Toward Guidance-Free AR Visual Generation via Condition Contrastive Alignment
Huayu Chen, Hang Su, Peize Sun, Jun Zhu
2024-10-18
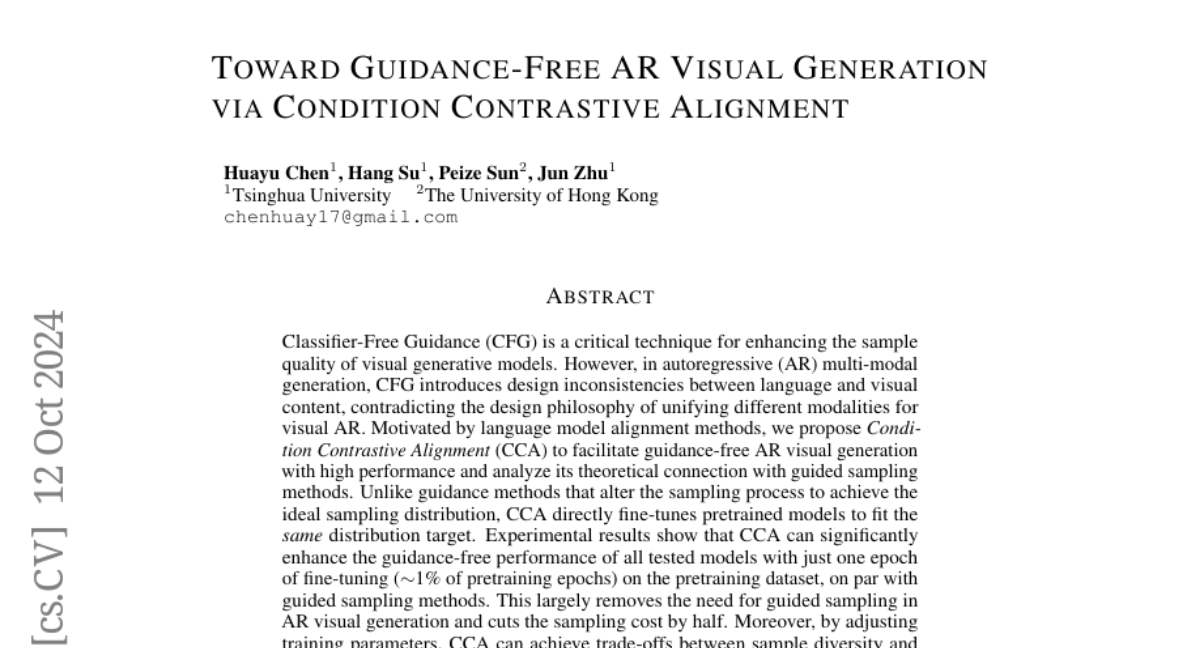
Summary
This paper presents a new method called Condition Contrastive Alignment (CCA) that improves visual generation in autoregressive models without needing additional guidance, making the process more efficient and effective.
What's the problem?
In visual generation, existing techniques like Classifier-Free Guidance (CFG) help improve the quality of generated images but can create inconsistencies between how language and visuals are processed. This can make it difficult to unify different types of information, which is important for creating coherent visual content.
What's the solution?
To solve this issue, the authors propose CCA, which fine-tunes pre-trained models directly to improve their performance without altering the sampling process. This method uses a contrastive approach to compare positive and negative conditions related to images, allowing the model to learn from existing data without needing extra datasets. The authors found that CCA can enhance the performance of visual generation models significantly after just one training session, achieving results comparable to those using guided methods while cutting the sampling cost in half.
Why it matters?
This research is important because it simplifies the process of generating high-quality visual content by removing the need for complex guidance techniques. By making visual generation more efficient, CCA can help advance various applications in fields like computer graphics, animation, and virtual reality, where creating realistic visuals quickly is essential.
Abstract
Classifier-Free Guidance (CFG) is a critical technique for enhancing the sample quality of visual generative models. However, in autoregressive (AR) multi-modal generation, CFG introduces design inconsistencies between language and visual content, contradicting the design philosophy of unifying different modalities for visual AR. Motivated by language model alignment methods, we propose Condition Contrastive Alignment (CCA) to facilitate guidance-free AR visual generation with high performance and analyze its theoretical connection with guided sampling methods. Unlike guidance methods that alter the sampling process to achieve the ideal sampling distribution, CCA directly fine-tunes pretrained models to fit the same distribution target. Experimental results show that CCA can significantly enhance the guidance-free performance of all tested models with just one epoch of fine-tuning (sim 1\% of pretraining epochs) on the pretraining dataset, on par with guided sampling methods. This largely removes the need for guided sampling in AR visual generation and cuts the sampling cost by half. Moreover, by adjusting training parameters, CCA can achieve trade-offs between sample diversity and fidelity similar to CFG. This experimentally confirms the strong theoretical connection between language-targeted alignment and visual-targeted guidance methods, unifying two previously independent research fields. Code and model weights: https://github.com/thu-ml/CCA.