Toffee: Efficient Million-Scale Dataset Construction for Subject-Driven Text-to-Image Generation
Yufan Zhou, Ruiyi Zhang, Kaizhi Zheng, Nanxuan Zhao, Jiuxiang Gu, Zichao Wang, Xin Eric Wang, Tong Sun
2024-06-14
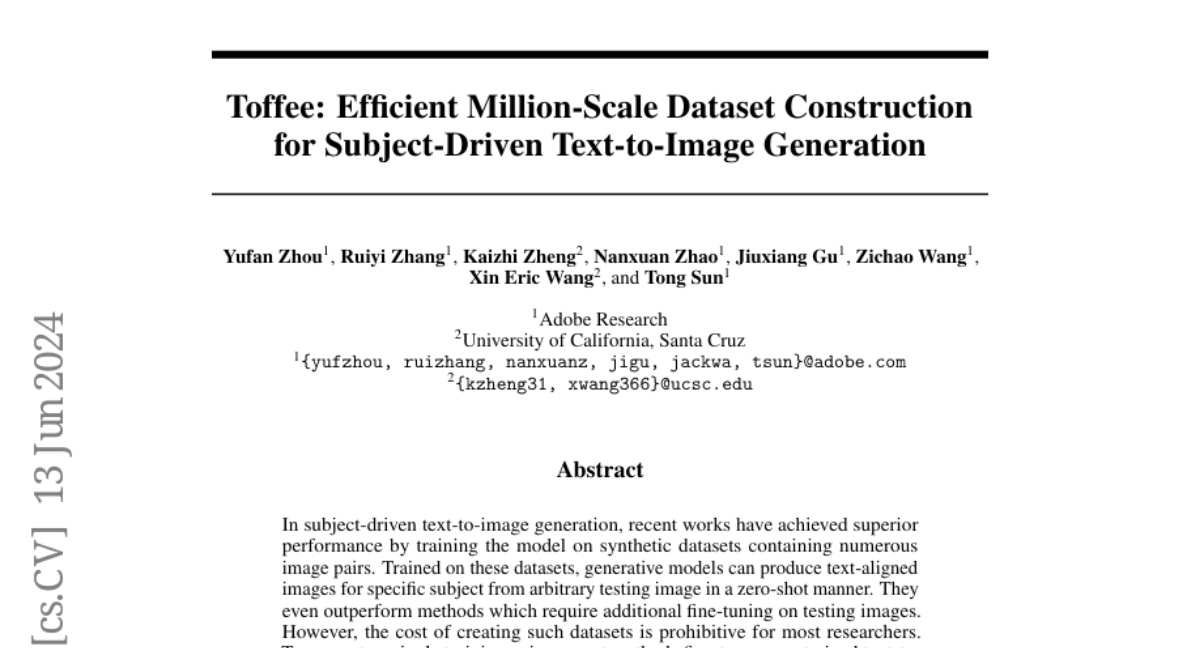
Summary
This paper introduces Toffee, a new method for creating large datasets that help AI models generate images based on specific subjects. It focuses on making the dataset construction process more efficient and less time-consuming.
What's the problem?
Creating datasets for training text-to-image models is often very expensive and time-consuming. Traditional methods require a lot of computational power, sometimes needing hundreds of thousands of GPU hours just to generate a single training pair of images and text. This makes it difficult for many researchers to build the large-scale datasets necessary for effective AI training.
What's the solution?
To solve this problem, the authors developed Toffee, which allows for the construction of large datasets without needing to fine-tune individual subject images. Instead, after pre-training two generative models, they can generate an unlimited number of high-quality image samples efficiently. They created a dataset containing 5 million image pairs, text prompts, and masks, which is five times larger than previous datasets but requires significantly less computational cost to produce.
Why it matters?
This research is important because it makes it easier and cheaper for researchers to create the large datasets needed for training AI models in generating images from text. By improving the efficiency of dataset construction, Toffee can help advance the field of AI image generation, enabling more innovative applications in art, design, and other areas where visual content is essential.
Abstract
In subject-driven text-to-image generation, recent works have achieved superior performance by training the model on synthetic datasets containing numerous image pairs. Trained on these datasets, generative models can produce text-aligned images for specific subject from arbitrary testing image in a zero-shot manner. They even outperform methods which require additional fine-tuning on testing images. However, the cost of creating such datasets is prohibitive for most researchers. To generate a single training pair, current methods fine-tune a pre-trained text-to-image model on the subject image to capture fine-grained details, then use the fine-tuned model to create images for the same subject based on creative text prompts. Consequently, constructing a large-scale dataset with millions of subjects can require hundreds of thousands of GPU hours. To tackle this problem, we propose Toffee, an efficient method to construct datasets for subject-driven editing and generation. Specifically, our dataset construction does not need any subject-level fine-tuning. After pre-training two generative models, we are able to generate infinite number of high-quality samples. We construct the first large-scale dataset for subject-driven image editing and generation, which contains 5 million image pairs, text prompts, and masks. Our dataset is 5 times the size of previous largest dataset, yet our cost is tens of thousands of GPU hours lower. To test the proposed dataset, we also propose a model which is capable of both subject-driven image editing and generation. By simply training the model on our proposed dataset, it obtains competitive results, illustrating the effectiveness of the proposed dataset construction framework.