Towards Robust Speech Representation Learning for Thousands of Languages
William Chen, Wangyou Zhang, Yifan Peng, Xinjian Li, Jinchuan Tian, Jiatong Shi, Xuankai Chang, Soumi Maiti, Karen Livescu, Shinji Watanabe
2024-07-02
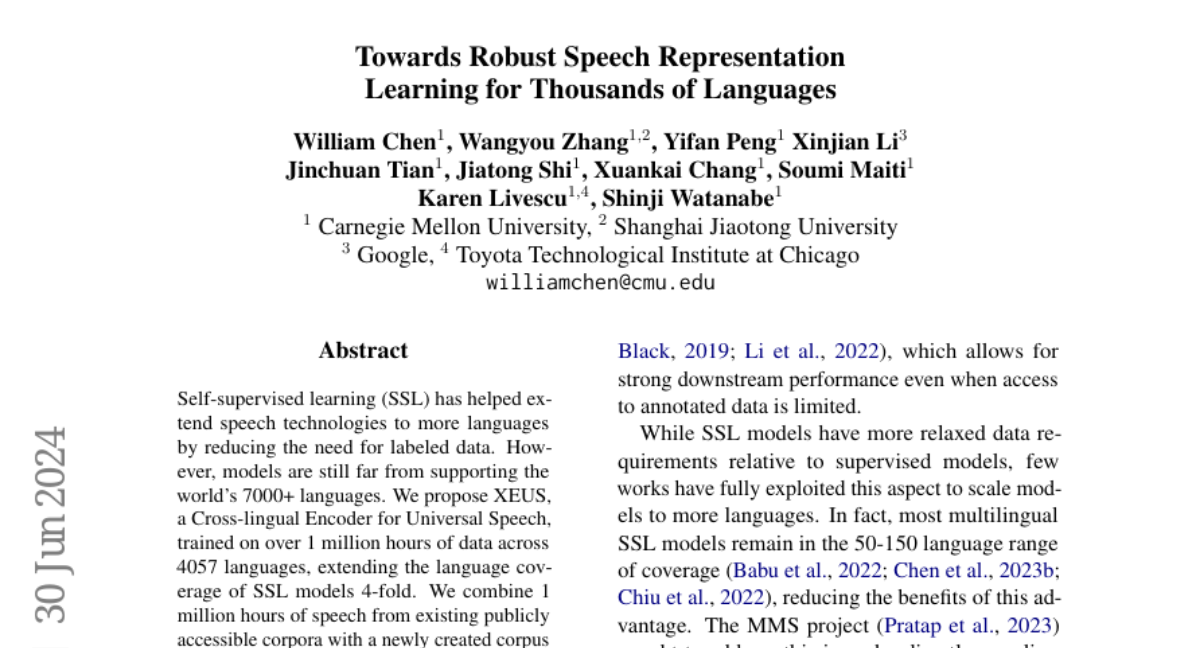
Summary
This paper talks about XEUS, a new model designed to improve how speech recognition technology works across many different languages. It uses self-supervised learning to help the model learn from a huge amount of speech data without needing a lot of labeled examples.
What's the problem?
Although speech technology has advanced, it still struggles to support the over 7,000 languages spoken worldwide. Most existing models rely on labeled data, which is time-consuming and expensive to create. This limits their ability to understand and process less common languages effectively.
What's the solution?
To tackle this issue, the authors developed XEUS, a Cross-lingual Encoder that has been trained on over 1 million hours of speech data from 4,057 languages. This includes both existing public datasets and a new collection of over 7,400 hours of speech that will be made available for others to use. XEUS enhances traditional self-supervised learning methods by adding a new objective that helps the model better handle different audio conditions, like echoes. The authors tested XEUS on various benchmarks and found that it consistently performs better than or as well as other leading models, even with fewer parameters.
Why it matters?
This research is important because it significantly expands the capabilities of speech recognition technology to include more languages, making it more inclusive and accessible. By improving how models learn from diverse speech data, XEUS can help bridge language barriers in technology, enabling better communication and understanding across cultures. This advancement could have a major impact in areas such as education, international business, and global communication.
Abstract
Self-supervised learning (SSL) has helped extend speech technologies to more languages by reducing the need for labeled data. However, models are still far from supporting the world's 7000+ languages. We propose XEUS, a Cross-lingual Encoder for Universal Speech, trained on over 1 million hours of data across 4057 languages, extending the language coverage of SSL models 4-fold. We combine 1 million hours of speech from existing publicly accessible corpora with a newly created corpus of 7400+ hours from 4057 languages, which will be publicly released. To handle the diverse conditions of multilingual speech data, we augment the typical SSL masked prediction approach with a novel dereverberation objective, increasing robustness. We evaluate XEUS on several benchmarks, and show that it consistently outperforms or achieves comparable results to state-of-the-art (SOTA) SSL models across a variety of tasks. XEUS sets a new SOTA on the ML-SUPERB benchmark: it outperforms MMS 1B and w2v-BERT 2.0 v2 by 0.8% and 4.4% respectively, despite having less parameters or pre-training data. Checkpoints, code, and data are found in https://www.wavlab.org/activities/2024/xeus/.