ThinkGrasp: A Vision-Language System for Strategic Part Grasping in Clutter
Yaoyao Qian, Xupeng Zhu, Ondrej Biza, Shuo Jiang, Linfeng Zhao, Haojie Huang, Yu Qi, Robert Platt
2024-07-18
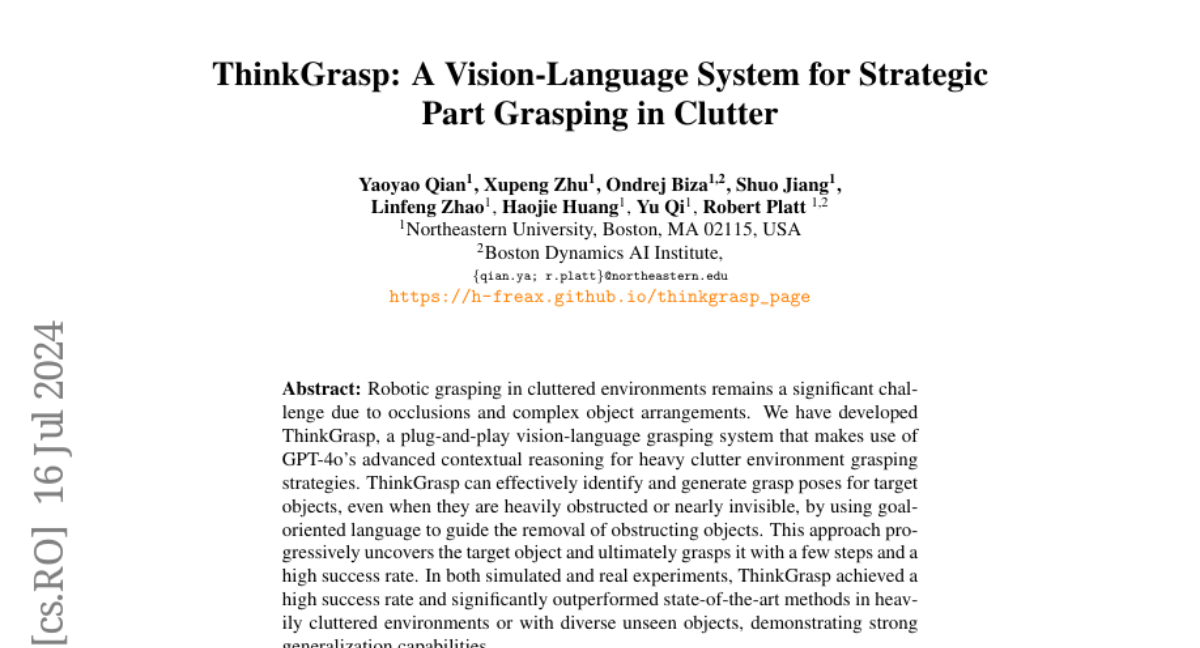
Summary
This paper introduces ThinkGrasp, a new system designed to help robots effectively grasp objects in messy and cluttered environments using advanced language understanding.
What's the problem?
Robotic grasping in cluttered spaces is difficult because objects can block each other, making it hard for robots to see and reach the target object. Traditional systems struggle with this problem, especially when the desired object is hidden or not clearly visible due to other items in the way.
What's the solution?
ThinkGrasp uses a combination of vision and language processing to identify and generate effective grasping strategies. It employs GPT-4o's advanced reasoning capabilities to understand the environment and plan how to remove obstacles. By using goal-oriented language, ThinkGrasp can progressively uncover the target object and successfully grasp it with fewer attempts. The system has been tested in both simulated environments and real-world scenarios, achieving high success rates and outperforming existing methods.
Why it matters?
This research is important because it enhances the ability of robots to operate in real-world settings where clutter is common, such as homes or warehouses. By improving robotic grasping techniques, ThinkGrasp can lead to more efficient automation in various industries, making tasks like sorting, picking, and organizing much easier for robots.
Abstract
Robotic grasping in cluttered environments remains a significant challenge due to occlusions and complex object arrangements. We have developed ThinkGrasp, a plug-and-play vision-language grasping system that makes use of GPT-4o's advanced contextual reasoning for heavy clutter environment grasping strategies. ThinkGrasp can effectively identify and generate grasp poses for target objects, even when they are heavily obstructed or nearly invisible, by using goal-oriented language to guide the removal of obstructing objects. This approach progressively uncovers the target object and ultimately grasps it with a few steps and a high success rate. In both simulated and real experiments, ThinkGrasp achieved a high success rate and significantly outperformed state-of-the-art methods in heavily cluttered environments or with diverse unseen objects, demonstrating strong generalization capabilities.