Swan and ArabicMTEB: Dialect-Aware, Arabic-Centric, Cross-Lingual, and Cross-Cultural Embedding Models and Benchmarks
Gagan Bhatia, El Moatez Billah Nagoudi, Abdellah El Mekki, Fakhraddin Alwajih, Muhammad Abdul-Mageed
2024-11-05
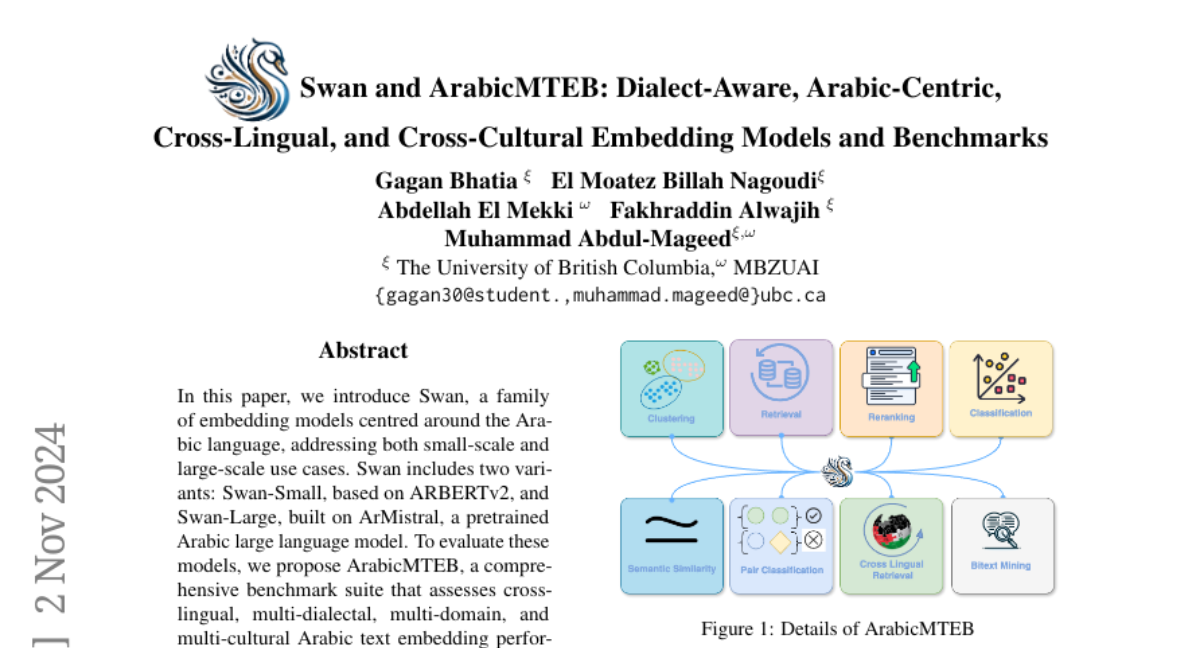
Summary
This paper introduces Swan, a set of advanced models designed specifically for understanding and processing the Arabic language, along with a benchmark called ArabicMTEB to evaluate their performance across various tasks.
What's the problem?
Arabic language processing faces challenges due to its many dialects and cultural contexts, making it difficult for existing models to accurately understand and generate Arabic text. This lack of effective tools can hinder advancements in natural language processing for Arabic speakers.
What's the solution?
The researchers created two versions of the Swan model: Swan-Small and Swan-Large, tailored for different scales of use. They also developed ArabicMTEB, a benchmark that tests these models on eight different tasks using 94 datasets, ensuring they perform well across various Arabic dialects and cultural contexts. The Swan models outperformed other existing models in many tasks, showcasing their effectiveness.
Why it matters?
This work is significant because it enhances the ability to process Arabic text accurately, which is crucial for applications like translation, sentiment analysis, and more. By improving these technologies, it can lead to better communication and understanding in diverse Arabic-speaking communities.
Abstract
We introduce Swan, a family of embedding models centred around the Arabic language, addressing both small-scale and large-scale use cases. Swan includes two variants: Swan-Small, based on ARBERTv2, and Swan-Large, built on ArMistral, a pretrained Arabic large language model. To evaluate these models, we propose ArabicMTEB, a comprehensive benchmark suite that assesses cross-lingual, multi-dialectal, multi-domain, and multi-cultural Arabic text embedding performance, covering eight diverse tasks and spanning 94 datasets. Swan-Large achieves state-of-the-art results, outperforming Multilingual-E5-large in most Arabic tasks, while the Swan-Small consistently surpasses Multilingual-E5 base. Our extensive evaluations demonstrate that Swan models are both dialectally and culturally aware, excelling across various Arabic domains while offering significant monetary efficiency. This work significantly advances the field of Arabic language modelling and provides valuable resources for future research and applications in Arabic natural language processing. Our models and benchmark will be made publicly accessible for research.