Towards a Unified View of Preference Learning for Large Language Models: A Survey
Bofei Gao, Feifan Song, Yibo Miao, Zefan Cai, Zhe Yang, Liang Chen, Helan Hu, Runxin Xu, Qingxiu Dong, Ce Zheng, Wen Xiao, Ge Zhang, Daoguang Zan, Keming Lu, Bowen Yu, Dayiheng Liu, Zeyu Cui, Jian Yang, Lei Sha, Houfeng Wang, Zhifang Sui, Peiyi Wang
2024-09-10
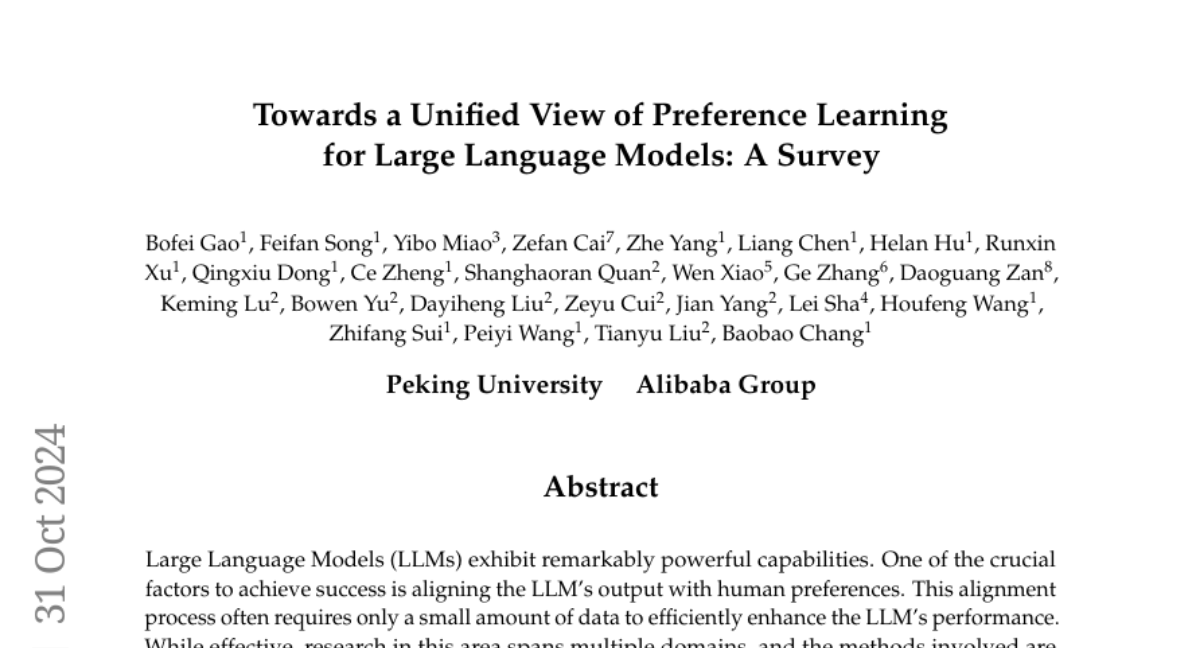
Summary
This paper talks about how to improve the way large language models (LLMs) are trained to better match human preferences by reviewing and organizing different strategies used in preference learning.
What's the problem?
While LLMs are powerful, they need to produce outputs that align with what humans want. Current methods for training these models to understand human preferences are spread across different areas and can be complicated, making it hard to see how they connect and how effective they really are.
What's the solution?
The authors break down existing methods into four main parts: model, data, feedback, and algorithm. By organizing these components into a unified framework, they provide a clearer understanding of how different strategies work together. They also include examples of popular algorithms to help readers grasp the concepts better. This structured approach aims to highlight the strengths of various methods and suggest ways to improve preference alignment in LLMs.
Why it matters?
This research is important because it helps researchers and developers create better LLMs that can understand and respond to human needs more effectively. By improving how these models are trained, we can enhance their performance in real-world applications, such as chatbots, content generation, and other AI tools.
Abstract
Large Language Models (LLMs) exhibit remarkably powerful capabilities. One of the crucial factors to achieve success is aligning the LLM's output with human preferences. This alignment process often requires only a small amount of data to efficiently enhance the LLM's performance. While effective, research in this area spans multiple domains, and the methods involved are relatively complex to understand. The relationships between different methods have been under-explored, limiting the development of the preference alignment. In light of this, we break down the existing popular alignment strategies into different components and provide a unified framework to study the current alignment strategies, thereby establishing connections among them. In this survey, we decompose all the strategies in preference learning into four components: model, data, feedback, and algorithm. This unified view offers an in-depth understanding of existing alignment algorithms and also opens up possibilities to synergize the strengths of different strategies. Furthermore, we present detailed working examples of prevalent existing algorithms to facilitate a comprehensive understanding for the readers. Finally, based on our unified perspective, we explore the challenges and future research directions for aligning large language models with human preferences.