Towards Predicting Temporal Changes in a Patient's Chest X-ray Images based on Electronic Health Records
Daeun Kyung, Junu Kim, Tackeun Kim, Edward Choi
2024-09-17
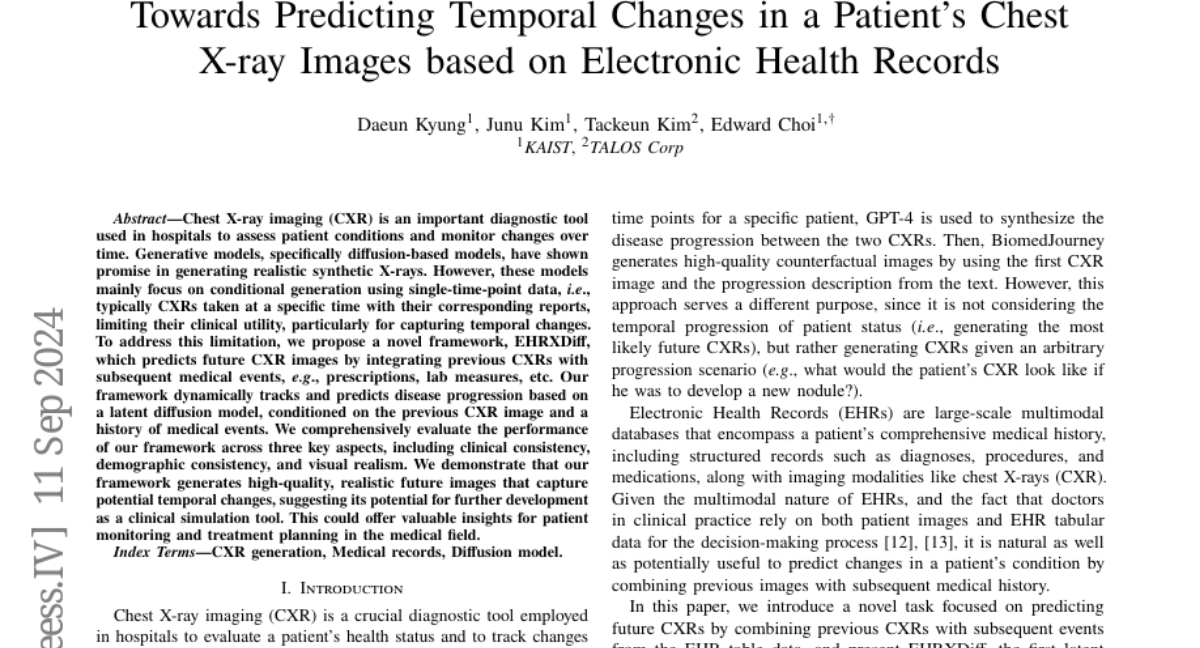
Summary
This paper presents a new method called EHRXDiff, which predicts future chest X-ray images by combining previous X-ray images with a patient's medical history, helping doctors monitor disease progression.
What's the problem?
Chest X-rays are crucial for diagnosing and tracking patient health over time, but existing models mainly generate images based on single-time-point data. This limits their ability to show how a patient's condition changes, making it harder for doctors to make informed decisions about treatment.
What's the solution?
The researchers developed EHRXDiff, a framework that uses previous chest X-rays along with subsequent medical events (like prescriptions or lab results) to predict what future X-rays might look like. This approach allows for a better understanding of how diseases progress over time. They tested the framework on three key aspects: clinical consistency, demographic consistency, and visual realism, and found that it generates high-quality images that reflect potential changes in a patient's condition.
Why it matters?
This research is important because it could significantly improve how doctors monitor and plan treatments for patients. By providing more accurate predictions of how conditions may evolve, EHRXDiff can enhance patient care and lead to better health outcomes.
Abstract
Chest X-ray imaging (CXR) is an important diagnostic tool used in hospitals to assess patient conditions and monitor changes over time. Generative models, specifically diffusion-based models, have shown promise in generating realistic synthetic X-rays. However, these models mainly focus on conditional generation using single-time-point data, i.e., typically CXRs taken at a specific time with their corresponding reports, limiting their clinical utility, particularly for capturing temporal changes. To address this limitation, we propose a novel framework, EHRXDiff, which predicts future CXR images by integrating previous CXRs with subsequent medical events, e.g., prescriptions, lab measures, etc. Our framework dynamically tracks and predicts disease progression based on a latent diffusion model, conditioned on the previous CXR image and a history of medical events. We comprehensively evaluate the performance of our framework across three key aspects, including clinical consistency, demographic consistency, and visual realism. We demonstrate that our framework generates high-quality, realistic future images that capture potential temporal changes, suggesting its potential for further development as a clinical simulation tool. This could offer valuable insights for patient monitoring and treatment planning in the medical field.