The Nature of Mathematical Modeling and Probabilistic Optimization Engineering in Generative AI
Fulu Li
2024-10-25
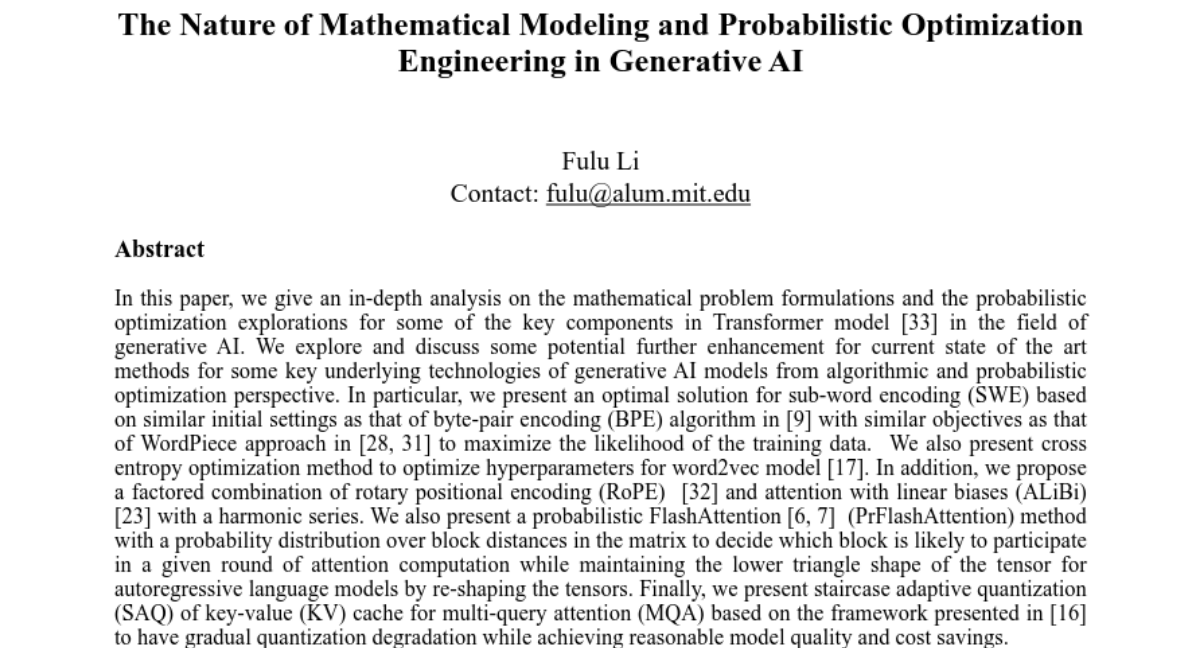
Summary
This paper discusses the mathematical modeling and optimization techniques used in generative AI, particularly focusing on improving Transformer models through various innovative methods.
What's the problem?
Generative AI models, especially those based on Transformers, require complex mathematical formulations and optimizations to function effectively. However, existing methods may not fully utilize the potential of these models, leading to inefficiencies in how they process and generate data. This can limit their performance in tasks like language understanding and generation.
What's the solution?
The authors propose several enhancements to current generative AI techniques. They introduce a new approach for sub-word encoding that maximizes the likelihood of training data, optimize hyperparameters for models like word2vec, and combine different encoding methods to improve efficiency. Additionally, they present a probabilistic method called FlashAttention that helps decide which parts of the data are most relevant during processing. These innovations aim to streamline the training process and improve model performance without requiring excessive computational resources.
Why it matters?
This research is important because it advances our understanding of how to effectively train and optimize generative AI models. By improving the mathematical foundations and optimization strategies used in these models, we can enhance their capabilities in generating high-quality text, images, and other forms of data, making them more useful for applications in various fields like natural language processing, computer vision, and beyond.
Abstract
In this paper, we give an in-depth analysis on the mathematical problem formulations and the probabilistic optimization explorations for some of the key components in Transformer model [33] in the field of generative AI. We explore and discuss some potential further enhancement for current state of the art methods for some key underlying technologies of generative AI models from algorithmic and probabilistic optimization perspective. In particular, we present an optimal solution for sub-word encoding (SWE) based on similar initial settings as that of byte-pair encoding (BPE) algorithm in [9] with similar objectives as that of WordPiece approach in [28, 31] to maximize the likelihood of the training data. We also present cross entropy optimization method to optimize hyperparameters for word2vec model [17]. In addition, we propose a factored combination of rotary positional encoding (RoPE) [32] and attention with linear biases (ALiBi) [23] with a harmonic series. We also present a probabilistic FlashAttention [6, 7] (PrFlashAttention) method with a probability distribution over block distances in the matrix to decide which block is likely to participate in a given round of attention computation while maintaining the lower triangle shape of the tensor for autoregressive language models by re-shaping the tensors. Finally, we present staircase adaptive quantization (SAQ) of key-value (KV) cache for multi-query attention (MQA) based on the framework presented in [16] to have gradual quantization degradation while achieving reasonable model quality and cost savings.