THEANINE: Revisiting Memory Management in Long-term Conversations with Timeline-augmented Response Generation
Seo Hyun Kim, Kai Tzu-iunn Ong, Taeyoon Kwon, Namyoung Kim, Keummin Ka, SeongHyeon Bae, Yohan Jo, Seung-won Hwang, Dongha Lee, Jinyoung Yeo
2024-06-18
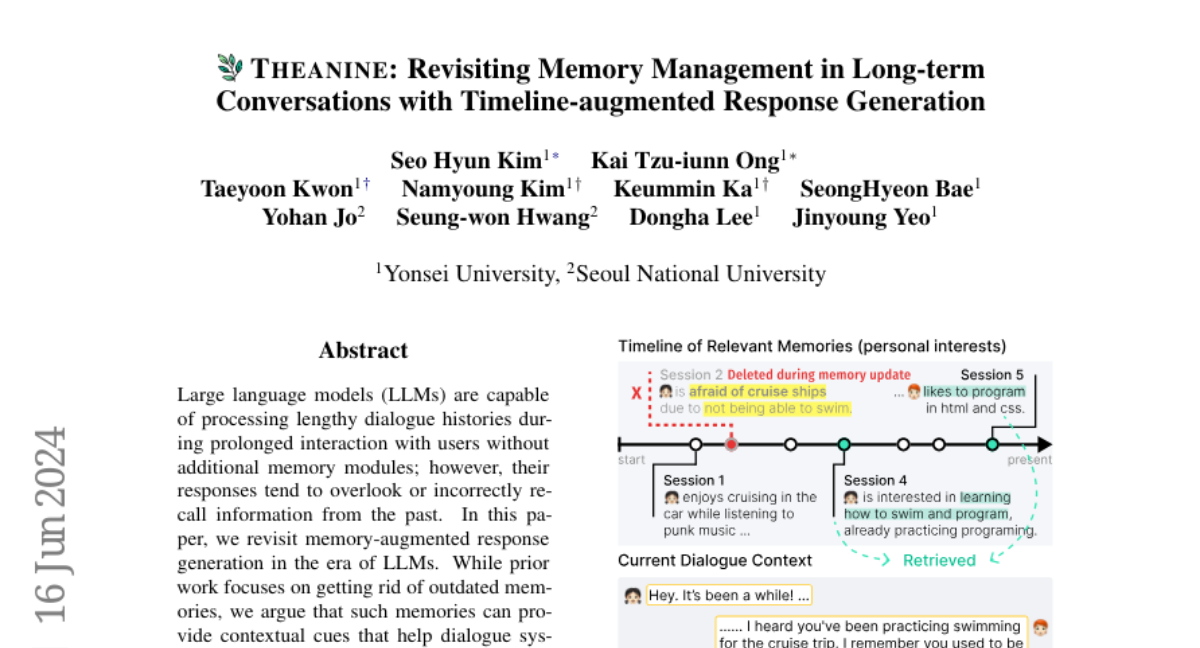
Summary
This paper introduces Theanine, a new framework designed to improve how large language models (LLMs) handle long-term conversations by using memory timelines. It aims to enhance the quality of responses by better utilizing past information in dialogues.
What's the problem?
While LLMs can process long conversations, they often struggle to remember or accurately recall important details from earlier in the dialogue. This can lead to responses that are irrelevant or incorrect, which diminishes the effectiveness of AI in maintaining coherent and meaningful conversations over time. Previous approaches have focused on removing outdated memories, but this can overlook valuable context that could help the model understand the conversation better.
What's the solution?
To address this issue, the authors developed Theanine, which incorporates memory timelines into the response generation process. These timelines consist of a series of memories that show how events have developed and relate to each other over time. By using these timelines, LLMs can generate responses that are more informed and contextually relevant. Additionally, they introduced TeaFarm, a new question-answering system that helps evaluate the effectiveness of these memory-augmented responses in long-term conversations.
Why it matters?
This research is important because it enhances the ability of AI systems to engage in meaningful and coherent conversations with users. By improving how LLMs utilize past information, Theanine can lead to more accurate and relevant interactions, making AI assistants more effective in real-world applications like customer service, therapy, and personal assistance. This advancement could significantly improve user satisfaction and trust in conversational AI technologies.
Abstract
Large language models (LLMs) are capable of processing lengthy dialogue histories during prolonged interaction with users without additional memory modules; however, their responses tend to overlook or incorrectly recall information from the past. In this paper, we revisit memory-augmented response generation in the era of LLMs. While prior work focuses on getting rid of outdated memories, we argue that such memories can provide contextual cues that help dialogue systems understand the development of past events and, therefore, benefit response generation. We present Theanine, a framework that augments LLMs' response generation with memory timelines -- series of memories that demonstrate the development and causality of relevant past events. Along with Theanine, we introduce TeaFarm, a counterfactual-driven question-answering pipeline addressing the limitation of G-Eval in long-term conversations. Supplementary videos of our methods and the TeaBag dataset for TeaFarm evaluation are in https://theanine-693b0.web.app/.