TCAN: Animating Human Images with Temporally Consistent Pose Guidance using Diffusion Models
Jeongho Kim, Min-Jung Kim, Junsoo Lee, Jaegul Choo
2024-07-15
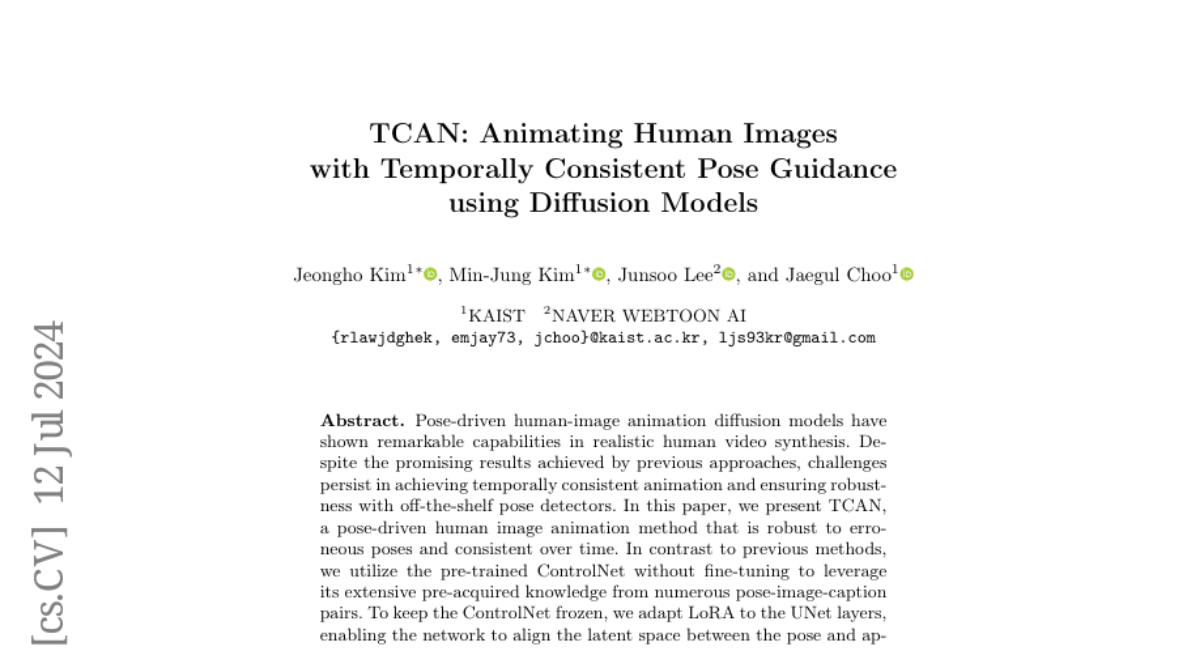
Summary
This paper introduces TCAN, a new method for animating human images using advanced AI techniques to create smooth and consistent animations based on poses.
What's the problem?
Creating realistic animations from still images can be challenging, especially when trying to maintain smooth movements over time. Previous methods often struggled with inconsistencies in the animation and could be sensitive to errors in pose detection, leading to unnatural results.
What's the solution?
TCAN addresses these issues by using a technique called diffusion models to generate poses that guide the animation of human images. It leverages a pre-trained model called ControlNet without changing its settings, which helps it understand poses better. TCAN also adds a special layer to handle time-based information, making the animations more stable and less affected by errors in pose detection. This allows for better quality animations that are consistent over time, even when the initial pose estimates are not perfect.
Why it matters?
This research is significant because it improves how we animate human figures in videos, making it easier to create high-quality animations from just a single image. This can be useful in various fields such as video game design, film production, and virtual reality, where realistic character movements are essential for engaging storytelling and immersive experiences.
Abstract
Pose-driven human-image animation diffusion models have shown remarkable capabilities in realistic human video synthesis. Despite the promising results achieved by previous approaches, challenges persist in achieving temporally consistent animation and ensuring robustness with off-the-shelf pose detectors. In this paper, we present TCAN, a pose-driven human image animation method that is robust to erroneous poses and consistent over time. In contrast to previous methods, we utilize the pre-trained ControlNet without fine-tuning to leverage its extensive pre-acquired knowledge from numerous pose-image-caption pairs. To keep the ControlNet frozen, we adapt LoRA to the UNet layers, enabling the network to align the latent space between the pose and appearance features. Additionally, by introducing an additional temporal layer to the ControlNet, we enhance robustness against outliers of the pose detector. Through the analysis of attention maps over the temporal axis, we also designed a novel temperature map leveraging pose information, allowing for a more static background. Extensive experiments demonstrate that the proposed method can achieve promising results in video synthesis tasks encompassing various poses, like chibi. Project Page: https://eccv2024tcan.github.io/