TokenFormer: Rethinking Transformer Scaling with Tokenized Model Parameters
Haiyang Wang, Yue Fan, Muhammad Ferjad Naeem, Yongqin Xian, Jan Eric Lenssen, Liwei Wang, Federico Tombari, Bernt Schiele
2024-10-31
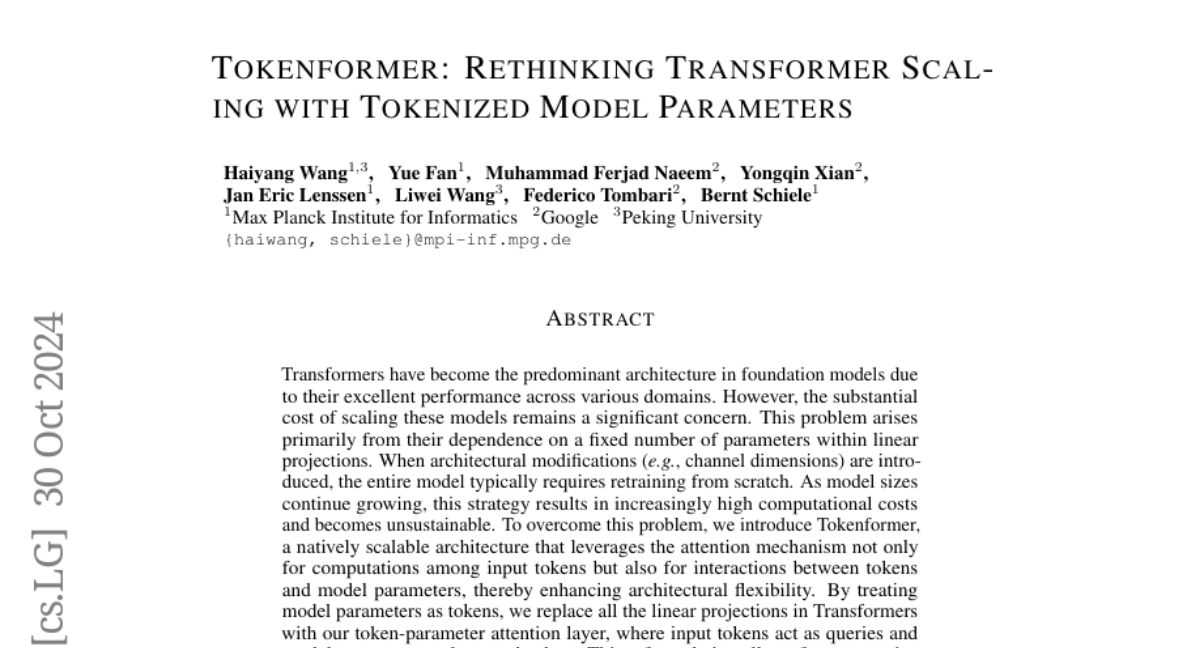
Summary
This paper presents TokenFormer, a new way to scale transformer models by using tokenized model parameters. This approach aims to make it easier and cheaper to train large language models (LLMs) without losing performance.
What's the problem?
Scaling transformer models is expensive and complicated because they rely on a fixed number of parameters. When changes are made to the model's architecture, like adjusting its size, the entire model often needs to be retrained from scratch. This process can become very costly and is not sustainable as models continue to grow larger.
What's the solution?
TokenFormer solves this problem by treating model parameters as tokens, which allows for more flexible scaling. Instead of using linear projections that require complete retraining, TokenFormer uses an attention mechanism that lets the model interact with these tokenized parameters directly. This means that new parameters can be added incrementally without starting over, allowing the model to grow from 124 million to 1.4 billion parameters efficiently. The authors demonstrate that this method achieves similar performance to traditional training methods while significantly reducing training costs.
Why it matters?
This research is important because it provides a more efficient way to develop large language models, which are crucial for many AI applications. By making it easier and cheaper to scale these models, TokenFormer can help researchers and developers create more powerful AI systems without the high computational costs typically associated with training large models.
Abstract
Transformers have become the predominant architecture in foundation models due to their excellent performance across various domains. However, the substantial cost of scaling these models remains a significant concern. This problem arises primarily from their dependence on a fixed number of parameters within linear projections. When architectural modifications (e.g., channel dimensions) are introduced, the entire model typically requires retraining from scratch. As model sizes continue growing, this strategy results in increasingly high computational costs and becomes unsustainable. To overcome this problem, we introduce TokenFormer, a natively scalable architecture that leverages the attention mechanism not only for computations among input tokens but also for interactions between tokens and model parameters, thereby enhancing architectural flexibility. By treating model parameters as tokens, we replace all the linear projections in Transformers with our token-parameter attention layer, where input tokens act as queries and model parameters as keys and values. This reformulation allows for progressive and efficient scaling without necessitating retraining from scratch. Our model scales from 124M to 1.4B parameters by incrementally adding new key-value parameter pairs, achieving performance comparable to Transformers trained from scratch while greatly reducing training costs. Code and models are available at https://github.com/Haiyang-W/TokenFormer.