Token Erasure as a Footprint of Implicit Vocabulary Items in LLMs
Sheridan Feucht, David Atkinson, Byron Wallace, David Bau
2024-07-02
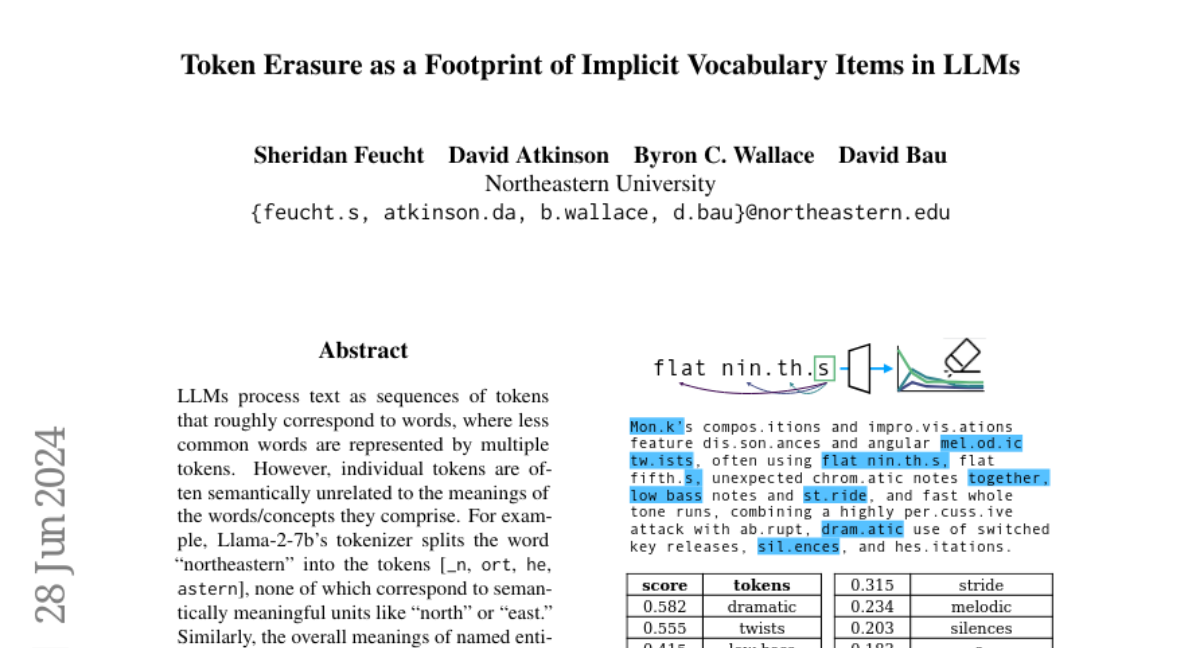
Summary
This paper talks about how large language models (LLMs) understand and represent words and phrases, particularly focusing on a phenomenon called 'token erasure' that helps reveal the model's hidden vocabulary.
What's the problem?
The main issue is that LLMs process text using tokens, which are small pieces of words. However, these tokens often do not reflect the actual meanings of the words they form. For example, the word 'northeastern' is broken down into tokens that don't relate to its meaning, making it hard to understand how the model recognizes complex words and phrases.
What's the solution?
The authors discovered that when LLMs process multi-token words or named entities, they exhibit an 'erasure' effect where information about earlier tokens is quickly forgotten in the model's early layers. To explore this further, they proposed a method to analyze how these tokens are represented across different layers of the model, allowing researchers to uncover the model's implicit vocabulary—essentially what the model 'knows' about language.
Why it matters?
This research is significant because it provides insights into how LLMs form their understanding of language. By revealing the implicit vocabulary and understanding token erasure, developers can improve LLM transparency and performance, making AI systems more reliable and effective in understanding human language.
Abstract
LLMs process text as sequences of tokens that roughly correspond to words, where less common words are represented by multiple tokens. However, individual tokens are often semantically unrelated to the meanings of the words/concepts they comprise. For example, Llama-2-7b's tokenizer splits the word "northeastern" into the tokens ['_n', 'ort', 'he', 'astern'], none of which correspond to semantically meaningful units like "north" or "east." Similarly, the overall meanings of named entities like "Neil Young" and multi-word expressions like "break a leg" cannot be directly inferred from their constituent tokens. Mechanistically, how do LLMs convert such arbitrary groups of tokens into useful higher-level representations? In this work, we find that last token representations of named entities and multi-token words exhibit a pronounced "erasure" effect, where information about previous and current tokens is rapidly forgotten in early layers. Using this observation, we propose a method to "read out" the implicit vocabulary of an autoregressive LLM by examining differences in token representations across layers, and present results of this method for Llama-2-7b and Llama-3-8B. To our knowledge, this is the first attempt to probe the implicit vocabulary of an LLM.