Task-oriented Sequential Grounding in 3D Scenes
Zhuofan Zhang, Ziyu Zhu, Pengxiang Li, Tengyu Liu, Xiaojian Ma, Yixin Chen, Baoxiong Jia, Siyuan Huang, Qing Li
2024-08-09
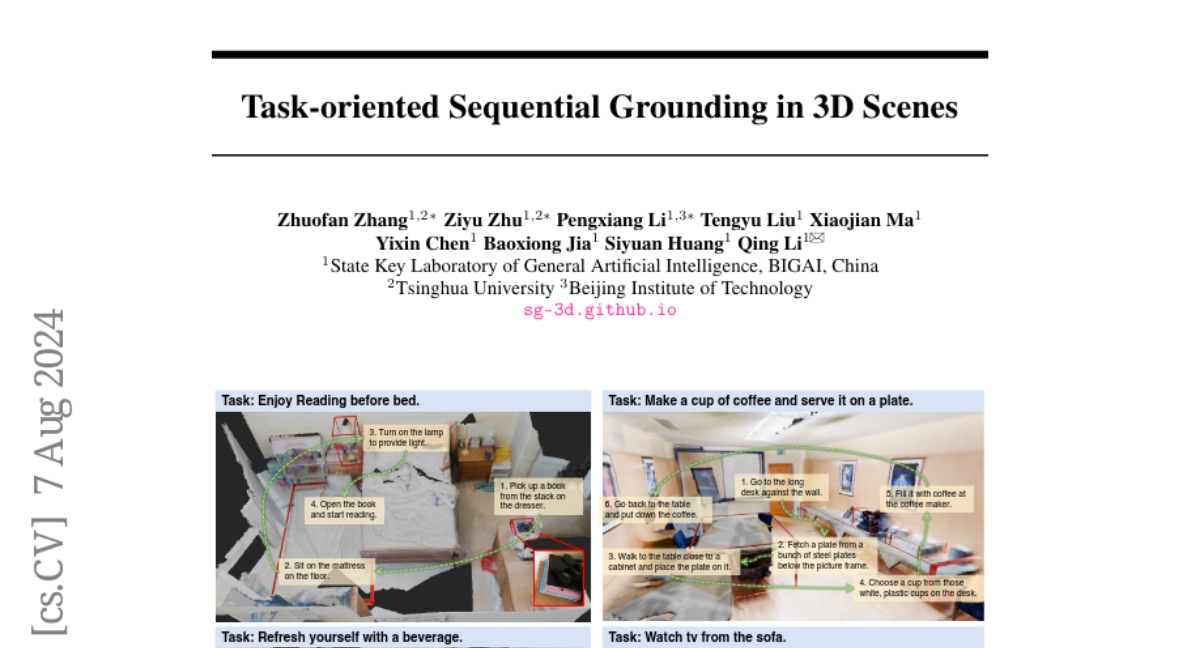
Summary
This paper presents a new approach called Task-oriented Sequential Grounding, which helps artificial intelligence (AI) understand and interact with 3D environments by following step-by-step instructions.
What's the problem?
Current AI systems often struggle to understand complex tasks in dynamic 3D spaces because most existing datasets focus on identifying objects in static scenes. This makes it hard for AI to perform real-world tasks that require a sequence of actions, like finding and using multiple objects in a room.
What's the solution?
To tackle this issue, the authors created a large dataset named SG3D, which includes over 22,000 tasks that involve locating objects in various indoor 3D scenes. They used real-world 3D scans and an automated system to generate tasks that require the AI to follow detailed instructions. Each task is verified by humans to ensure it makes sense and is achievable. The researchers then tested three advanced AI models on this dataset to see how well they could complete the tasks.
Why it matters?
This research is significant because it helps improve how AI interacts with the physical world, making it more capable of performing complex tasks in real-life situations. By providing a better understanding of how to ground language in 3D environments, this work could lead to advancements in robotics, virtual assistants, and other applications where AI needs to navigate and manipulate objects.
Abstract
Grounding natural language in physical 3D environments is essential for the advancement of embodied artificial intelligence. Current datasets and models for 3D visual grounding predominantly focus on identifying and localizing objects from static, object-centric descriptions. These approaches do not adequately address the dynamic and sequential nature of task-oriented grounding necessary for practical applications. In this work, we propose a new task: Task-oriented Sequential Grounding in 3D scenes, wherein an agent must follow detailed step-by-step instructions to complete daily activities by locating a sequence of target objects in indoor scenes. To facilitate this task, we introduce SG3D, a large-scale dataset containing 22,346 tasks with 112,236 steps across 4,895 real-world 3D scenes. The dataset is constructed using a combination of RGB-D scans from various 3D scene datasets and an automated task generation pipeline, followed by human verification for quality assurance. We adapted three state-of-the-art 3D visual grounding models to the sequential grounding task and evaluated their performance on SG3D. Our results reveal that while these models perform well on traditional benchmarks, they face significant challenges with task-oriented sequential grounding, underscoring the need for further research in this area.