ToVo: Toxicity Taxonomy via Voting
Tinh Son Luong, Thanh-Thien Le, Thang Viet Doan, Linh Ngo Van, Thien Huu Nguyen, Diep Thi-Ngoc Nguyen
2024-06-24
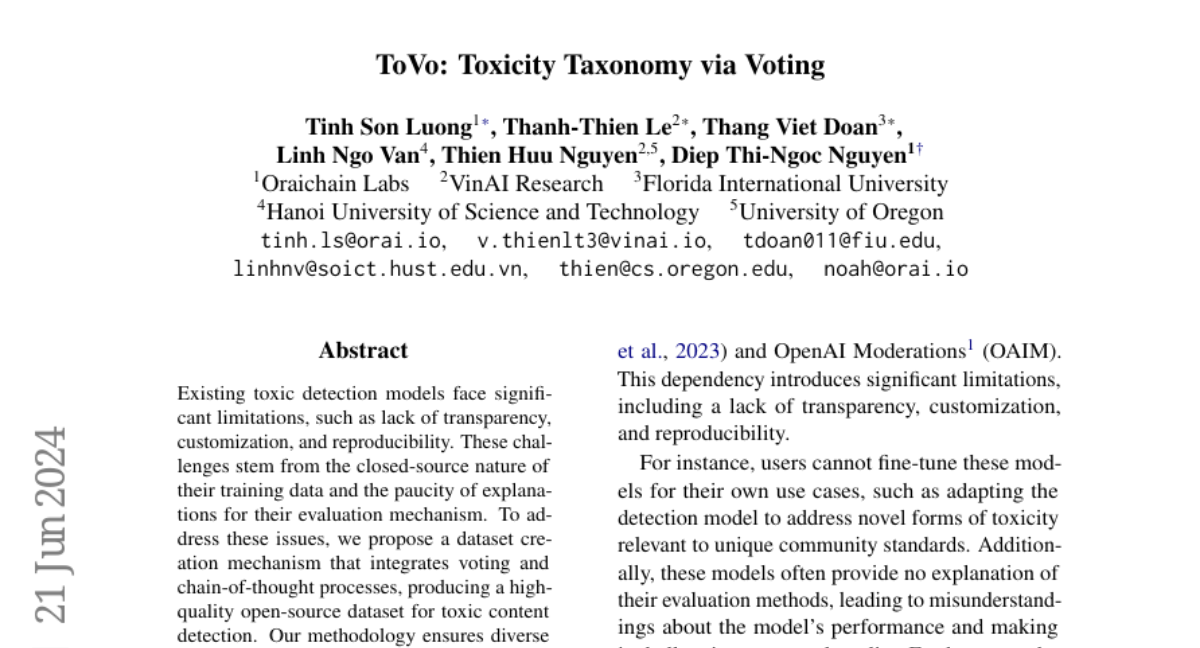
Summary
This paper introduces ToVo, a new method for creating a dataset that helps in detecting toxic content online. It focuses on making the process more transparent and customizable, allowing for better understanding and adaptation of toxicity detection models.
What's the problem?
Current models for detecting toxic content often have issues like being hard to understand, not allowing for customization, and lacking reproducibility. These problems arise because their training data is typically closed-source and there are not enough explanations about how they evaluate toxicity.
What's the solution?
The authors developed a new approach to create a dataset that uses voting and chain-of-thought reasoning. This method produces a high-quality open-source dataset that includes various classification metrics for each sample, along with scores and explanations for why something is classified as toxic. They then used this dataset to train their model and compared its performance with existing toxicity detectors.
Why it matters?
This research is important because it promotes transparency and adaptability in toxicity detection models. By providing an open-source dataset and a clear methodology, it allows developers to fine-tune models for specific needs, leading to more effective moderation of toxic content online.
Abstract
Existing toxic detection models face significant limitations, such as lack of transparency, customization, and reproducibility. These challenges stem from the closed-source nature of their training data and the paucity of explanations for their evaluation mechanism. To address these issues, we propose a dataset creation mechanism that integrates voting and chain-of-thought processes, producing a high-quality open-source dataset for toxic content detection. Our methodology ensures diverse classification metrics for each sample and includes both classification scores and explanatory reasoning for the classifications. We utilize the dataset created through our proposed mechanism to train our model, which is then compared against existing widely-used detectors. Our approach not only enhances transparency and customizability but also facilitates better fine-tuning for specific use cases. This work contributes a robust framework for developing toxic content detection models, emphasizing openness and adaptability, thus paving the way for more effective and user-specific content moderation solutions.