The Good, The Bad, and The Greedy: Evaluation of LLMs Should Not Ignore Non-Determinism
Yifan Song, Guoyin Wang, Sujian Li, Bill Yuchen Lin
2024-07-16
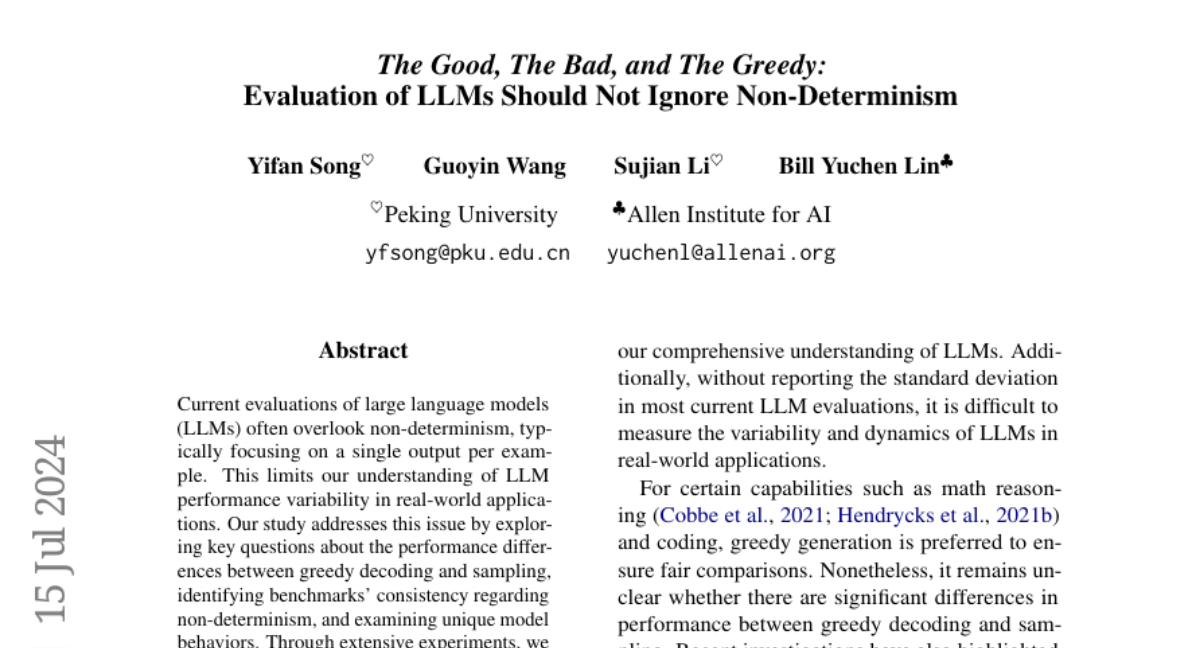
Summary
This paper discusses the importance of considering non-determinism in evaluating large language models (LLMs) and compares different methods for generating outputs from these models.
What's the problem?
Current evaluations of LLMs often focus on a single output for each input, which doesn't capture the variability in how these models can respond. This oversight makes it hard to understand how well LLMs perform in real-world situations, where they might give different answers to the same question depending on the method used to generate those answers.
What's the solution?
The authors explore two main methods for generating outputs: greedy decoding, which always picks the most likely next word, and sampling, which introduces randomness. They conduct experiments to see how these methods affect performance across various tasks. Their findings show that greedy decoding generally performs better than sampling for most tasks, but sampling can be beneficial for creative tasks. They also introduce a 'best-of-N' sampling method that allows smaller LLMs to perform as well as larger models like GPT-4-Turbo, highlighting the potential of smaller models.
Why it matters?
This research is important because it encourages a more comprehensive evaluation of LLMs by considering their variability in responses. By understanding how different generation methods impact performance, developers can create better and more reliable AI systems that perform well across a wider range of tasks and applications.
Abstract
Current evaluations of large language models (LLMs) often overlook non-determinism, typically focusing on a single output per example. This limits our understanding of LLM performance variability in real-world applications. Our study addresses this issue by exploring key questions about the performance differences between greedy decoding and sampling, identifying benchmarks' consistency regarding non-determinism, and examining unique model behaviors. Through extensive experiments, we observe that greedy decoding generally outperforms sampling methods for most evaluated tasks. We also observe consistent performance across different LLM sizes and alignment methods, noting that alignment can reduce sampling variance. Moreover, our best-of-N sampling approach demonstrates that smaller LLMs can match or surpass larger models such as GPT-4-Turbo, highlighting the untapped potential of smaller LLMs. This research shows the importance of considering non-determinism in LLM evaluations and provides insights for future LLM development and evaluation.