Towards Retrieval Augmented Generation over Large Video Libraries
Yannis Tevissen, Khalil Guetari, Frédéric Petitpont
2024-06-24
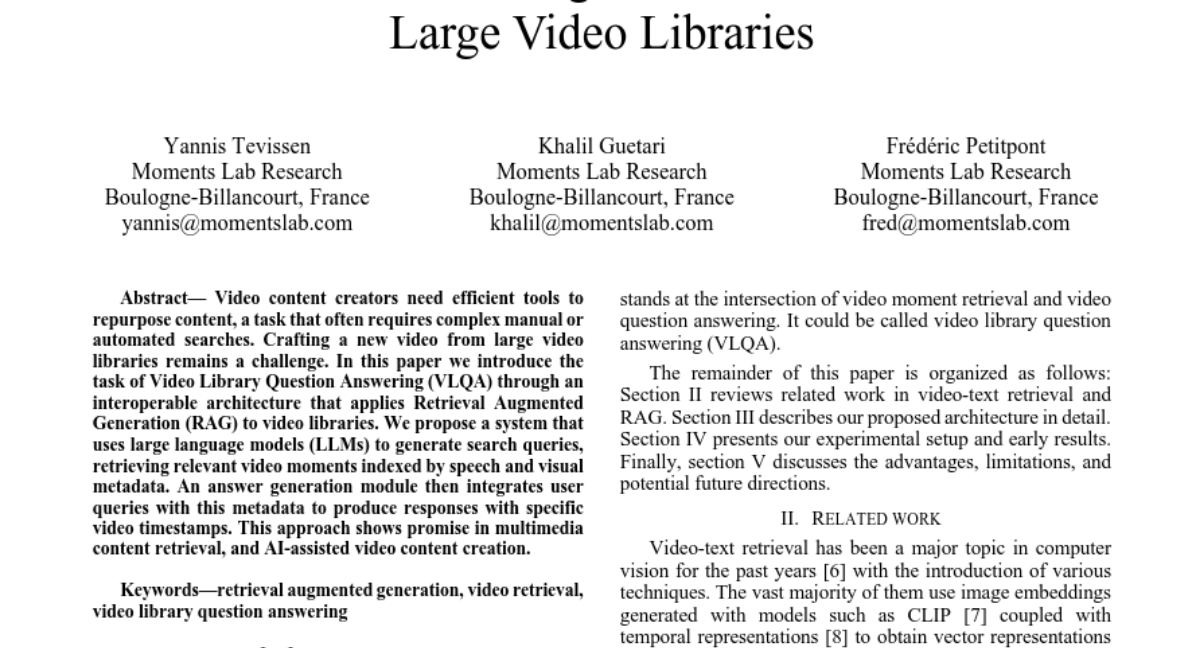
Summary
This paper introduces a new task called Video Library Question Answering (VLQA), which aims to help video content creators efficiently find and use clips from large video libraries by using advanced AI techniques.
What's the problem?
Video content creators often face challenges when trying to repurpose existing videos from large libraries. Searching for the right clips can be complicated and time-consuming, whether done manually or through automated systems. This makes it difficult to create new videos quickly and effectively, especially when dealing with vast amounts of footage.
What's the solution?
The authors propose a system that combines Retrieval Augmented Generation (RAG) with large language models (LLMs) to improve how video content is retrieved and used. This system generates search queries based on user questions, allowing it to find relevant video moments that are indexed with both speech and visual information. Once the relevant clips are identified, an answer generation module combines the user’s query with the metadata from these clips to produce responses that include specific timestamps for where the information can be found in the videos. This method streamlines the process of finding and repurposing video content.
Why it matters?
This research is important because it provides a more efficient way for creators to access and utilize large video libraries. By improving how videos are searched and retrieved, this system can save time and effort in content creation, making it easier for filmmakers, educators, and other professionals to produce high-quality videos. As video content continues to grow in importance across various fields, tools like this can significantly enhance productivity and creativity.
Abstract
Video content creators need efficient tools to repurpose content, a task that often requires complex manual or automated searches. Crafting a new video from large video libraries remains a challenge. In this paper we introduce the task of Video Library Question Answering (VLQA) through an interoperable architecture that applies Retrieval Augmented Generation (RAG) to video libraries. We propose a system that uses large language models (LLMs) to generate search queries, retrieving relevant video moments indexed by speech and visual metadata. An answer generation module then integrates user queries with this metadata to produce responses with specific video timestamps. This approach shows promise in multimedia content retrieval, and AI-assisted video content creation.