To CoT or not to CoT? Chain-of-thought helps mainly on math and symbolic reasoning
Zayne Sprague, Fangcong Yin, Juan Diego Rodriguez, Dongwei Jiang, Manya Wadhwa, Prasann Singhal, Xinyu Zhao, Xi Ye, Kyle Mahowald, Greg Durrett
2024-09-19
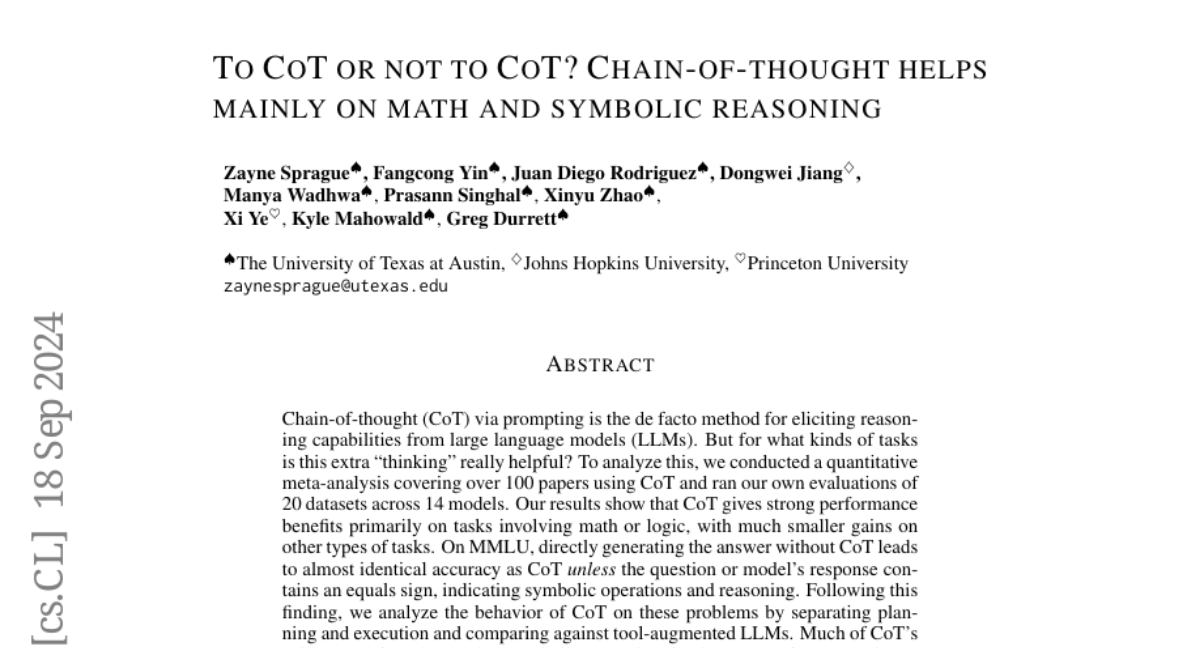
Summary
This paper examines how effective the Chain-of-Thought (CoT) prompting method is for helping large language models (LLMs) solve problems, particularly focusing on math and logic tasks.
What's the problem?
While CoT prompting is commonly used to improve reasoning in LLMs, it's unclear for which types of tasks it is most beneficial. Many existing studies have not clearly compared the effectiveness of CoT across different tasks, leading to uncertainty about when it is helpful and when it might not be necessary.
What's the solution?
The researchers conducted a thorough analysis of over 100 studies and evaluated 20 datasets across 14 different models. They found that CoT significantly improves performance mainly on math and logic tasks, while its benefits are much smaller for other types of tasks. They also discovered that directly generating answers without using CoT often yields similar accuracy unless the task involves symbolic reasoning, like equations. The study suggests that CoT should be used selectively to save resources while still maintaining performance.
Why it matters?
This research is important because it clarifies the situations where CoT is most effective, helping developers decide when to use this method with LLMs. By understanding how to apply CoT more effectively, AI systems can become better at solving complex problems, particularly in areas that require logical reasoning or mathematical skills.
Abstract
Chain-of-thought (CoT) via prompting is the de facto method for eliciting reasoning capabilities from large language models (LLMs). But for what kinds of tasks is this extra ``thinking'' really helpful? To analyze this, we conducted a quantitative meta-analysis covering over 100 papers using CoT and ran our own evaluations of 20 datasets across 14 models. Our results show that CoT gives strong performance benefits primarily on tasks involving math or logic, with much smaller gains on other types of tasks. On MMLU, directly generating the answer without CoT leads to almost identical accuracy as CoT unless the question or model's response contains an equals sign, indicating symbolic operations and reasoning. Following this finding, we analyze the behavior of CoT on these problems by separating planning and execution and comparing against tool-augmented LLMs. Much of CoT's gain comes from improving symbolic execution, but it underperforms relative to using a symbolic solver. Our results indicate that CoT can be applied selectively, maintaining performance while saving inference costs. Furthermore, they suggest a need to move beyond prompt-based CoT to new paradigms that better leverage intermediate computation across the whole range of LLM applications.