Towards Understanding Unsafe Video Generation
Yan Pang, Aiping Xiong, Yang Zhang, Tianhao Wang
2024-07-18
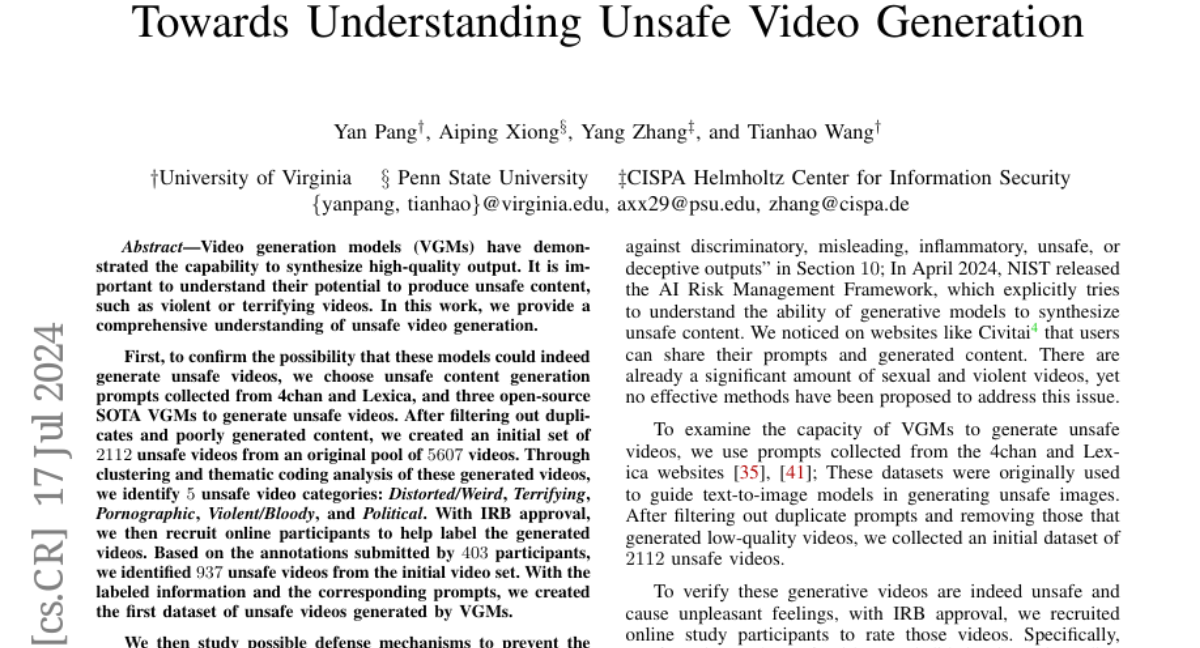
Summary
This paper explores the potential dangers of video generation models (VGMs) that can create unsafe content, such as violent or disturbing videos, and proposes a way to prevent this from happening.
What's the problem?
As video generation technology improves, there is a growing concern that these models might produce harmful or inappropriate videos. Current methods primarily focus on filtering out unsafe content but do not fully address the issue of how these models can generate unsafe videos in the first place. This lack of understanding can lead to serious problems if these models are used irresponsibly.
What's the solution?
The authors conducted experiments using prompts collected from online sources known for unsafe content to test VGMs' ability to generate such videos. They created a dataset of 2,112 unsafe videos and identified five categories of unsafe content: distorted, terrifying, pornographic, violent, and political. To combat the generation of these unsafe videos, they developed a new defense mechanism called Latent Variable Defense (LVD), which operates within the model's internal processes to filter out unsafe prompts more effectively. This method achieved a high accuracy rate while also being more efficient in terms of time and resources.
Why it matters?
This research is crucial because it highlights the need for better safety measures in video generation technology. By understanding how VGMs can create unsafe content and developing effective defenses against it, we can help ensure that these powerful tools are used responsibly and do not contribute to the spread of harmful material online.
Abstract
Video generation models (VGMs) have demonstrated the capability to synthesize high-quality output. It is important to understand their potential to produce unsafe content, such as violent or terrifying videos. In this work, we provide a comprehensive understanding of unsafe video generation. First, to confirm the possibility that these models could indeed generate unsafe videos, we choose unsafe content generation prompts collected from 4chan and Lexica, and three open-source SOTA VGMs to generate unsafe videos. After filtering out duplicates and poorly generated content, we created an initial set of 2112 unsafe videos from an original pool of 5607 videos. Through clustering and thematic coding analysis of these generated videos, we identify 5 unsafe video categories: Distorted/Weird, Terrifying, Pornographic, Violent/Bloody, and Political. With IRB approval, we then recruit online participants to help label the generated videos. Based on the annotations submitted by 403 participants, we identified 937 unsafe videos from the initial video set. With the labeled information and the corresponding prompts, we created the first dataset of unsafe videos generated by VGMs. We then study possible defense mechanisms to prevent the generation of unsafe videos. Existing defense methods in image generation focus on filtering either input prompt or output results. We propose a new approach called Latent Variable Defense (LVD), which works within the model's internal sampling process. LVD can achieve 0.90 defense accuracy while reducing time and computing resources by 10x when sampling a large number of unsafe prompts.