TAROT: Task-Oriented Authorship Obfuscation Using Policy Optimization Methods
Gabriel Loiseau, Damien Sileo, Damien Riquet, Maxime Meyer, Marc Tommasi
2024-08-01
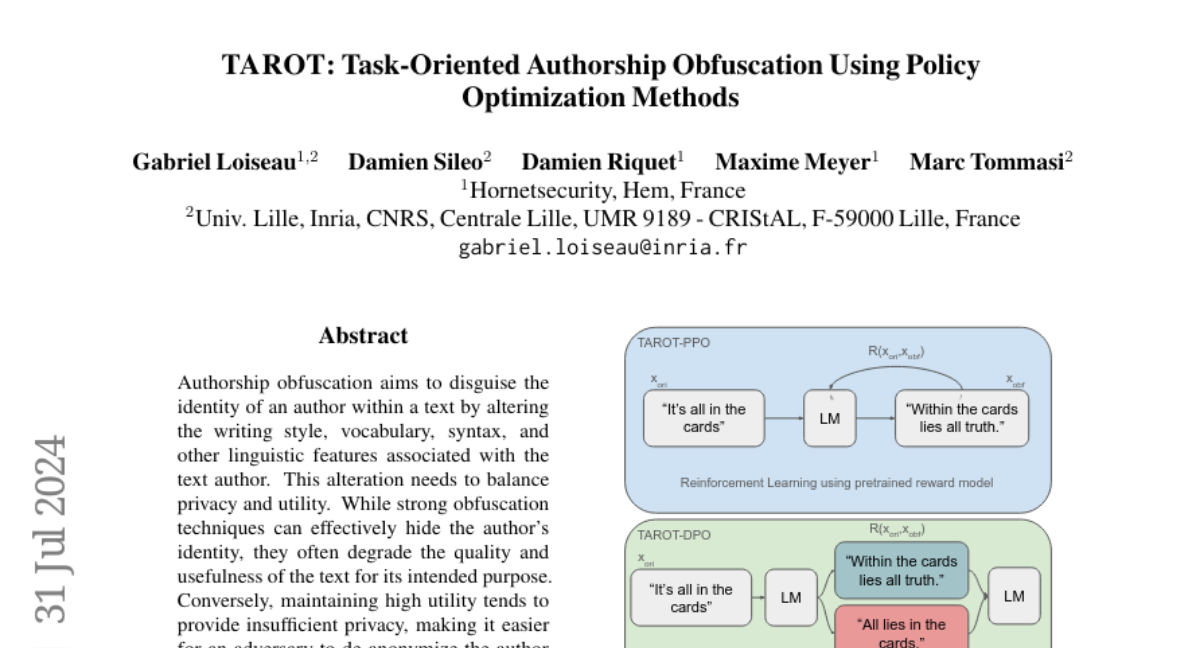
Summary
This paper discusses TAROT, a new method for authorship obfuscation that aims to hide the identity of an author in a text while still keeping the text useful and readable. It uses advanced techniques to balance privacy and the quality of the writing.
What's the problem?
When trying to disguise who wrote a piece of text, many existing methods either do a great job of hiding the author's identity but make the text hard to understand, or they keep the text clear and useful but fail to protect the author's privacy. This creates a challenge in finding a good middle ground where both privacy and utility are maintained.
What's the solution?
To solve this issue, the authors developed TAROT, which uses a technique called policy optimization to improve how texts are rewritten. This method allows TAROT to change the writing style and vocabulary while keeping the overall meaning intact. By focusing on both privacy and how well the text serves its purpose, TAROT can effectively reduce the chances of someone figuring out who wrote it without sacrificing quality.
Why it matters?
This research is important because it helps protect authors' identities in various contexts, such as academic writing or online communication, where privacy is crucial. By improving authorship obfuscation techniques, TAROT can contribute to safer and more secure ways for people to share their ideas without fear of being identified or targeted.
Abstract
Authorship obfuscation aims to disguise the identity of an author within a text by altering the writing style, vocabulary, syntax, and other linguistic features associated with the text author. This alteration needs to balance privacy and utility. While strong obfuscation techniques can effectively hide the author's identity, they often degrade the quality and usefulness of the text for its intended purpose. Conversely, maintaining high utility tends to provide insufficient privacy, making it easier for an adversary to de-anonymize the author. Thus, achieving an optimal trade-off between these two conflicting objectives is crucial. In this paper, we propose TAROT: Task-Oriented Authorship Obfuscation Using Policy Optimization, a new unsupervised authorship obfuscation method whose goal is to optimize the privacy-utility trade-off by regenerating the entire text considering its downstream utility. Our approach leverages policy optimization as a fine-tuning paradigm over small language models in order to rewrite texts by preserving author identity and downstream task utility. We show that our approach largely reduce the accuracy of attackers while preserving utility. We make our code and models publicly available.