TangoFlux: Super Fast and Faithful Text to Audio Generation with Flow Matching and Clap-Ranked Preference Optimization
Chia-Yu Hung, Navonil Majumder, Zhifeng Kong, Ambuj Mehrish, Rafael Valle, Bryan Catanzaro, Soujanya Poria
2024-12-31
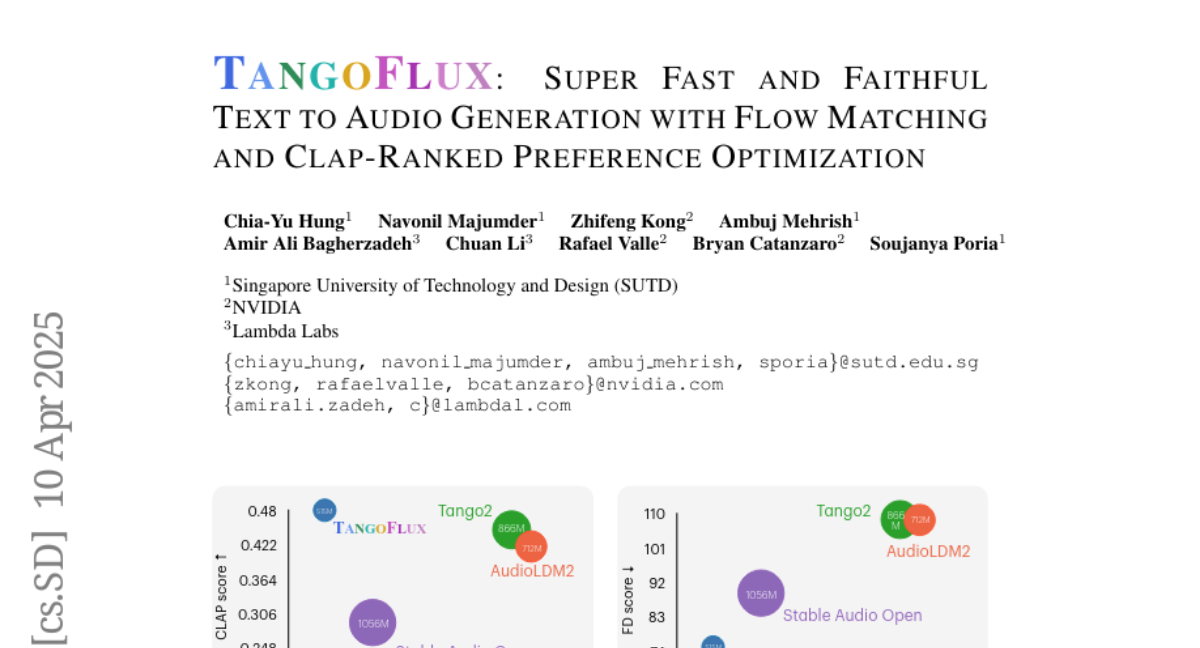
Summary
This paper talks about TangoFlux, a new and efficient model for generating audio from text prompts, capable of creating high-quality sound in just a few seconds.
What's the problem?
Generating audio from text can be challenging because existing models often struggle to create high-quality sound that matches the text description. Additionally, these models have difficulty understanding user preferences for audio, which can lead to less satisfying results. Traditional methods lack structured ways to measure how well the generated audio meets user expectations.
What's the solution?
To solve these problems, the authors developed TangoFlux, which uses a novel approach called CLAP-Ranked Preference Optimization (CRPO). This method helps the model learn from user preferences by generating and optimizing preference data. TangoFlux can create up to 30 seconds of audio at a high quality (44.1kHz) in just 3.7 seconds on a powerful GPU. It combines advanced techniques to ensure that the generated audio is both fast and faithful to the original text prompt.
Why it matters?
This research is important because it improves how AI can generate audio from text, making it useful for various applications like video games, movies, and virtual reality. By enhancing the quality and speed of audio generation, TangoFlux can help creators produce better sound effects and voiceovers quickly, leading to more engaging content.
Abstract
We introduce TangoFlux, an efficient Text-to-Audio (TTA) generative model with 515M parameters, capable of generating up to 30 seconds of 44.1kHz audio in just 3.7 seconds on a single A40 GPU. A key challenge in aligning TTA models lies in the difficulty of creating preference pairs, as TTA lacks structured mechanisms like verifiable rewards or gold-standard answers available for Large Language Models (LLMs). To address this, we propose CLAP-Ranked Preference Optimization (CRPO), a novel framework that iteratively generates and optimizes preference data to enhance TTA alignment. We demonstrate that the audio preference dataset generated using CRPO outperforms existing alternatives. With this framework, TangoFlux achieves state-of-the-art performance across both objective and subjective benchmarks. We open source all code and models to support further research in TTA generation.