Towards Physically Plausible Video Generation via VLM Planning
Xindi Yang, Baolu Li, Yiming Zhang, Zhenfei Yin, Lei Bai, Liqian Ma, Zhiyong Wang, Jianfei Cai, Tien-Tsin Wong, Huchuan Lu, Xu Jia
2025-04-03
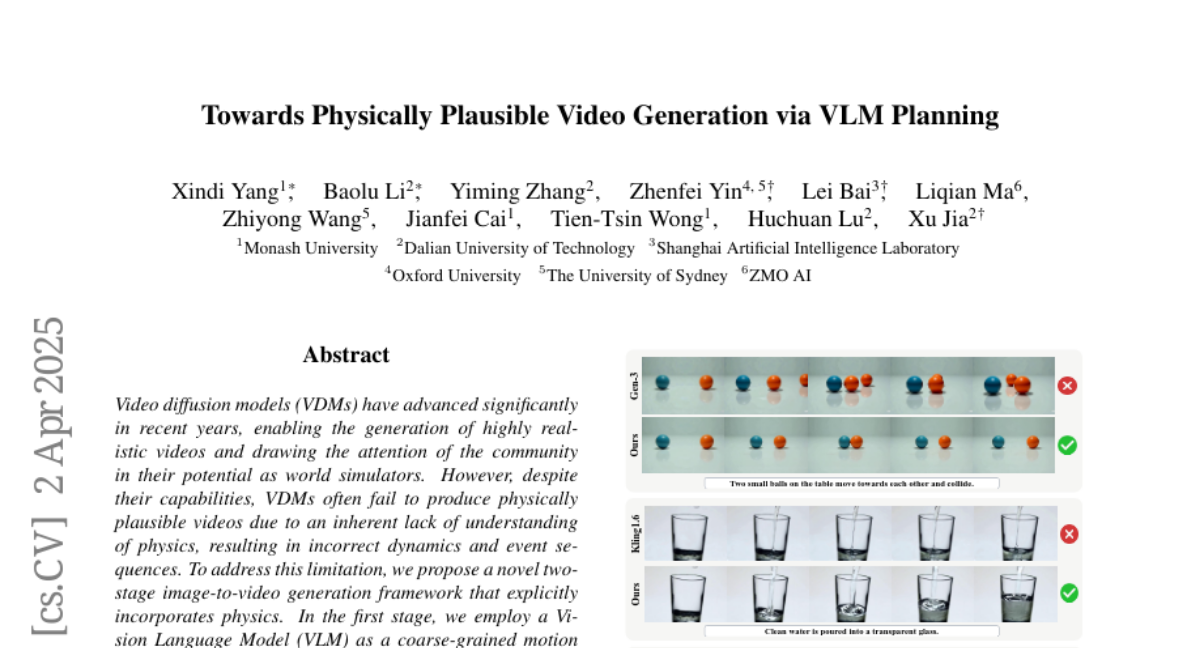
Summary
This paper explores how to make AI-generated videos more realistic by teaching the AI to understand and follow the laws of physics.
What's the problem?
AI-generated videos often look unrealistic because they don't obey the laws of physics, like objects moving in strange ways.
What's the solution?
The researchers created a system that first uses an AI model to plan out the basic movements in the video, making sure they follow physics. Then, another AI model fills in the details to create a realistic-looking video.
Why it matters?
This work matters because it can lead to AI-generated videos that are more believable and useful for things like simulations and virtual reality.
Abstract
Video diffusion models (VDMs) have advanced significantly in recent years, enabling the generation of highly realistic videos and drawing the attention of the community in their potential as world simulators. However, despite their capabilities, VDMs often fail to produce physically plausible videos due to an inherent lack of understanding of physics, resulting in incorrect dynamics and event sequences. To address this limitation, we propose a novel two-stage image-to-video generation framework that explicitly incorporates physics. In the first stage, we employ a Vision Language Model (VLM) as a coarse-grained motion planner, integrating chain-of-thought and physics-aware reasoning to predict a rough motion trajectories/changes that approximate real-world physical dynamics while ensuring the inter-frame consistency. In the second stage, we use the predicted motion trajectories/changes to guide the video generation of a VDM. As the predicted motion trajectories/changes are rough, noise is added during inference to provide freedom to the VDM in generating motion with more fine details. Extensive experimental results demonstrate that our framework can produce physically plausible motion, and comparative evaluations highlight the notable superiority of our approach over existing methods. More video results are available on our Project Page: https://madaoer.github.io/projects/physically_plausible_video_generation.