SynthLight: Portrait Relighting with Diffusion Model by Learning to Re-render Synthetic Faces
Sumit Chaturvedi, Mengwei Ren, Yannick Hold-Geoffroy, Jingyuan Liu, Julie Dorsey, Zhixin Shu
2025-01-17
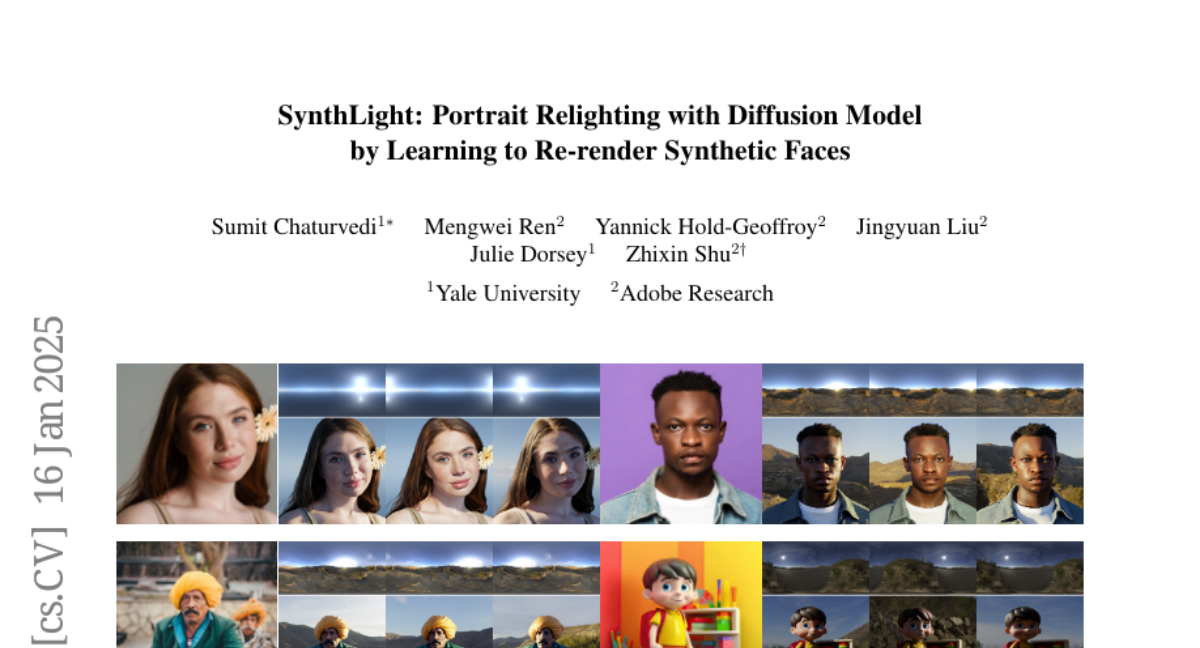
Summary
This paper talks about SynthLight, a new AI system that can change the lighting in portrait photos after they've been taken. It's like having a virtual photography studio where you can adjust the lights even after the picture is taken.
What's the problem?
Changing the lighting in a photo after it's been taken is really hard. Current methods often don't look realistic, especially when it comes to things like how light reflects off skin or creates shadows. It's especially tricky because the AI needs to understand how light works in the real world, which is complex.
What's the solution?
The researchers created SynthLight, which uses a type of AI called a diffusion model. They trained this AI by showing it lots of computer-generated 3D faces under different lighting conditions. To make it work well on real photos, they used two clever tricks: they included some real portraits in the training (even though they didn't know the original lighting), and they developed a special way to preserve the details of the original photo when changing the lighting.
Why it matters?
This matters because it could change how we take and edit photos. Imagine taking a selfie in bad lighting, then using AI to make it look like it was taken in a professional studio. It could be huge for photographers, social media users, and even the movie industry. Plus, the techniques they developed could help other AI systems better understand how light works in the real world, which could be useful for things like virtual reality or self-driving cars.
Abstract
We introduce SynthLight, a diffusion model for portrait relighting. Our approach frames image relighting as a re-rendering problem, where pixels are transformed in response to changes in environmental lighting conditions. Using a physically-based rendering engine, we synthesize a dataset to simulate this lighting-conditioned transformation with 3D head assets under varying lighting. We propose two training and inference strategies to bridge the gap between the synthetic and real image domains: (1) multi-task training that takes advantage of real human portraits without lighting labels; (2) an inference time diffusion sampling procedure based on classifier-free guidance that leverages the input portrait to better preserve details. Our method generalizes to diverse real photographs and produces realistic illumination effects, including specular highlights and cast shadows, while preserving the subject's identity. Our quantitative experiments on Light Stage data demonstrate results comparable to state-of-the-art relighting methods. Our qualitative results on in-the-wild images showcase rich and unprecedented illumination effects. Project Page: https://vrroom.github.io/synthlight/