Tabular Data Generation using Binary Diffusion
Vitaliy Kinakh, Slava Voloshynovskiy
2024-09-25
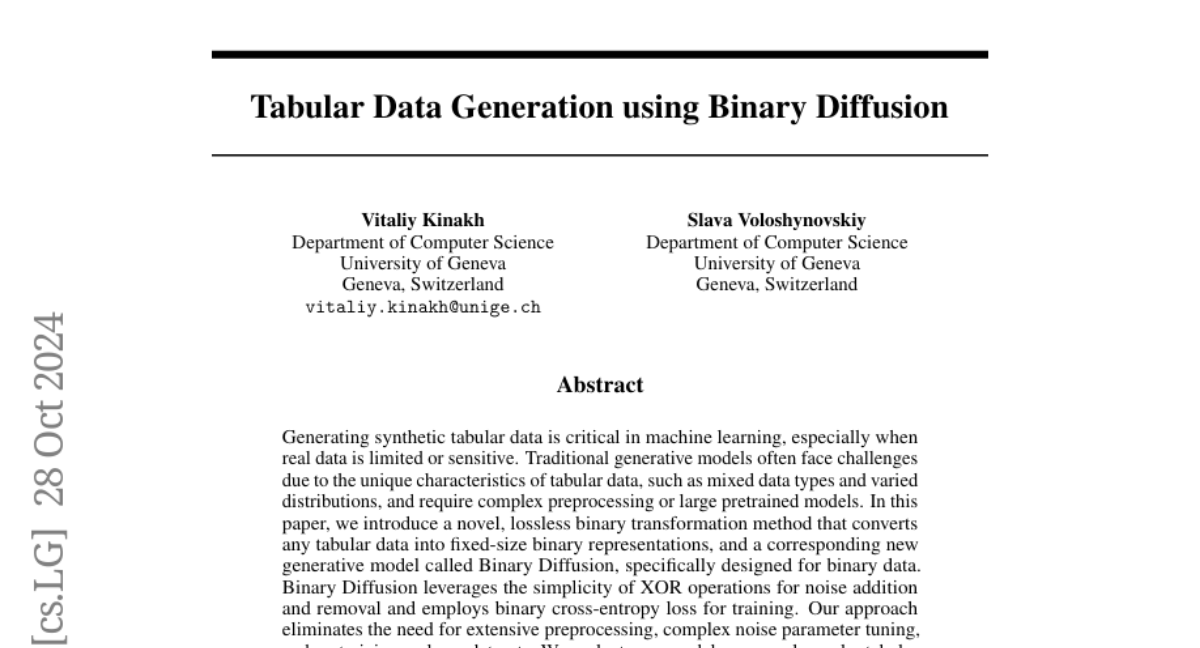
Summary
This paper discusses a new method for generating synthetic tabular data using a technique called Binary Diffusion. This approach helps create realistic data for machine learning applications, especially when real data is limited or sensitive.
What's the problem?
Generating synthetic tabular data is important for machine learning, but traditional methods struggle because tabular data can have different types of information (like numbers and categories) and various distributions. These methods often require a lot of preprocessing and large models, making them complex and inefficient.
What's the solution?
The authors introduce a novel method that transforms any tabular data into fixed-size binary representations, which simplifies the data structure. They developed a new generative model called Binary Diffusion that uses simple operations to add and remove noise from the data. This model does not need extensive preprocessing or large amounts of training data, making it easier to use. The experiments showed that Binary Diffusion performs better than existing methods on popular datasets like Travel, Adult Income, and Diabetes while being smaller in size.
Why it matters?
This research is significant because it provides a more efficient way to generate synthetic tabular data, which is crucial for various applications in machine learning. By simplifying the process and improving performance, this method can help researchers and companies create better models without needing large amounts of sensitive or hard-to-collect real-world data.
Abstract
Generating synthetic tabular data is critical in machine learning, especially when real data is limited or sensitive. Traditional generative models often face challenges due to the unique characteristics of tabular data, such as mixed data types and varied distributions, and require complex preprocessing or large pretrained models. In this paper, we introduce a novel, lossless binary transformation method that converts any tabular data into fixed-size binary representations, and a corresponding new generative model called Binary Diffusion, specifically designed for binary data. Binary Diffusion leverages the simplicity of XOR operations for noise addition and removal and employs binary cross-entropy loss for training. Our approach eliminates the need for extensive preprocessing, complex noise parameter tuning, and pretraining on large datasets. We evaluate our model on several popular tabular benchmark datasets, demonstrating that Binary Diffusion outperforms existing state-of-the-art models on Travel, Adult Income, and Diabetes datasets while being significantly smaller in size.