The VoxCeleb Speaker Recognition Challenge: A Retrospective
Jaesung Huh, Joon Son Chung, Arsha Nagrani, Andrew Brown, Jee-weon Jung, Daniel Garcia-Romero, Andrew Zisserman
2024-09-02
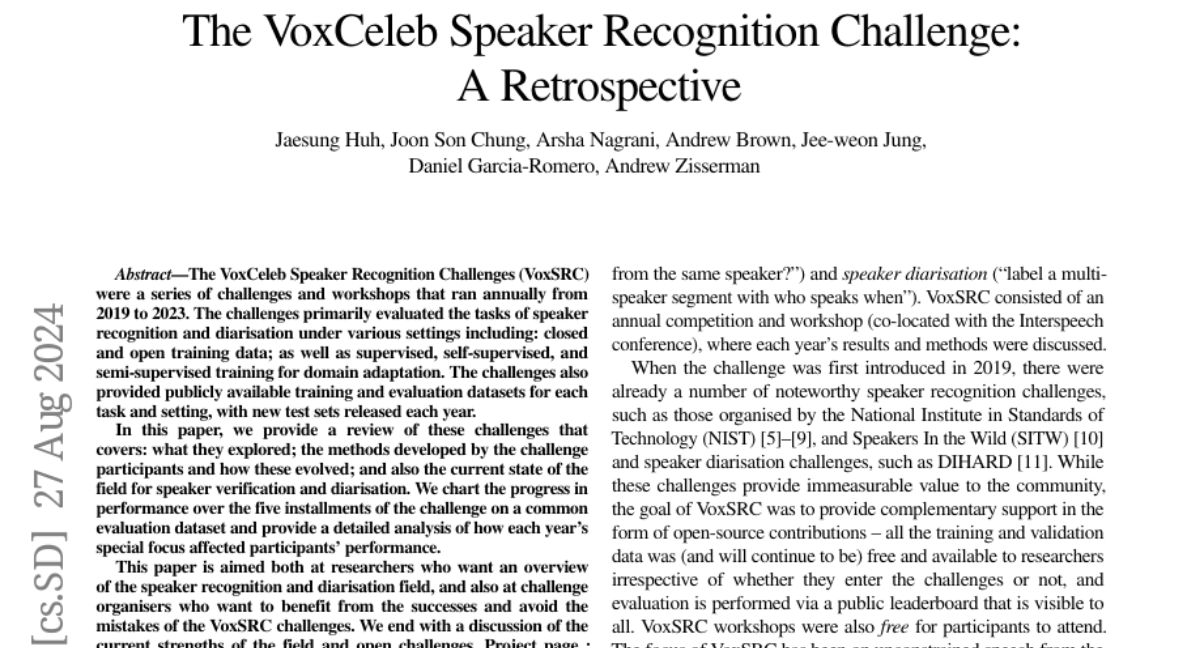
Summary
This paper reviews the VoxCeleb Speaker Recognition Challenges (VoxSRC), which were held annually from 2019 to 2023 to improve speaker recognition technology.
What's the problem?
There is a need for better speaker recognition systems that can accurately identify and track speakers in various situations. Previous challenges focused on limited tasks and didn't fully test how well these systems perform in real-world scenarios, especially with different types of training data.
What's the solution?
The authors provide a retrospective analysis of the VoxSRC challenges, highlighting the methods developed by participants and how these methods evolved over time. They discuss the progress made in speaker recognition and diarization (the process of identifying who is speaking when) across the five challenges, including how different focuses each year affected performance. They also present new evaluation metrics to assess the effectiveness of the models used in these challenges.
Why it matters?
This research is significant because it helps researchers understand the advancements made in speaker recognition technology over the years. By analyzing past challenges, it offers insights that can guide future developments in this field, ultimately leading to more accurate and reliable systems for identifying speakers in various contexts.
Abstract
The VoxCeleb Speaker Recognition Challenges (VoxSRC) were a series of challenges and workshops that ran annually from 2019 to 2023. The challenges primarily evaluated the tasks of speaker recognition and diarisation under various settings including: closed and open training data; as well as supervised, self-supervised, and semi-supervised training for domain adaptation. The challenges also provided publicly available training and evaluation datasets for each task and setting, with new test sets released each year. In this paper, we provide a review of these challenges that covers: what they explored; the methods developed by the challenge participants and how these evolved; and also the current state of the field for speaker verification and diarisation. We chart the progress in performance over the five installments of the challenge on a common evaluation dataset and provide a detailed analysis of how each year's special focus affected participants' performance. This paper is aimed both at researchers who want an overview of the speaker recognition and diarisation field, and also at challenge organisers who want to benefit from the successes and avoid the mistakes of the VoxSRC challenges. We end with a discussion of the current strengths of the field and open challenges. Project page : https://mm.kaist.ac.kr/datasets/voxceleb/voxsrc/workshop.html