SuperCorrect: Supervising and Correcting Language Models with Error-Driven Insights
Ling Yang, Zhaochen Yu, Tianjun Zhang, Minkai Xu, Joseph E. Gonzalez, Bin Cui, Shuicheng Yan
2024-10-14
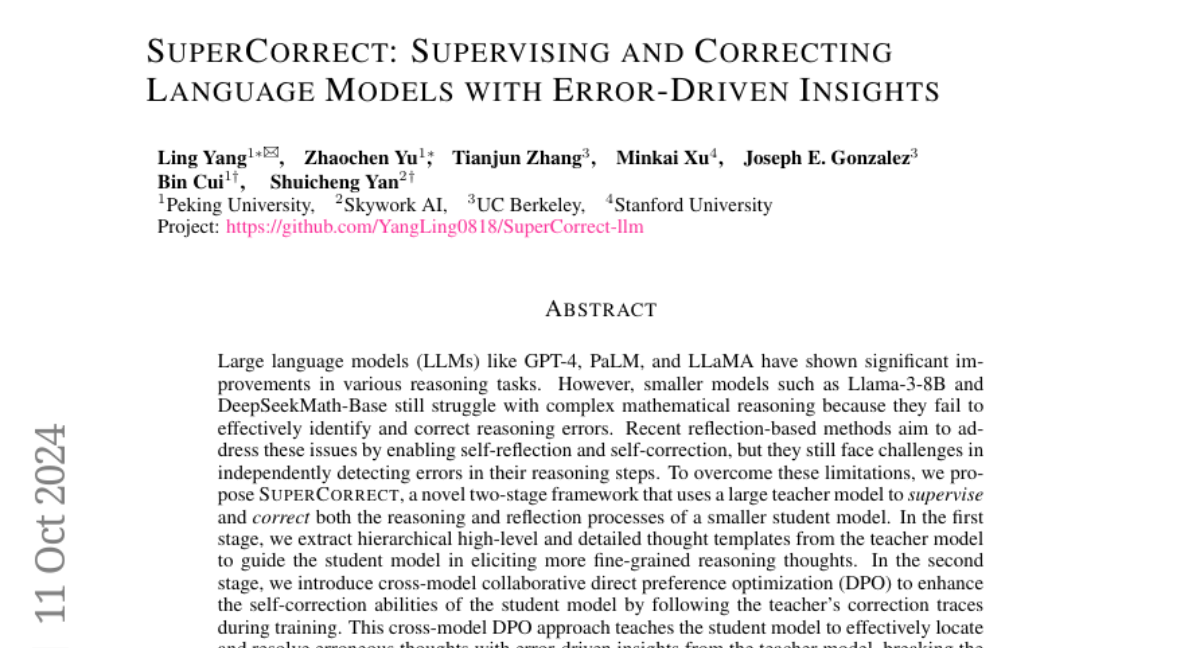
Summary
This paper discusses SuperCorrect, a new framework designed to help smaller language models improve their reasoning abilities by learning from larger, more advanced models.
What's the problem?
While large language models like GPT-4 and PaLM perform well in reasoning tasks, smaller models often struggle with complex problems, especially in mathematics. They have difficulty identifying and correcting their own mistakes, which limits their effectiveness.
What's the solution?
SuperCorrect introduces a two-stage approach where a larger 'teacher' model supervises and corrects a smaller 'student' model. In the first stage, the teacher model provides structured templates to guide the student in better reasoning. In the second stage, the student learns to self-correct by following the teacher's corrections during training. This method helps the student model recognize and fix its errors more effectively.
Why it matters?
This research is important because it enhances the capabilities of smaller language models, making them more competitive with larger models. By improving their reasoning skills, SuperCorrect can lead to better performance in various applications, such as educational tools and automated problem-solving systems.
Abstract
Large language models (LLMs) like GPT-4, PaLM, and LLaMA have shown significant improvements in various reasoning tasks. However, smaller models such as Llama-3-8B and DeepSeekMath-Base still struggle with complex mathematical reasoning because they fail to effectively identify and correct reasoning errors. Recent reflection-based methods aim to address these issues by enabling self-reflection and self-correction, but they still face challenges in independently detecting errors in their reasoning steps. To overcome these limitations, we propose SuperCorrect, a novel two-stage framework that uses a large teacher model to supervise and correct both the reasoning and reflection processes of a smaller student model. In the first stage, we extract hierarchical high-level and detailed thought templates from the teacher model to guide the student model in eliciting more fine-grained reasoning thoughts. In the second stage, we introduce cross-model collaborative direct preference optimization (DPO) to enhance the self-correction abilities of the student model by following the teacher's correction traces during training. This cross-model DPO approach teaches the student model to effectively locate and resolve erroneous thoughts with error-driven insights from the teacher model, breaking the bottleneck of its thoughts and acquiring new skills and knowledge to tackle challenging problems. Extensive experiments consistently demonstrate our superiority over previous methods. Notably, our SuperCorrect-7B model significantly surpasses powerful DeepSeekMath-7B by 7.8%/5.3% and Qwen2.5-Math-7B by 15.1%/6.3% on MATH/GSM8K benchmarks, achieving new SOTA performance among all 7B models. Code: https://github.com/YangLing0818/SuperCorrect-llm