TalkinNeRF: Animatable Neural Fields for Full-Body Talking Humans
Aggelina Chatziagapi, Bindita Chaudhuri, Amit Kumar, Rakesh Ranjan, Dimitris Samaras, Nikolaos Sarafianos
2024-09-26
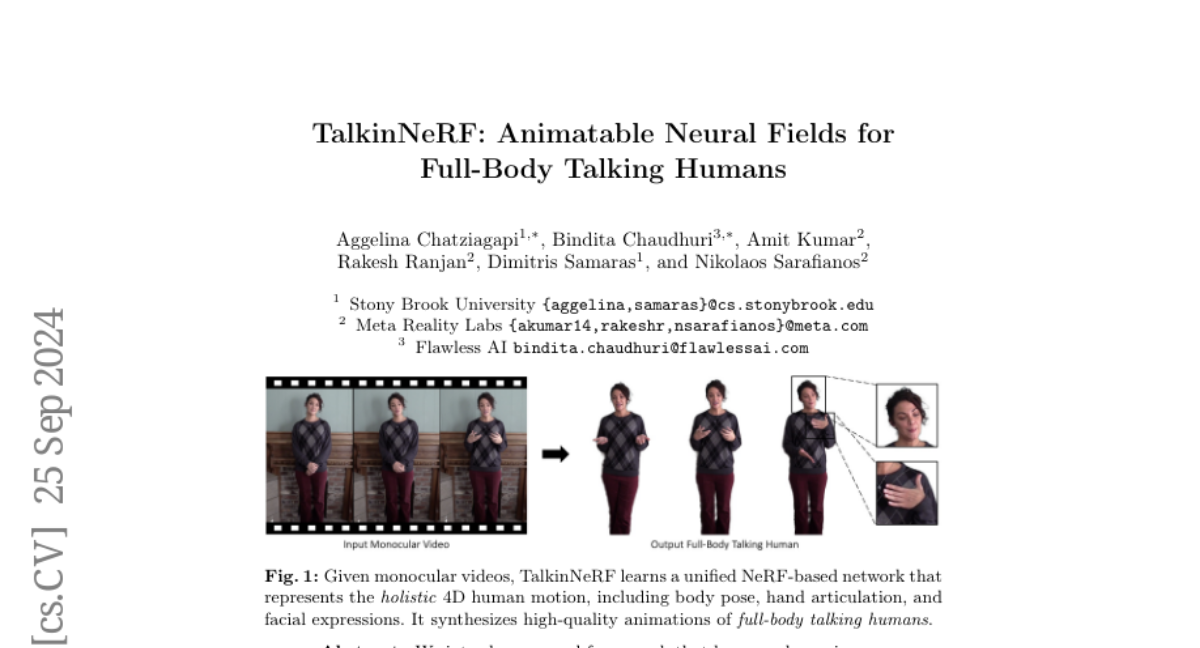
Summary
This paper introduces TalkinNeRF, a new framework that creates realistic animations of full-body talking humans using just regular videos. It captures the entire body's movements, including facial expressions and hand gestures, to produce lifelike animations.
What's the problem?
Previous methods for animating humans from video often focused only on specific parts, like the face or body pose, which doesn't fully represent how people communicate. Humans use their entire bodies to express themselves, so relying on partial representations can lead to unnatural animations that lack realism and expressiveness.
What's the solution?
TalkinNeRF addresses this issue by using a unified system that learns from monocular videos (videos taken from a single camera angle) to capture the complete motion of the body, face, and hands. The framework combines different modules for each body part and includes a special technique to accurately animate complex finger movements. This allows it to create detailed and expressive animations of talking humans that can adapt to new poses and identities based on just a short video input.
Why it matters?
This research is significant because it enhances the ability to create realistic digital humans for various applications, such as virtual assistants, video games, and animated films. By capturing full-body movements and expressions, TalkinNeRF can help produce more engaging and lifelike characters that improve human-computer interaction and storytelling.
Abstract
We introduce a novel framework that learns a dynamic neural radiance field (NeRF) for full-body talking humans from monocular videos. Prior work represents only the body pose or the face. However, humans communicate with their full body, combining body pose, hand gestures, as well as facial expressions. In this work, we propose TalkinNeRF, a unified NeRF-based network that represents the holistic 4D human motion. Given a monocular video of a subject, we learn corresponding modules for the body, face, and hands, that are combined together to generate the final result. To capture complex finger articulation, we learn an additional deformation field for the hands. Our multi-identity representation enables simultaneous training for multiple subjects, as well as robust animation under completely unseen poses. It can also generalize to novel identities, given only a short video as input. We demonstrate state-of-the-art performance for animating full-body talking humans, with fine-grained hand articulation and facial expressions.