual content. In this paper, we propose three complementary strategies to address these issues. First, we introduce a cross-modal Adaptive Instance Normalization (AdaIN) mechanism for better integration of style and text features, enhancing alignment. Second, we develop a Style-based Classifier-Free Guidance (SCFG) approach that enables selective control over stylistic elements, reducing irrelevant influences. Finally, we incorporate a teacher model during early generation stages to stabilize spatial layouts and mitigate artifacts. Our extensive evaluations demonstrate significant improvements in style transfer quality and alignment with textual prompts. Furthermore, our approach can be integrated into existing style transfer frameworks without fine-tuning.