Thinking in Space: How Multimodal Large Language Models See, Remember, and Recall Spaces
Jihan Yang, Shusheng Yang, Anjali W. Gupta, Rilyn Han, Li Fei-Fei, Saining Xie
2024-12-19
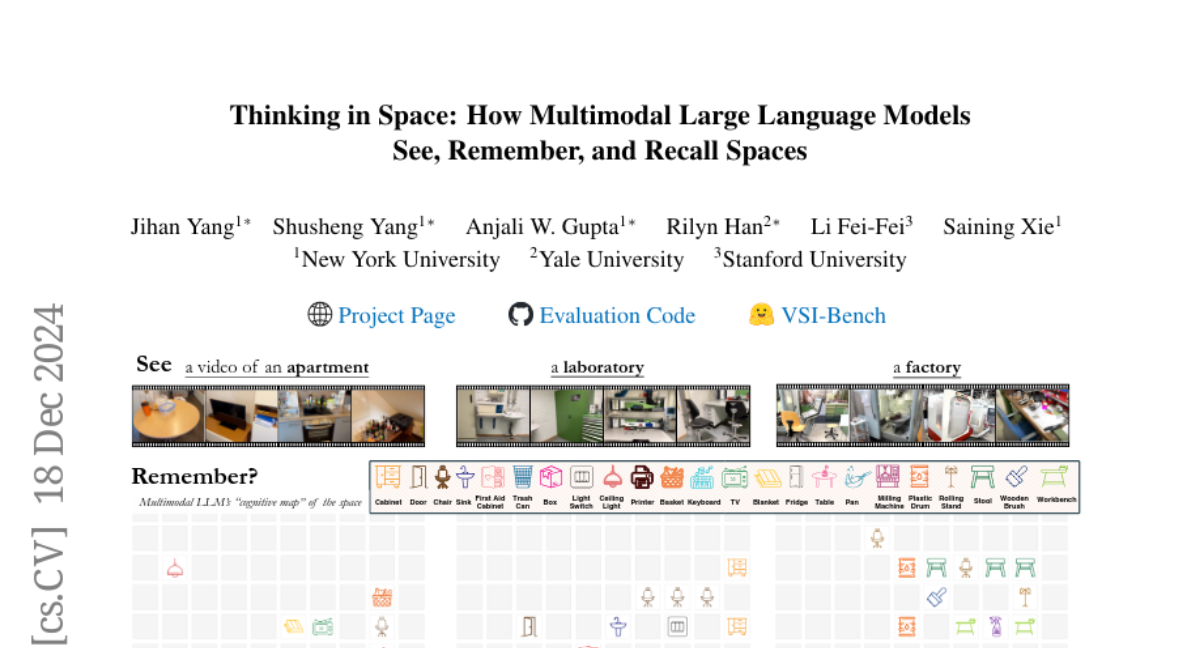
Summary
This paper talks about how Multimodal Large Language Models (MLLMs) can understand and remember spaces from videos, and it introduces a new benchmark to test their visual-spatial intelligence.
What's the problem?
Humans are good at remembering and understanding spaces based on what they see. However, it's unclear if AI models, especially MLLMs that analyze both text and video, can do the same. Many existing models struggle with spatial reasoning, which is the ability to understand the relationships between objects in space. This limitation can hinder their performance in tasks that require a good grasp of spatial information.
What's the solution?
The researchers developed a new benchmark called VSI-Bench, which includes over 5,000 questions and answers designed to test how well MLLMs can think about space. They found that while these models show some ability to understand spatial relationships, they still perform below human levels. The study also revealed that traditional reasoning techniques used in language models didn't help improve their spatial reasoning abilities. Instead, creating cognitive maps—visual representations of spaces—during question-answering led to better performance in understanding distances and relationships between objects.
Why it matters?
This research is important because it highlights the strengths and weaknesses of AI models when it comes to understanding spaces, which is essential for applications like robotics, virtual reality, and autonomous vehicles. By improving how these models think about space, we can make them more effective in real-world tasks that require spatial awareness.
Abstract
Humans possess the visual-spatial intelligence to remember spaces from sequential visual observations. However, can Multimodal Large Language Models (MLLMs) trained on million-scale video datasets also ``think in space'' from videos? We present a novel video-based visual-spatial intelligence benchmark (VSI-Bench) of over 5,000 question-answer pairs, and find that MLLMs exhibit competitive - though subhuman - visual-spatial intelligence. We probe models to express how they think in space both linguistically and visually and find that while spatial reasoning capabilities remain the primary bottleneck for MLLMs to reach higher benchmark performance, local world models and spatial awareness do emerge within these models. Notably, prevailing linguistic reasoning techniques (e.g., chain-of-thought, self-consistency, tree-of-thoughts) fail to improve performance, whereas explicitly generating cognitive maps during question-answering enhances MLLMs' spatial distance ability.