TAPNext: Tracking Any Point (TAP) as Next Token Prediction
Artem Zholus, Carl Doersch, Yi Yang, Skanda Koppula, Viorica Patraucean, Xu Owen He, Ignacio Rocco, Mehdi S. M. Sajjadi, Sarath Chandar, Ross Goroshin
2025-04-11
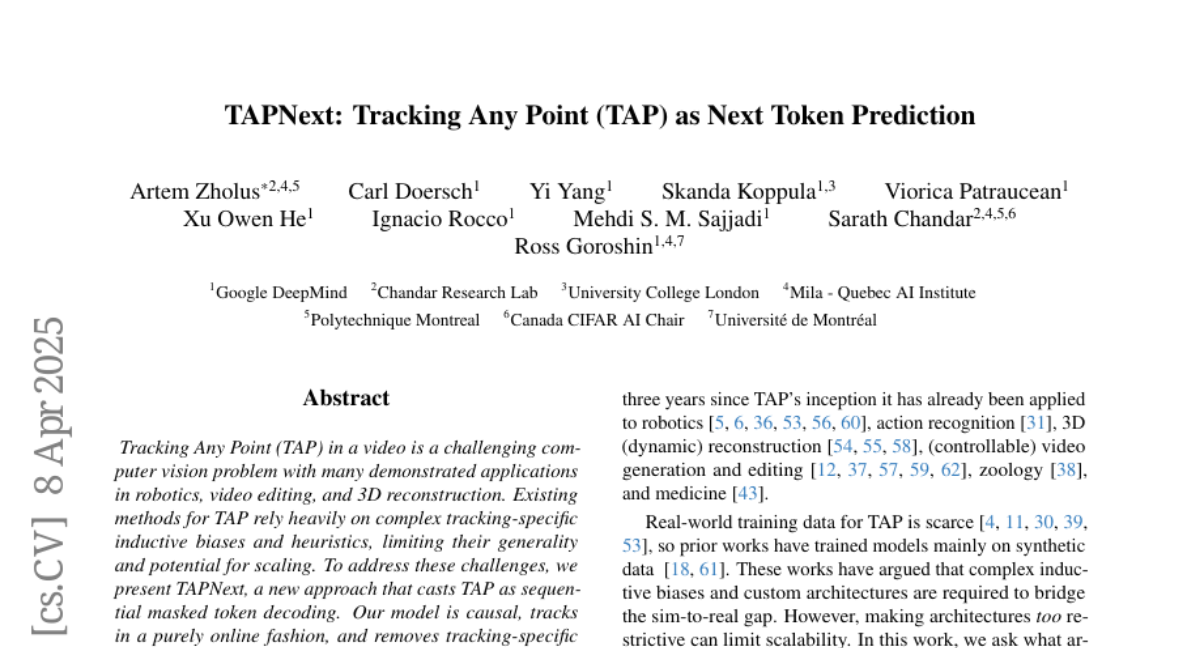
Summary
This paper talks about TAPNext, a new AI tool that tracks any point in a video super accurately, like following a speck of dust in a movie clip, by treating each point’s movement like predicting words in a sentence.
What's the problem?
Current video tracking tools need lots of special rules and can’t handle long videos well, making them slow and limited for real-time uses like robot navigation or video editing.
What's the solution?
TAPNext simplifies tracking by breaking it into small steps (like guessing the next word in a text), removing complex rules, and letting the AI learn patterns naturally, which makes it faster and better at handling live video.
Why it matters?
This helps robots follow objects smoothly, improves video editing tools for creators, and speeds up 3D modeling from videos, all while using less computing power.
Abstract
Tracking Any Point (TAP) in a video is a challenging computer vision problem with many demonstrated applications in robotics, video editing, and 3D reconstruction. Existing methods for TAP rely heavily on complex tracking-specific inductive biases and heuristics, limiting their generality and potential for scaling. To address these challenges, we present TAPNext, a new approach that casts TAP as sequential masked token decoding. Our model is causal, tracks in a purely online fashion, and removes tracking-specific inductive biases. This enables TAPNext to run with minimal latency, and removes the temporal windowing required by many existing state of art trackers. Despite its simplicity, TAPNext achieves a new state-of-the-art tracking performance among both online and offline trackers. Finally, we present evidence that many widely used tracking heuristics emerge naturally in TAPNext through end-to-end training.