ToolSandbox: A Stateful, Conversational, Interactive Evaluation Benchmark for LLM Tool Use Capabilities
Jiarui Lu, Thomas Holleis, Yizhe Zhang, Bernhard Aumayer, Feng Nan, Felix Bai, Shuang Ma, Shen Ma, Mengyu Li, Guoli Yin, Zirui Wang, Ruoming Pang
2024-08-12
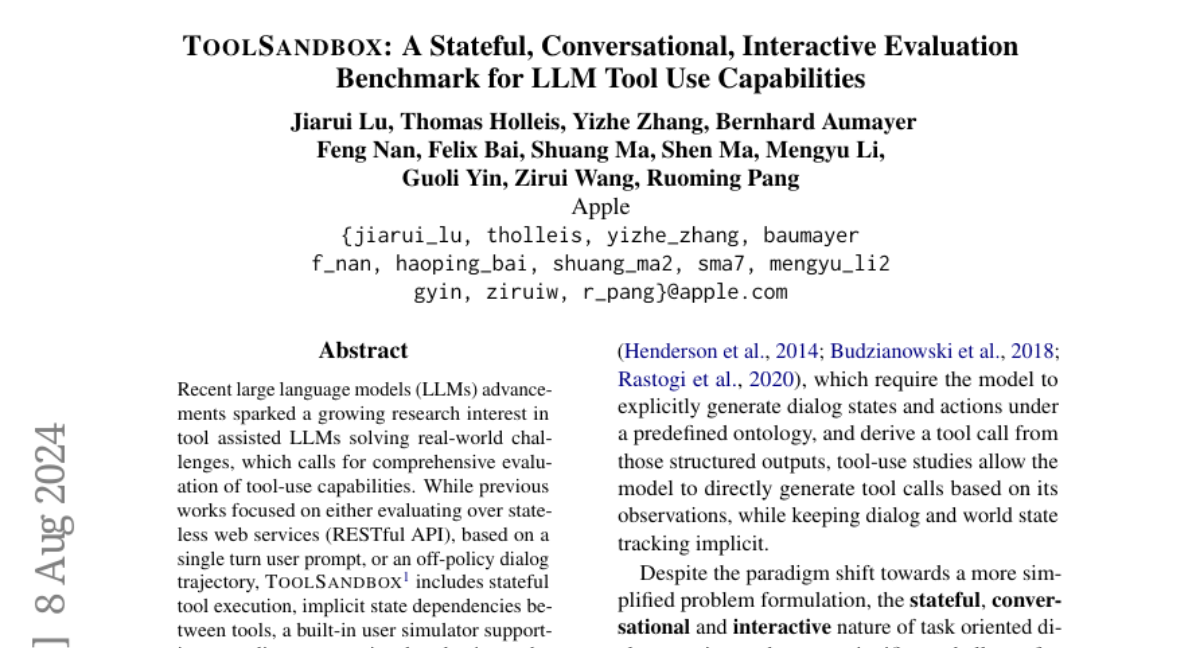
Summary
This paper introduces ToolSandbox, a new evaluation framework designed to assess how well large language models (LLMs) can use tools in interactive and real-world scenarios.
What's the problem?
As LLMs become more advanced, there is a need to evaluate their ability to use external tools effectively. Previous evaluation methods often focused on simple tasks or did not consider the ongoing context of interactions, making it hard to understand how well these models can handle complex, multi-step problems in real-life situations.
What's the solution?
ToolSandbox addresses these challenges by creating a stateful, conversational, and interactive environment where LLMs can perform tasks that require using tools. It tracks the model's previous actions (stateful), allows for natural conversation (conversational), and lets the model actively engage with the tasks (interactive). This means that LLMs can make decisions based on past interactions and handle complex tasks that involve multiple steps and dependencies between tools. The authors tested various models using this framework and found significant performance gaps, highlighting areas where even the best models struggle.
Why it matters?
This research is important because it provides a more realistic way to evaluate how LLMs can assist users in solving real-world problems. By understanding the strengths and weaknesses of these models in tool use, developers can improve AI systems to be more effective and reliable in practical applications, such as customer service or automated assistance.
Abstract
Recent large language models (LLMs) advancements sparked a growing research interest in tool assisted LLMs solving real-world challenges, which calls for comprehensive evaluation of tool-use capabilities. While previous works focused on either evaluating over stateless web services (RESTful API), based on a single turn user prompt, or an off-policy dialog trajectory, ToolSandbox includes stateful tool execution, implicit state dependencies between tools, a built-in user simulator supporting on-policy conversational evaluation and a dynamic evaluation strategy for intermediate and final milestones over an arbitrary trajectory. We show that open source and proprietary models have a significant performance gap, and complex tasks like State Dependency, Canonicalization and Insufficient Information defined in ToolSandbox are challenging even the most capable SOTA LLMs, providing brand-new insights into tool-use LLM capabilities. ToolSandbox evaluation framework is released at https://github.com/apple/ToolSandbox