Synth-SONAR: Sonar Image Synthesis with Enhanced Diversity and Realism via Dual Diffusion Models and GPT Prompting
Purushothaman Natarajan, Kamal Basha, Athira Nambiar
2024-10-14
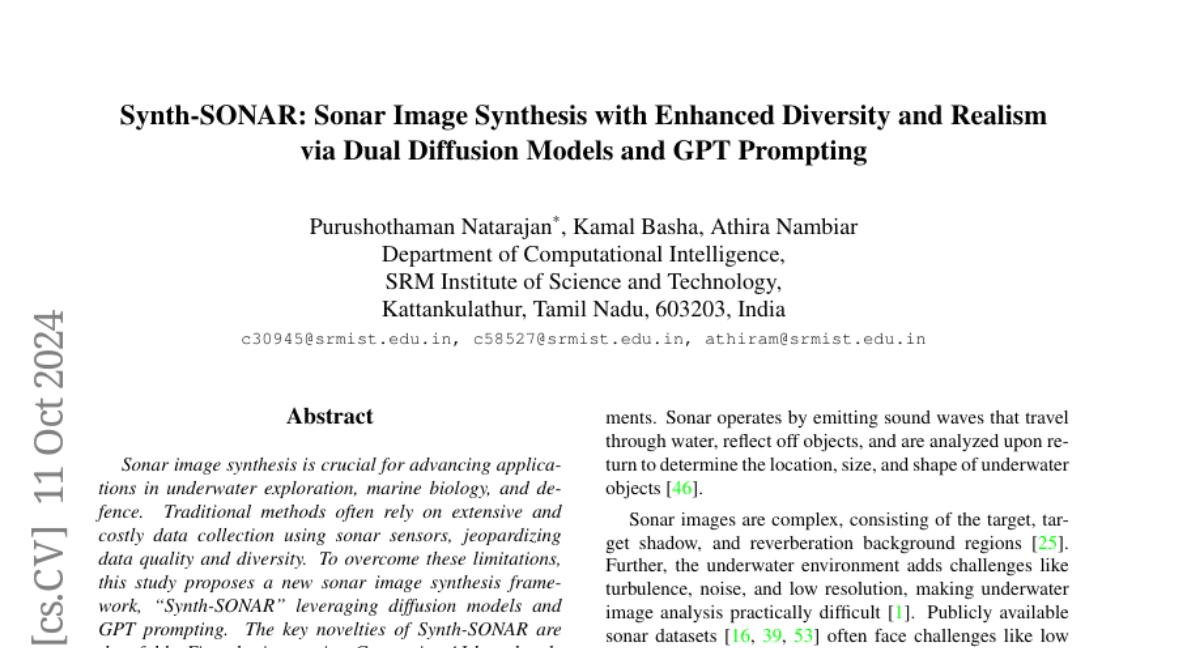
Summary
This paper discusses Synth-SONAR, a new system designed to create realistic sonar images using advanced AI techniques, which can help in underwater exploration and other applications.
What's the problem?
Traditional methods of creating sonar images require a lot of time and money to collect data using sonar sensors. This can lead to problems with the quality and variety of the images produced, making it hard to get accurate information about underwater environments.
What's the solution?
Synth-SONAR addresses these issues by using a combination of diffusion models and GPT prompting to generate sonar images. It creates a large dataset by mixing real and simulated sonar data, which helps improve the diversity and realism of the generated images. The system works in three phases: first, it gathers data; second, it generates initial (coarse) images; and third, it refines these images into detailed outputs using advanced AI techniques.
Why it matters?
This research is important because it provides a more efficient way to create high-quality sonar images without needing extensive data collection. By improving how sonar images are generated, Synth-SONAR can enhance various fields such as marine biology, underwater exploration, and defense, making it easier to analyze and understand underwater environments.
Abstract
Sonar image synthesis is crucial for advancing applications in underwater exploration, marine biology, and defence. Traditional methods often rely on extensive and costly data collection using sonar sensors, jeopardizing data quality and diversity. To overcome these limitations, this study proposes a new sonar image synthesis framework, Synth-SONAR leveraging diffusion models and GPT prompting. The key novelties of Synth-SONAR are threefold: First, by integrating Generative AI-based style injection techniques along with publicly available real/simulated data, thereby producing one of the largest sonar data corpus for sonar research. Second, a dual text-conditioning sonar diffusion model hierarchy synthesizes coarse and fine-grained sonar images with enhanced quality and diversity. Third, high-level (coarse) and low-level (detailed) text-based sonar generation methods leverage advanced semantic information available in visual language models (VLMs) and GPT-prompting. During inference, the method generates diverse and realistic sonar images from textual prompts, bridging the gap between textual descriptions and sonar image generation. This marks the application of GPT-prompting in sonar imagery for the first time, to the best of our knowledge. Synth-SONAR achieves state-of-the-art results in producing high-quality synthetic sonar datasets, significantly enhancing their diversity and realism.