The MERIT Dataset: Modelling and Efficiently Rendering Interpretable Transcripts
I. de Rodrigo, A. Sanchez-Cuadrado, J. Boal, A. J. Lopez-Lopez
2024-09-04
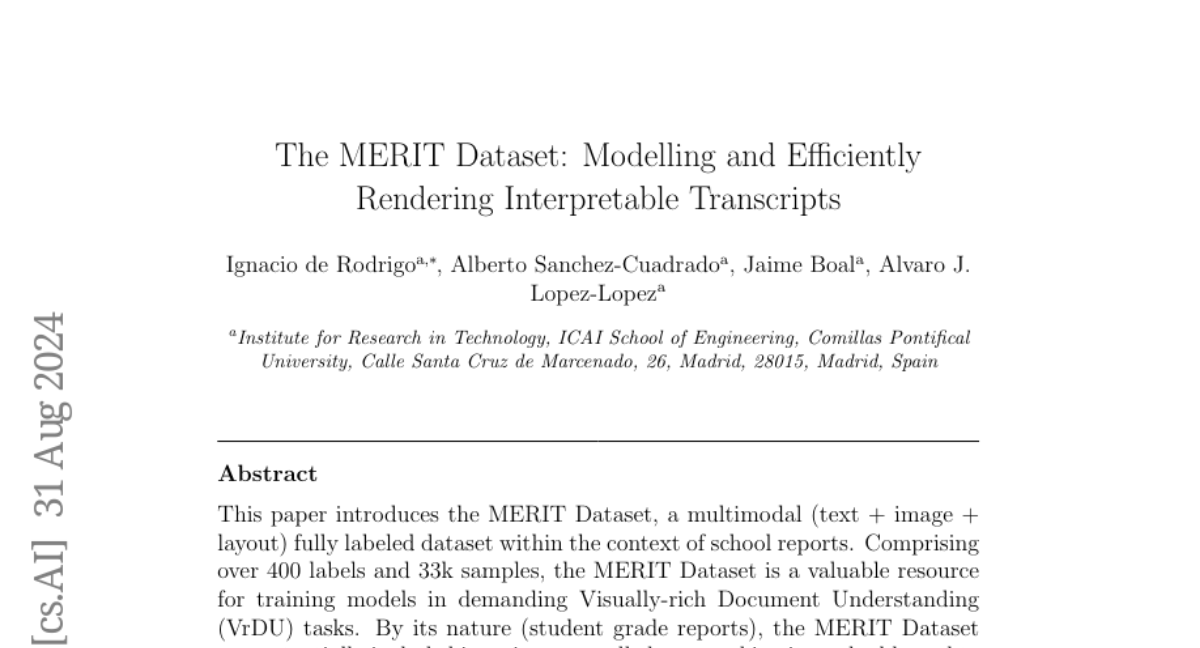
Summary
This paper talks about the MERIT Dataset, a new resource designed for understanding and analyzing school reports by combining text, images, and layouts.
What's the problem?
Existing datasets for analyzing documents like school reports are often limited and may not provide enough information to train effective models. Additionally, these datasets may contain biases that aren't well understood, making it difficult to evaluate how AI systems might perform in real-world scenarios.
What's the solution?
The MERIT Dataset includes over 33,000 samples and more than 400 labels that cover various aspects of school reports. It is fully labeled and multimodal, meaning it combines text, images, and layout information. The dataset was created to help researchers study biases in language models and improve their performance in visually-rich document understanding tasks. The authors also present a benchmark showing that even advanced models struggle with this dataset, indicating its complexity and usefulness for training.
Why it matters?
This research is important because it provides a comprehensive tool for training AI systems to better understand complex documents like school reports. By addressing potential biases and offering a rich dataset, the MERIT Dataset can lead to more reliable AI applications in education and beyond, ultimately improving how we analyze and interpret important information.
Abstract
This paper introduces the MERIT Dataset, a multimodal (text + image + layout) fully labeled dataset within the context of school reports. Comprising over 400 labels and 33k samples, the MERIT Dataset is a valuable resource for training models in demanding Visually-rich Document Understanding (VrDU) tasks. By its nature (student grade reports), the MERIT Dataset can potentially include biases in a controlled way, making it a valuable tool to benchmark biases induced in Language Models (LLMs). The paper outlines the dataset's generation pipeline and highlights its main features in the textual, visual, layout, and bias domains. To demonstrate the dataset's utility, we present a benchmark with token classification models, showing that the dataset poses a significant challenge even for SOTA models and that these would greatly benefit from including samples from the MERIT Dataset in their pretraining phase.