Towards Best Practices for Open Datasets for LLM Training
Stefan Baack, Stella Biderman, Kasia Odrozek, Aviya Skowron, Ayah Bdeir, Jillian Bommarito, Jennifer Ding, Maximilian Gahntz, Paul Keller, Pierre-Carl Langlais, Greg Lindahl, Sebastian Majstorovic, Nik Marda, Guilherme Penedo, Maarten Van Segbroeck, Jennifer Wang, Leandro von Werra, Mitchell Baker, Julie Belião, Kasia Chmielinski, Marzieh Fadaee, Lisa Gutermuth
2025-01-16
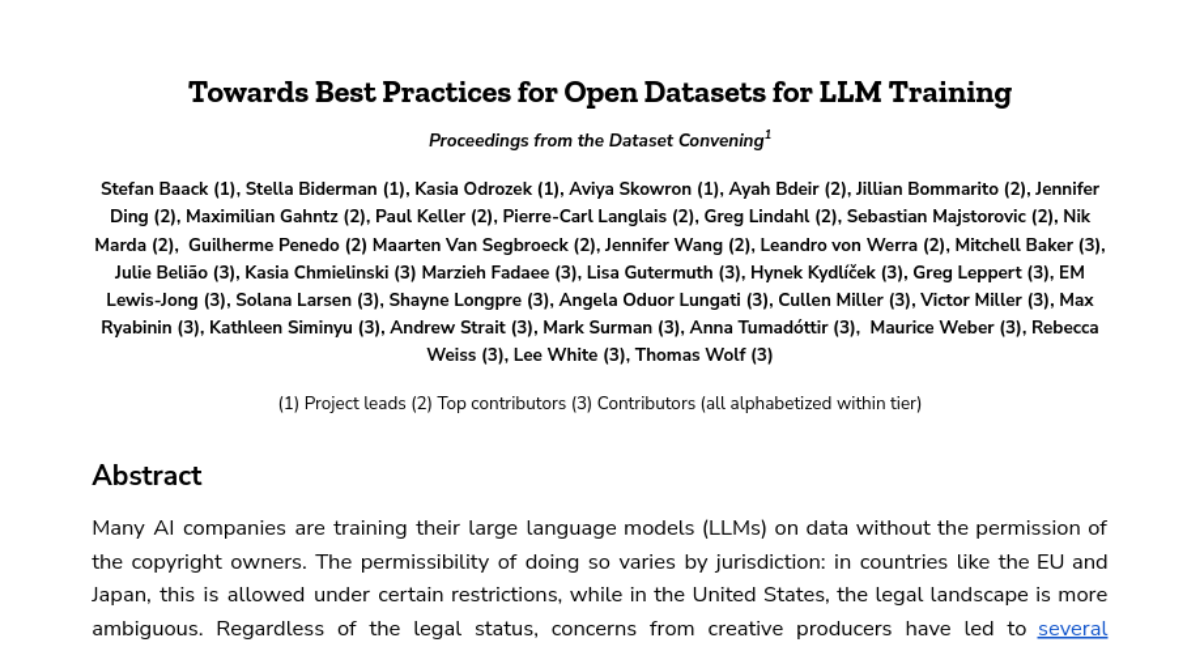
Summary
This paper talks about the challenges and potential solutions for creating open datasets to train large language models (LLMs) without violating copyright laws or facing legal issues.
What's the problem?
Many AI companies are using copyrighted data without permission to train their LLMs, which has led to lawsuits and a trend of hiding information about training datasets. This lack of transparency makes it hard for researchers and others to understand how these AI models work. While using open access and public domain data could solve this, there aren't any large-scale models trained this way yet because it's really hard to put together such a dataset.
What's the solution?
The paper suggests working towards a future where AI systems can be trained on openly licensed data that is carefully chosen and managed. This requires teamwork between legal experts, tech specialists, and policymakers. They need to improve how data is labeled and organized, make it easier to turn physical records into digital ones, and encourage a culture of openness. The goal is to create datasets that are legal to use, well-documented, and diverse.
Why it matters?
This matters because it could make AI development more transparent and fair. If we can create good open datasets for training LLMs, it would help researchers understand these models better, make it easier for more people to develop AI responsibly, and reduce legal risks. It could lead to AI systems that are more trustworthy and beneficial to society, while also respecting the rights of content creators.
Abstract
Many AI companies are training their large language models (LLMs) on data without the permission of the copyright owners. The permissibility of doing so varies by jurisdiction: in countries like the EU and Japan, this is allowed under certain restrictions, while in the United States, the legal landscape is more ambiguous. Regardless of the legal status, concerns from creative producers have led to several high-profile copyright lawsuits, and the threat of litigation is commonly cited as a reason for the recent trend towards minimizing the information shared about training datasets by both corporate and public interest actors. This trend in limiting data information causes harm by hindering transparency, accountability, and innovation in the broader ecosystem by denying researchers, auditors, and impacted individuals access to the information needed to understand AI models. While this could be mitigated by training language models on open access and public domain data, at the time of writing, there are no such models (trained at a meaningful scale) due to the substantial technical and sociological challenges in assembling the necessary corpus. These challenges include incomplete and unreliable metadata, the cost and complexity of digitizing physical records, and the diverse set of legal and technical skills required to ensure relevance and responsibility in a quickly changing landscape. Building towards a future where AI systems can be trained on openly licensed data that is responsibly curated and governed requires collaboration across legal, technical, and policy domains, along with investments in metadata standards, digitization, and fostering a culture of openness.