Text-Driven Neural Collaborative Filtering Model for Paper Source Tracing
Aobo Xu, Bingyu Chang, Qingpeng Liu, Ling Jian
2024-07-26
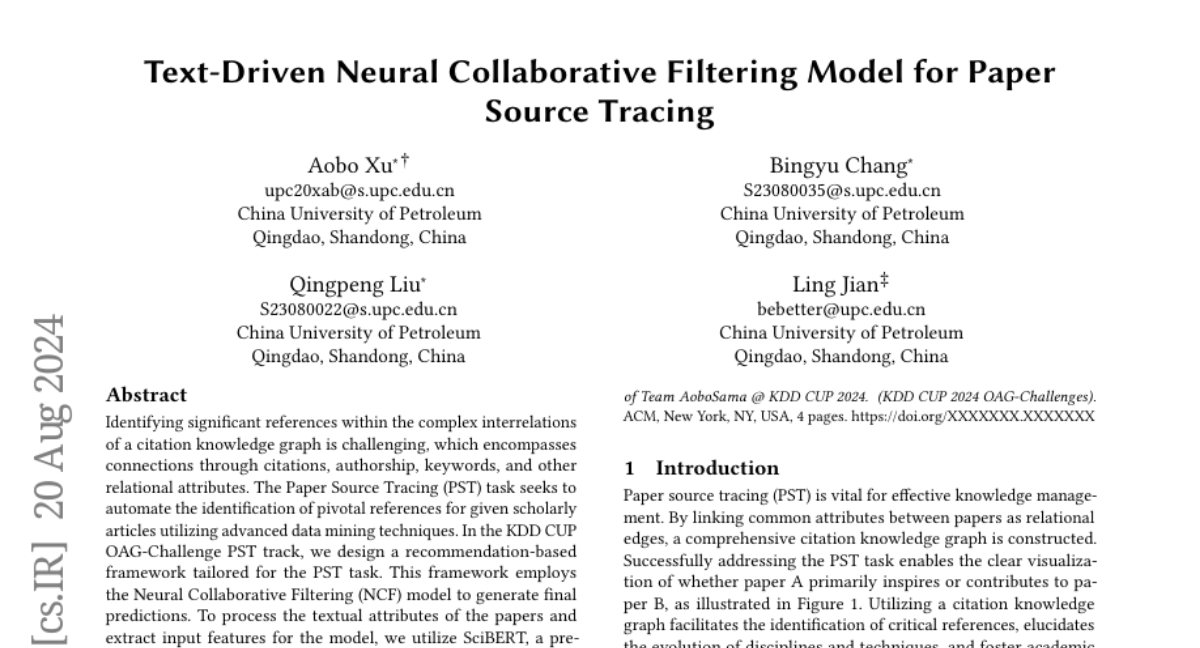
Summary
This paper discusses a new method called Text-Driven Neural Collaborative Filtering Model for Paper Source Tracing, which helps automatically identify important references in academic papers using advanced data analysis techniques.
What's the problem?
Finding significant references within the complex web of citations and relationships in academic articles is difficult. Researchers often struggle to pinpoint which sources are most important for their work, especially when dealing with many papers that cite each other in various ways. This makes it hard to trace back the origins of ideas and research effectively.
What's the solution?
To tackle this issue, the authors developed a recommendation-based framework specifically for the Paper Source Tracing (PST) task. They used a model called Neural Collaborative Filtering (NCF) to analyze the text of scholarly articles and predict which references are pivotal. They also employed SciBERT, a pre-trained language model, to process the text and extract useful features. Their method achieved a high score on the Mean Average Precision (MAP) metric, outperforming other models and ranking well in a competition.
Why it matters?
This research is important because it automates the process of identifying key references in academic papers, making it easier for researchers to find relevant sources quickly. By improving how we trace the origins of research ideas, this method can enhance academic work and collaboration, ultimately contributing to more effective scientific communication.
Abstract
Identifying significant references within the complex interrelations of a citation knowledge graph is challenging, which encompasses connections through citations, authorship, keywords, and other relational attributes. The Paper Source Tracing (PST) task seeks to automate the identification of pivotal references for given scholarly articles utilizing advanced data mining techniques. In the KDD CUP 2024, we design a recommendation-based framework tailored for the PST task. This framework employs the Neural Collaborative Filtering (NCF) model to generate final predictions. To process the textual attributes of the papers and extract input features for the model, we utilize SciBERT, a pre-trained language model. According to the experimental results, our method achieved a score of 0.37814 on the Mean Average Precision (MAP) metric, outperforming baseline models and ranking 11th among all participating teams. The source code is publicly available at https://github.com/MyLove-XAB/KDDCupFinal.