Towards Visual Text Grounding of Multimodal Large Language Model
Ming Li, Ruiyi Zhang, Jian Chen, Jiuxiang Gu, Yufan Zhou, Franck Dernoncourt, Wanrong Zhu, Tianyi Zhou, Tong Sun
2025-04-11
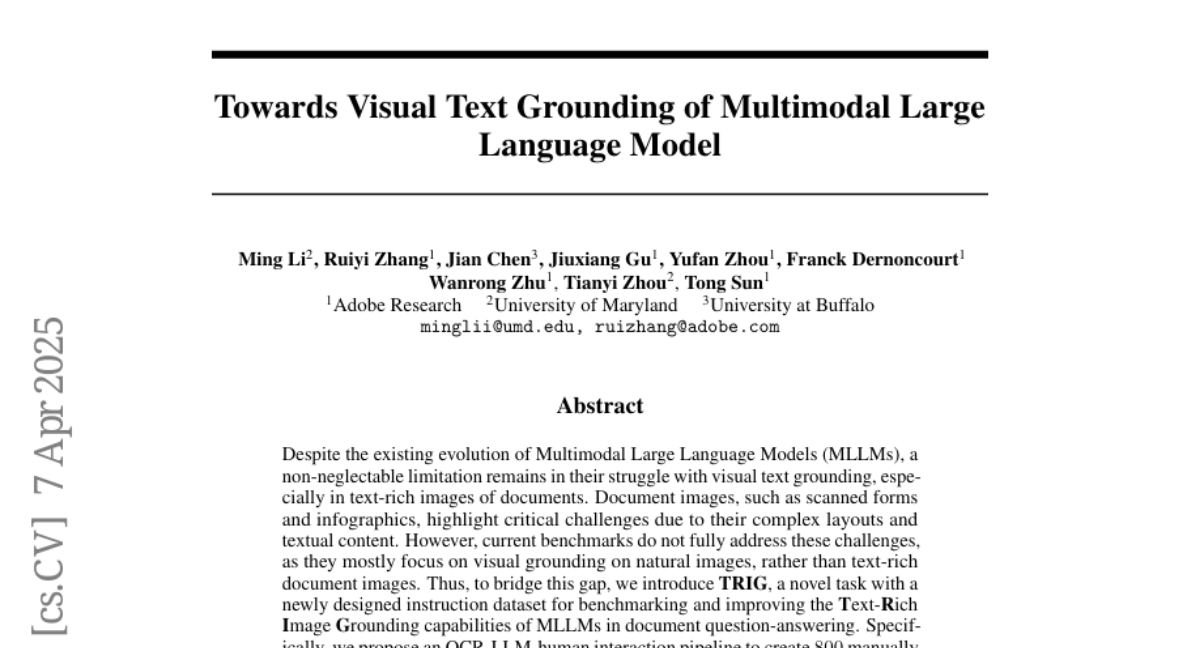
Summary
This paper talks about teaching AI to better understand and point out specific text areas in complex document images like forms or charts, where words and layouts are mixed together.
What's the problem?
Current AI struggles to accurately link text answers to their exact locations in cluttered document images, leading to mistakes in tasks like form analysis or chart understanding.
What's the solution?
The researchers created a new test (TRIG) with 800 real examples and 90k synthetic ones, using OCR tools and AI helpers to map questions to text locations, then trained models to focus on these connections.
Why it matters?
This helps AI assistants reliably answer questions about documents (like tax forms or infographics) while showing exactly where the info is, reducing errors and improving trust in automated systems.
Abstract
Despite the existing evolution of Multimodal Large Language Models (MLLMs), a non-neglectable limitation remains in their struggle with visual text grounding, especially in text-rich images of documents. Document images, such as scanned forms and infographics, highlight critical challenges due to their complex layouts and textual content. However, current benchmarks do not fully address these challenges, as they mostly focus on visual grounding on natural images, rather than text-rich document images. Thus, to bridge this gap, we introduce TRIG, a novel task with a newly designed instruction dataset for benchmarking and improving the Text-Rich Image Grounding capabilities of MLLMs in document question-answering. Specifically, we propose an OCR-LLM-human interaction pipeline to create 800 manually annotated question-answer pairs as a benchmark and a large-scale training set of 90$ synthetic data based on four diverse datasets. A comprehensive evaluation of various MLLMs on our proposed benchmark exposes substantial limitations in their grounding capability on text-rich images. In addition, we propose two simple and effective TRIG methods based on general instruction tuning and plug-and-play efficient embedding, respectively. By finetuning MLLMs on our synthetic dataset, they promisingly improve spatial reasoning and grounding capabilities.