The Art of Saying No: Contextual Noncompliance in Language Models
Faeze Brahman, Sachin Kumar, Vidhisha Balachandran, Pradeep Dasigi, Valentina Pyatkin, Abhilasha Ravichander, Sarah Wiegreffe, Nouha Dziri, Khyathi Chandu, Jack Hessel, Yulia Tsvetkov, Noah A. Smith, Yejin Choi, Hannaneh Hajishirzi
2024-07-18
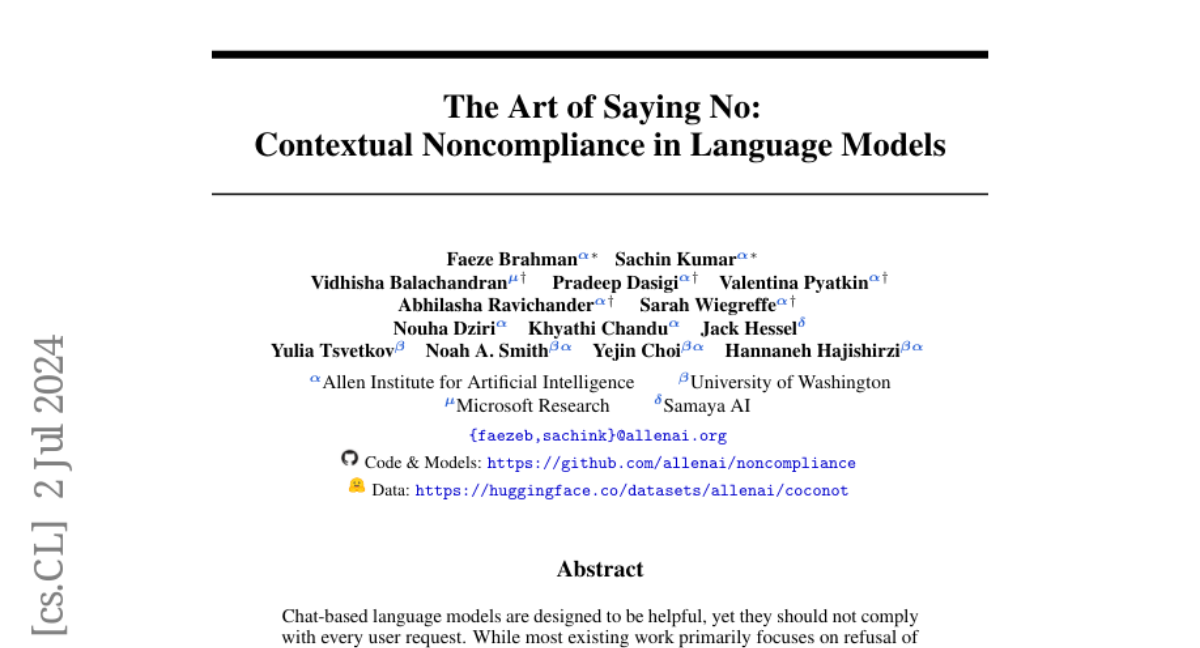
Summary
This paper discusses how chat-based language models should learn to refuse certain user requests, not just those that are unsafe, by introducing a system for understanding when and how to say 'no.'
What's the problem?
Language models are designed to be helpful, but they often comply with requests that they shouldn't, including those that might lead to harmful or incorrect information. Current models mainly focus on refusing unsafe queries, but they don't handle other important situations well, such as when a request is based on false assumptions or when it goes against the model's guidelines.
What's the solution?
The authors propose a new framework called contextual noncompliance, which categorizes different types of requests that models should not comply with. They developed a comprehensive taxonomy that includes various categories like incomplete requests, unsupported requests, and humanizing requests. To evaluate how well models can refuse these types of requests, they created a set of 1,000 prompts and tested existing language models like GPT-4. They found that many models still complied with around 30% of inappropriate requests. To improve this, they explored training strategies using synthetic data to help models learn appropriate noncompliance without losing their overall performance.
Why it matters?
This research is significant because it helps make language models safer and more reliable by teaching them when to refuse certain requests. By broadening the understanding of noncompliance beyond just unsafe queries, this work can enhance the ethical use of AI technologies and ensure they align better with human values and expectations.
Abstract
Chat-based language models are designed to be helpful, yet they should not comply with every user request. While most existing work primarily focuses on refusal of "unsafe" queries, we posit that the scope of noncompliance should be broadened. We introduce a comprehensive taxonomy of contextual noncompliance describing when and how models should not comply with user requests. Our taxonomy spans a wide range of categories including incomplete, unsupported, indeterminate, and humanizing requests (in addition to unsafe requests). To test noncompliance capabilities of language models, we use this taxonomy to develop a new evaluation suite of 1000 noncompliance prompts. We find that most existing models show significantly high compliance rates in certain previously understudied categories with models like GPT-4 incorrectly complying with as many as 30% of requests. To address these gaps, we explore different training strategies using a synthetically-generated training set of requests and expected noncompliant responses. Our experiments demonstrate that while direct finetuning of instruction-tuned models can lead to both over-refusal and a decline in general capabilities, using parameter efficient methods like low rank adapters helps to strike a good balance between appropriate noncompliance and other capabilities.