Takin: A Cohort of Superior Quality Zero-shot Speech Generation Models
EverestAI, Sijin Chen, Yuan Feng, Laipeng He, Tianwei He, Wendi He, Yanni Hu, Bin Lin, Yiting Lin, Pengfei Tan, Chengwei Tian, Chen Wang, Zhicheng Wang, Ruoye Xie, Jingjing Yin, Jianhao Ye, Jixun Yao, Quanlei Yan, Yuguang Yang
2024-09-19
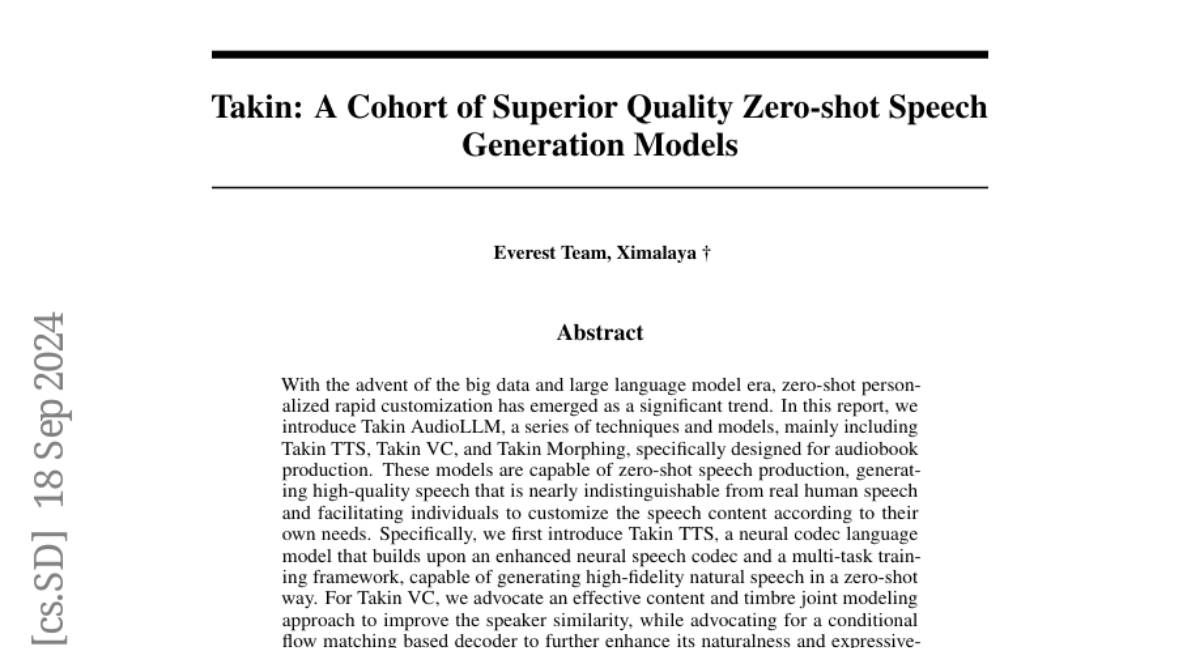
Summary
This paper introduces Takin, a series of advanced models designed for generating high-quality speech without needing specific training for each voice, making it ideal for creating audiobooks and other audio content.
What's the problem?
As the demand for personalized audio content grows, there is a need for models that can produce high-quality speech quickly and efficiently without requiring extensive data for each individual voice. Traditional methods often require training on specific voices, which is time-consuming and limits flexibility.
What's the solution?
The Takin series includes several models: Takin TTS (Text-to-Speech), Takin VC (Voice Conversion), and Takin Morphing. Takin TTS uses a neural codec to generate natural-sounding speech from text, while Takin VC focuses on changing the voice of the speech without altering its content. Takin Morphing allows users to customize the tone and style of the speech. These models are designed to work in a 'zero-shot' manner, meaning they can produce high-quality speech without needing prior training on specific voices. Extensive testing shows that these models perform very well across various tasks.
Why it matters?
This research is significant because it provides a powerful tool for creating personalized audio content quickly and efficiently. By enabling high-quality speech generation without specific training data, Takin can be used in applications like audiobooks, virtual assistants, and more, making it easier for creators to produce customized audio experiences.
Abstract
With the advent of the big data and large language model era, zero-shot personalized rapid customization has emerged as a significant trend. In this report, we introduce Takin AudioLLM, a series of techniques and models, mainly including Takin TTS, Takin VC, and Takin Morphing, specifically designed for audiobook production. These models are capable of zero-shot speech production, generating high-quality speech that is nearly indistinguishable from real human speech and facilitating individuals to customize the speech content according to their own needs. Specifically, we first introduce Takin TTS, a neural codec language model that builds upon an enhanced neural speech codec and a multi-task training framework, capable of generating high-fidelity natural speech in a zero-shot way. For Takin VC, we advocate an effective content and timbre joint modeling approach to improve the speaker similarity, while advocating for a conditional flow matching based decoder to further enhance its naturalness and expressiveness. Last, we propose the Takin Morphing system with highly decoupled and advanced timbre and prosody modeling approaches, which enables individuals to customize speech production with their preferred timbre and prosody in a precise and controllable manner. Extensive experiments validate the effectiveness and robustness of our Takin AudioLLM series models. For detailed demos, please refer to https://takinaudiollm.github.io.