Towards Diverse and Efficient Audio Captioning via Diffusion Models
Manjie Xu, Chenxing Li, Xinyi Tu, Yong Ren, Ruibo Fu, Wei Liang, Dong Yu
2024-09-19
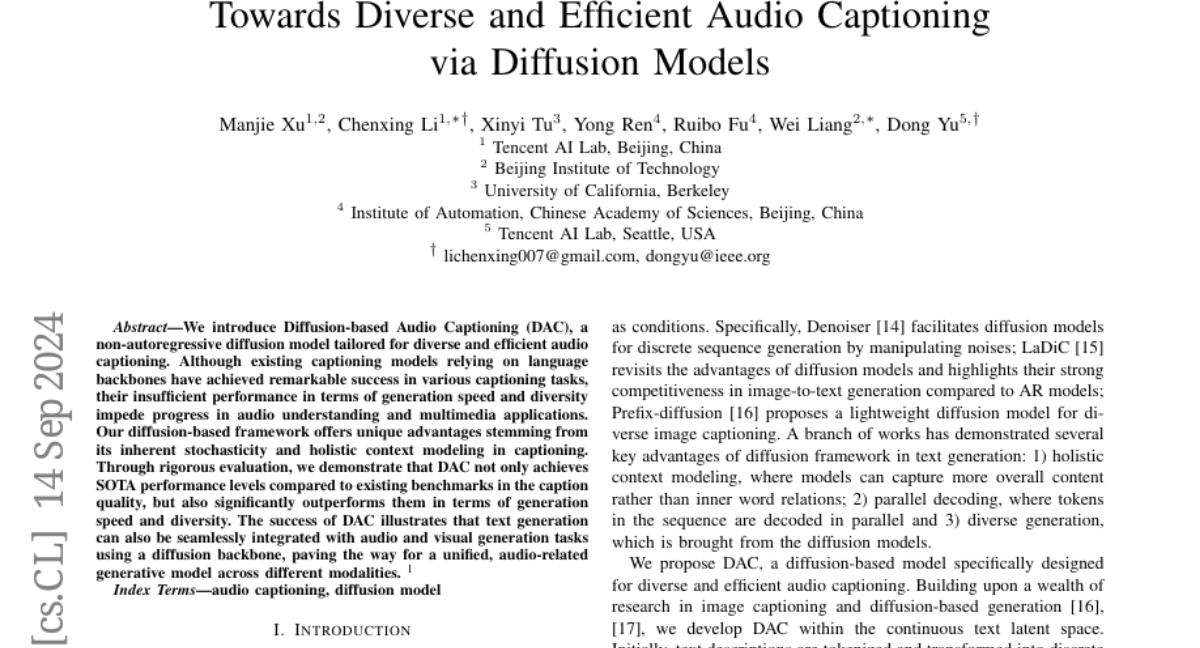
Summary
This paper introduces Diffusion-based Audio Captioning (DAC), a new model designed to create diverse and efficient captions for audio content using advanced diffusion techniques.
What's the problem?
Existing audio captioning models that rely on language processing often struggle with two main issues: they can be slow in generating captions and lack diversity in the captions they produce. This limits their effectiveness in understanding audio and applying it in multimedia projects, making it difficult to create engaging and varied content.
What's the solution?
The researchers developed DAC, which is a non-autoregressive model that uses diffusion methods to generate captions. This approach allows DAC to produce high-quality captions more quickly and with greater variety compared to traditional models. The model benefits from its ability to understand the overall context of the audio, leading to better caption generation. Through rigorous testing, DAC showed superior performance in both speed and the quality of captions when compared to existing benchmarks.
Why it matters?
This research is significant because it enhances how we can automatically describe audio content, which is crucial for applications like video production, accessibility for the hearing impaired, and content creation. By improving the efficiency and diversity of audio captioning, DAC opens up new possibilities for how we interact with and utilize audio in various media.
Abstract
We introduce Diffusion-based Audio Captioning (DAC), a non-autoregressive diffusion model tailored for diverse and efficient audio captioning. Although existing captioning models relying on language backbones have achieved remarkable success in various captioning tasks, their insufficient performance in terms of generation speed and diversity impede progress in audio understanding and multimedia applications. Our diffusion-based framework offers unique advantages stemming from its inherent stochasticity and holistic context modeling in captioning. Through rigorous evaluation, we demonstrate that DAC not only achieves SOTA performance levels compared to existing benchmarks in the caption quality, but also significantly outperforms them in terms of generation speed and diversity. The success of DAC illustrates that text generation can also be seamlessly integrated with audio and visual generation tasks using a diffusion backbone, paving the way for a unified, audio-related generative model across different modalities.