SVG: 3D Stereoscopic Video Generation via Denoising Frame Matrix
Peng Dai, Feitong Tan, Qiangeng Xu, David Futschik, Ruofei Du, Sean Fanello, Xiaojuan Qi, Yinda Zhang
2024-07-02
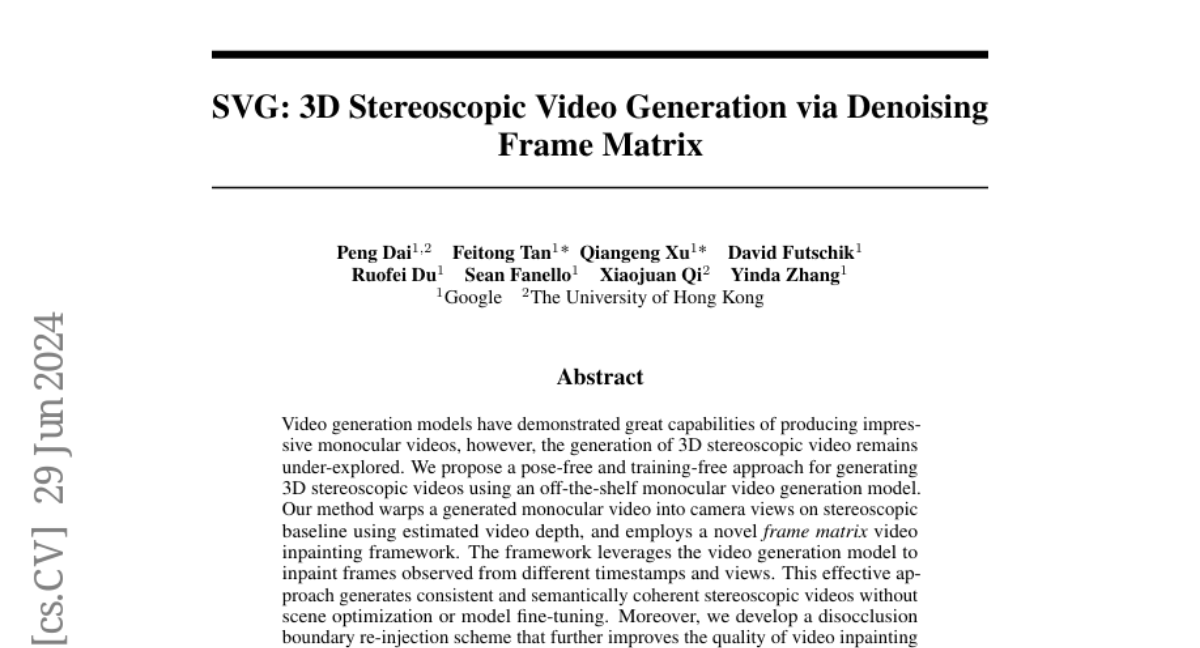
Summary
This paper talks about a new method for creating 3D stereoscopic videos from regular 2D videos without needing complex setups or extra training. It uses existing video generation models to produce high-quality 3D content.
What's the problem?
While video generation technology has advanced, creating 3D stereoscopic videos (which provide depth perception) is still a challenge. Many existing methods require detailed camera positioning and fine-tuning, which can be complicated and time-consuming. This makes it hard to generate high-quality 3D videos reliably, especially from standard 2D sources.
What's the solution?
To solve this problem, the authors developed a method that does not require camera pose estimation or extensive training. Instead, they use a technique that takes a regular 2D video and estimates its depth to create two views (left and right) needed for stereoscopic video. They also introduced a frame matrix video inpainting framework that fills in missing parts of the video based on different timestamps and views. This approach ensures that the generated 3D videos are consistent and visually coherent without needing complicated adjustments.
Why it matters?
This research is important because it simplifies the process of creating immersive 3D videos, making it more accessible for various applications like gaming, virtual reality, and film production. By improving how we can generate high-quality stereoscopic content from standard videos, this method could enhance the way we experience digital media and entertainment.
Abstract
Video generation models have demonstrated great capabilities of producing impressive monocular videos, however, the generation of 3D stereoscopic video remains under-explored. We propose a pose-free and training-free approach for generating 3D stereoscopic videos using an off-the-shelf monocular video generation model. Our method warps a generated monocular video into camera views on stereoscopic baseline using estimated video depth, and employs a novel frame matrix video inpainting framework. The framework leverages the video generation model to inpaint frames observed from different timestamps and views. This effective approach generates consistent and semantically coherent stereoscopic videos without scene optimization or model fine-tuning. Moreover, we develop a disocclusion boundary re-injection scheme that further improves the quality of video inpainting by alleviating the negative effects propagated from disoccluded areas in the latent space. We validate the efficacy of our proposed method by conducting experiments on videos from various generative models, including Sora [4 ], Lumiere [2], WALT [8 ], and Zeroscope [ 42]. The experiments demonstrate that our method has a significant improvement over previous methods. The code will be released at https://daipengwa.github.io/SVG_ProjectPage.