TacSL: A Library for Visuotactile Sensor Simulation and Learning
Iretiayo Akinola, Jie Xu, Jan Carius, Dieter Fox, Yashraj Narang
2024-08-14
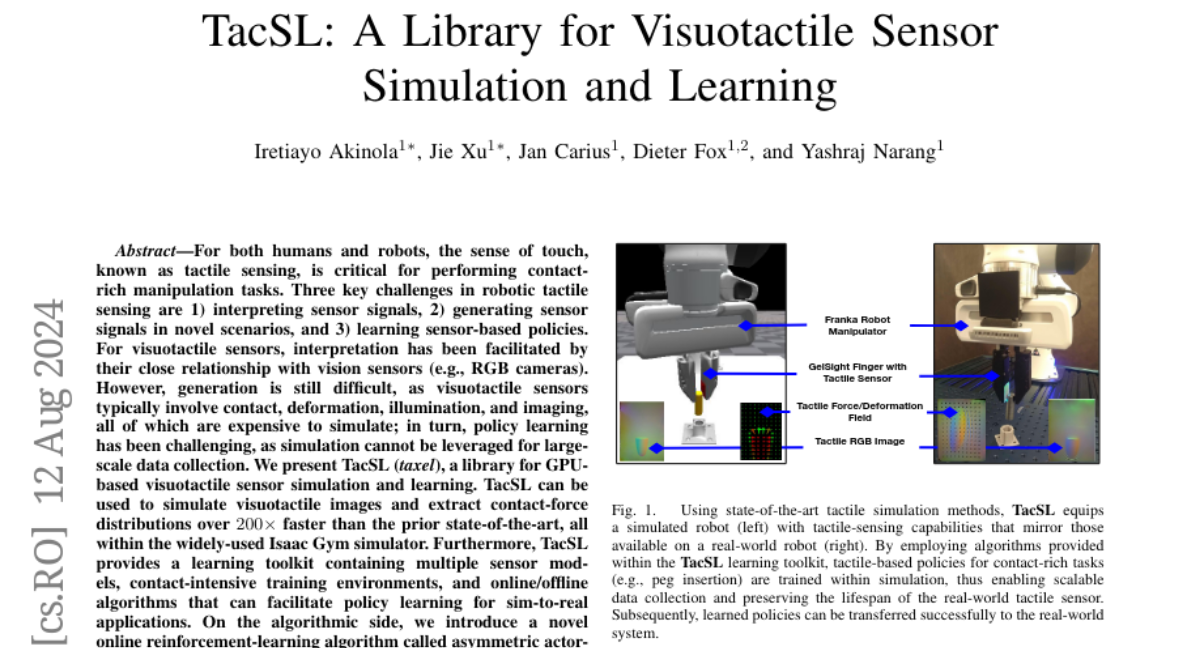
Summary
This paper introduces TacSL, a library designed for simulating and learning from visuotactile sensors, which are important for robots to effectively interact with objects through touch.
What's the problem?
Robots need to understand and use their sense of touch to perform tasks that involve contact with objects. However, there are three main challenges: interpreting the signals from their sensors, generating those signals in new situations, and learning how to use this sensory information to make decisions. Current methods for simulating these sensors can be slow and expensive, making it hard to train robots effectively.
What's the solution?
TacSL provides a fast and efficient way to simulate visuotactile sensors using GPU technology. It allows researchers to create realistic tactile images and measure contact forces quickly, over 200 times faster than previous methods. The library includes tools for training robots in virtual environments, enabling them to learn how to manipulate objects based on touch. Additionally, it features a new learning algorithm called asymmetric actor-critic distillation, which helps robots learn better policies for interacting with objects in the real world.
Why it matters?
This research is important because it helps improve how robots learn to use their sense of touch, making them more effective at handling and manipulating objects. By providing a robust simulation environment, TacSL accelerates the development of advanced robotic systems that can perform complex tasks in everyday situations, potentially leading to better applications in industries like manufacturing, healthcare, and service robotics.
Abstract
For both humans and robots, the sense of touch, known as tactile sensing, is critical for performing contact-rich manipulation tasks. Three key challenges in robotic tactile sensing are 1) interpreting sensor signals, 2) generating sensor signals in novel scenarios, and 3) learning sensor-based policies. For visuotactile sensors, interpretation has been facilitated by their close relationship with vision sensors (e.g., RGB cameras). However, generation is still difficult, as visuotactile sensors typically involve contact, deformation, illumination, and imaging, all of which are expensive to simulate; in turn, policy learning has been challenging, as simulation cannot be leveraged for large-scale data collection. We present TacSL (taxel), a library for GPU-based visuotactile sensor simulation and learning. TacSL can be used to simulate visuotactile images and extract contact-force distributions over 200times faster than the prior state-of-the-art, all within the widely-used Isaac Gym simulator. Furthermore, TacSL provides a learning toolkit containing multiple sensor models, contact-intensive training environments, and online/offline algorithms that can facilitate policy learning for sim-to-real applications. On the algorithmic side, we introduce a novel online reinforcement-learning algorithm called asymmetric actor-critic distillation (\sysName), designed to effectively and efficiently learn tactile-based policies in simulation that can transfer to the real world. Finally, we demonstrate the utility of our library and algorithms by evaluating the benefits of distillation and multimodal sensing for contact-rich manip ulation tasks, and most critically, performing sim-to-real transfer. Supplementary videos and results are at https://iakinola23.github.io/tacsl/.