The Imperative of Conversation Analysis in the Era of LLMs: A Survey of Tasks, Techniques, and Trends
Xinghua Zhang, Haiyang Yu, Yongbin Li, Minzheng Wang, Longze Chen, Fei Huang
2024-09-27
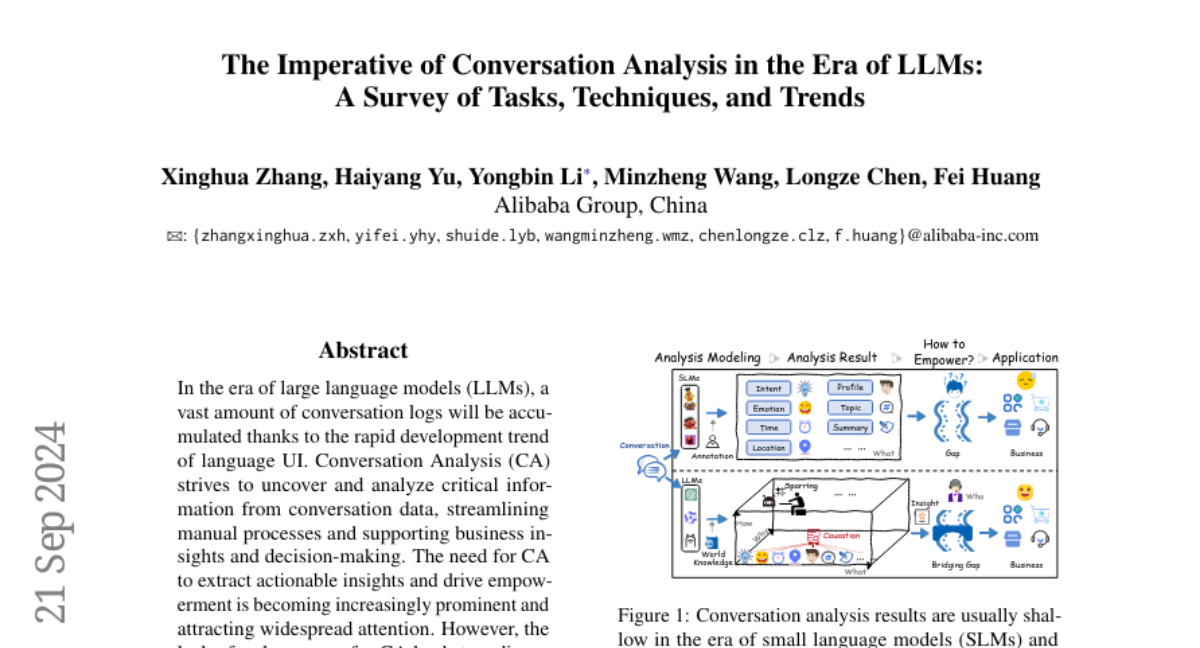
Summary
This paper talks about the importance of conversation analysis (CA) in the context of large language models (LLMs). It reviews how CA can help extract valuable insights from conversation data to improve business decisions and interactions.
What's the problem?
As LLMs become more advanced, they generate a lot of conversation logs, but there is a challenge in effectively analyzing this data. The current methods for conversation analysis are scattered and lack a clear framework, making it hard to use these insights systematically in business applications. This fragmentation means that many potential benefits of analyzing conversations are not being fully realized.
What's the solution?
The authors propose a structured approach to conversation analysis by defining clear tasks and steps involved in the process. They outline four key steps: reconstructing the conversation scene, analyzing details, conducting targeted training, and generating conversations based on this training. By organizing the field of CA, they aim to create a more systematic way to analyze conversations and derive actionable insights, which can help bridge the gap between research and practical business applications.
Why it matters?
This research is important because it highlights how effective conversation analysis can enhance the use of LLMs in real-world scenarios. By improving our understanding of conversations, businesses can make better decisions, improve customer interactions, and leverage data more effectively. As LLMs continue to evolve, having robust conversation analysis techniques will be crucial for maximizing their potential in various industries.
Abstract
In the era of large language models (LLMs), a vast amount of conversation logs will be accumulated thanks to the rapid development trend of language UI. Conversation Analysis (CA) strives to uncover and analyze critical information from conversation data, streamlining manual processes and supporting business insights and decision-making. The need for CA to extract actionable insights and drive empowerment is becoming increasingly prominent and attracting widespread attention. However, the lack of a clear scope for CA leads to a dispersion of various techniques, making it difficult to form a systematic technical synergy to empower business applications. In this paper, we perform a thorough review and systematize CA task to summarize the existing related work. Specifically, we formally define CA task to confront the fragmented and chaotic landscape in this field, and derive four key steps of CA from conversation scene reconstruction, to in-depth attribution analysis, and then to performing targeted training, finally generating conversations based on the targeted training for achieving the specific goals. In addition, we showcase the relevant benchmarks, discuss potential challenges and point out future directions in both industry and academia. In view of current advancements, it is evident that the majority of efforts are still concentrated on the analysis of shallow conversation elements, which presents a considerable gap between the research and business, and with the assist of LLMs, recent work has shown a trend towards research on causality and strategic tasks which are sophisticated and high-level. The analyzed experiences and insights will inevitably have broader application value in business operations that target conversation logs.