Teaching Embodied Reinforcement Learning Agents: Informativeness and Diversity of Language Use
Jiajun Xi, Yinong He, Jianing Yang, Yinpei Dai, Joyce Chai
2024-11-01
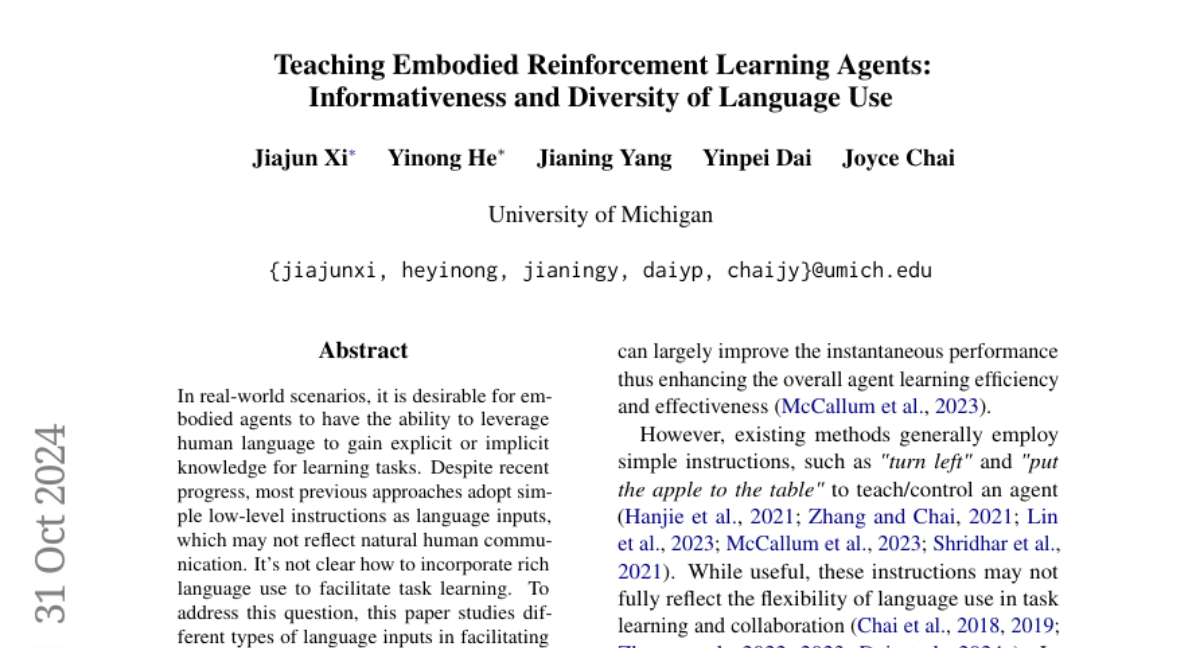
Summary
This paper explores how using different types of language can help robots and AI agents learn tasks better. It focuses on how informative and diverse language inputs can improve the learning process for these agents.
What's the problem?
Many AI agents currently rely on simple, low-level instructions that don't resemble natural human communication. This makes it difficult for them to learn effectively from human language, as they miss out on the richness and complexity of how people express ideas.
What's the solution?
The authors investigate various types of language inputs, specifically looking at how informative language (which gives feedback about past actions and guidance for future actions) and diverse language (which uses different ways to express the same idea) affect the learning of AI agents. They find that agents trained with richer and more varied language feedback perform better and adapt faster to new tasks compared to those trained with simpler instructions.
Why it matters?
This research is significant because it shows that improving the way we communicate with AI agents can lead to better learning outcomes. By using more informative and diverse language, we can help these agents understand tasks more effectively, which is crucial for their use in real-world applications like robotics, virtual assistants, and more.
Abstract
In real-world scenarios, it is desirable for embodied agents to have the ability to leverage human language to gain explicit or implicit knowledge for learning tasks. Despite recent progress, most previous approaches adopt simple low-level instructions as language inputs, which may not reflect natural human communication. It's not clear how to incorporate rich language use to facilitate task learning. To address this question, this paper studies different types of language inputs in facilitating reinforcement learning (RL) embodied agents. More specifically, we examine how different levels of language informativeness (i.e., feedback on past behaviors and future guidance) and diversity (i.e., variation of language expressions) impact agent learning and inference. Our empirical results based on four RL benchmarks demonstrate that agents trained with diverse and informative language feedback can achieve enhanced generalization and fast adaptation to new tasks. These findings highlight the pivotal role of language use in teaching embodied agents new tasks in an open world. Project website: https://github.com/sled-group/Teachable_RL