The Russian-focused embedders' exploration: ruMTEB benchmark and Russian embedding model design
Artem Snegirev, Maria Tikhonova, Anna Maksimova, Alena Fenogenova, Alexander Abramov
2024-08-23
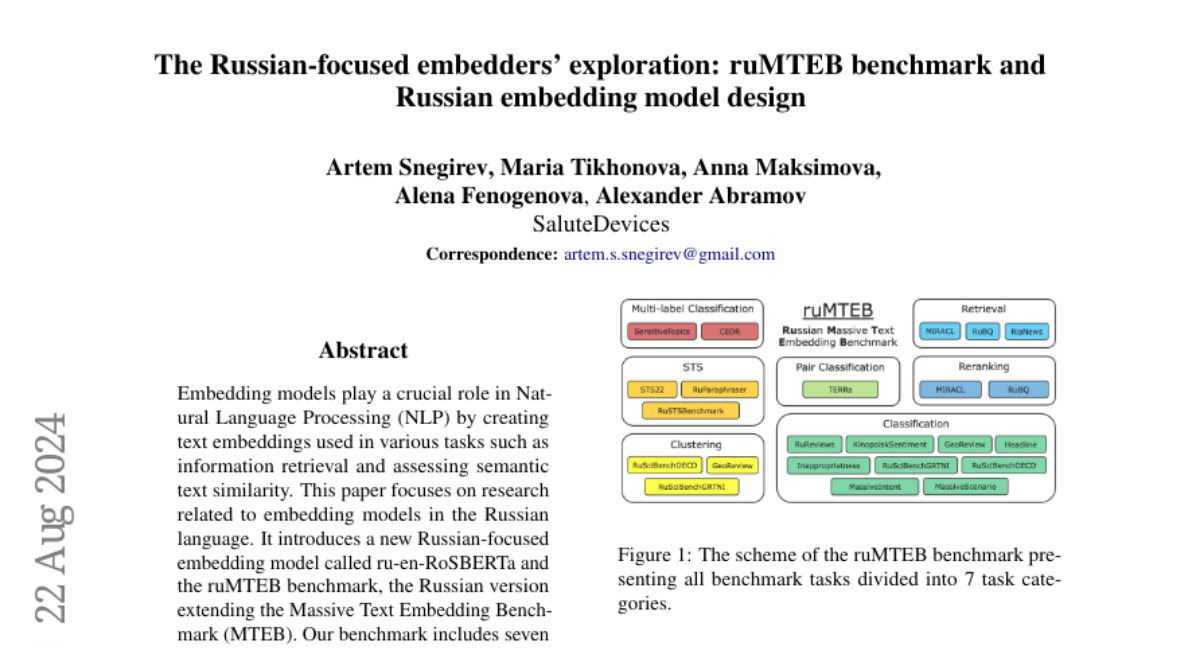
Summary
This paper discusses the development of a new embedding model for the Russian language called ru-en-RoSBERTa and introduces the ruMTEB benchmark, which helps evaluate how well different models perform on various language tasks.
What's the problem?
Embedding models are essential in Natural Language Processing (NLP) for understanding and processing text. However, there has been limited research focused on Russian language models, making it difficult to assess their effectiveness in tasks such as text similarity and classification.
What's the solution?
The authors created the ruMTEB benchmark, which includes seven different tasks to evaluate Russian language models. They also designed the ru-en-RoSBERTa embedding model, which is tested against other Russian and multilingual models on this benchmark. The results show that their new model performs as well as or better than existing top models.
Why it matters?
This research is important because it enhances the tools available for processing the Russian language, which can improve applications in areas like information retrieval and machine translation. By providing a robust evaluation framework and a high-performing model, it supports further advancements in NLP for Russian.
Abstract
Embedding models play a crucial role in Natural Language Processing (NLP) by creating text embeddings used in various tasks such as information retrieval and assessing semantic text similarity. This paper focuses on research related to embedding models in the Russian language. It introduces a new Russian-focused embedding model called ru-en-RoSBERTa and the ruMTEB benchmark, the Russian version extending the Massive Text Embedding Benchmark (MTEB). Our benchmark includes seven categories of tasks, such as semantic textual similarity, text classification, reranking, and retrieval. The research also assesses a representative set of Russian and multilingual models on the proposed benchmark. The findings indicate that the new model achieves results that are on par with state-of-the-art models in Russian. We release the model ru-en-RoSBERTa, and the ruMTEB framework comes with open-source code, integration into the original framework and a public leaderboard.