TOMATO: Assessing Visual Temporal Reasoning Capabilities in Multimodal Foundation Models
Ziyao Shangguan, Chuhan Li, Yuxuan Ding, Yanan Zheng, Yilun Zhao, Tesca Fitzgerald, Arman Cohan
2024-11-04
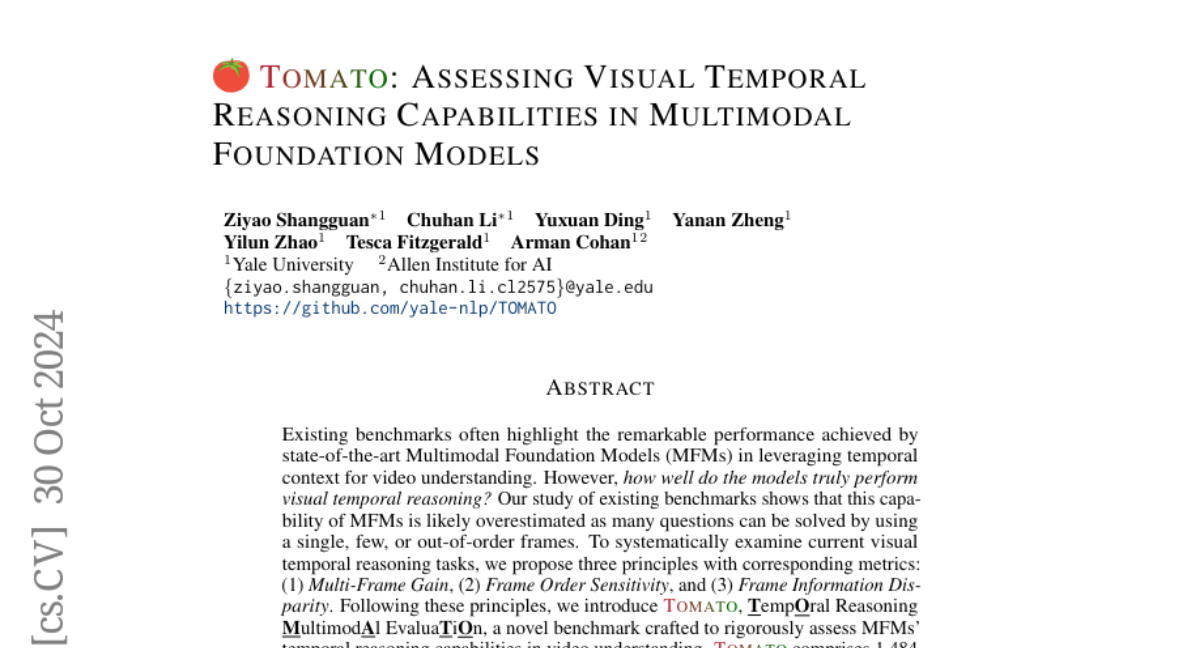
Summary
This paper introduces TOMATO, a new evaluation framework designed to test how well Multimodal Foundation Models (MFMs) can understand and reason about videos over time. It aims to provide a clearer picture of these models' capabilities in visual temporal reasoning.
What's the problem?
Current benchmarks suggest that MFMs perform exceptionally well at understanding video content, but this might not be true. Many tasks can be solved using only a few frames or even out-of-order frames, which means the models might not be as good at reasoning about the sequence of events in videos as previously thought.
What's the solution?
To address this issue, the authors propose TOMATO, which is based on three principles that help assess how well MFMs handle visual temporal reasoning. They created a benchmark with 1,484 questions covering various tasks related to video understanding, using a large dataset of 1,417 videos. This comprehensive evaluation helps reveal the gaps between human performance and what these models can achieve.
Why it matters?
This research is important because it challenges the current understanding of how well AI models can reason about video content over time. By providing a rigorous way to evaluate these capabilities, TOMATO encourages improvements in future AI systems, ultimately leading to better performance in applications like video analysis and understanding human actions.
Abstract
Existing benchmarks often highlight the remarkable performance achieved by state-of-the-art Multimodal Foundation Models (MFMs) in leveraging temporal context for video understanding. However, how well do the models truly perform visual temporal reasoning? Our study of existing benchmarks shows that this capability of MFMs is likely overestimated as many questions can be solved by using a single, few, or out-of-order frames. To systematically examine current visual temporal reasoning tasks, we propose three principles with corresponding metrics: (1) Multi-Frame Gain, (2) Frame Order Sensitivity, and (3) Frame Information Disparity. Following these principles, we introduce TOMATO, Temporal Reasoning Multimodal Evaluation, a novel benchmark crafted to rigorously assess MFMs' temporal reasoning capabilities in video understanding. TOMATO comprises 1,484 carefully curated, human-annotated questions spanning six tasks (i.e., action count, direction, rotation, shape & trend, velocity & frequency, and visual cues), applied to 1,417 videos, including 805 self-recorded and -generated videos, that encompass human-centric, real-world, and simulated scenarios. Our comprehensive evaluation reveals a human-model performance gap of 57.3% with the best-performing model. Moreover, our in-depth analysis uncovers more fundamental limitations beyond this gap in current MFMs. While they can accurately recognize events in isolated frames, they fail to interpret these frames as a continuous sequence. We believe TOMATO will serve as a crucial testbed for evaluating the next-generation MFMs and as a call to the community to develop AI systems capable of comprehending human world dynamics through the video modality.