Toxicity of the Commons: Curating Open-Source Pre-Training Data
Catherine Arnett, Eliot Jones, Ivan P. Yamshchikov, Pierre-Carl Langlais
2024-10-31
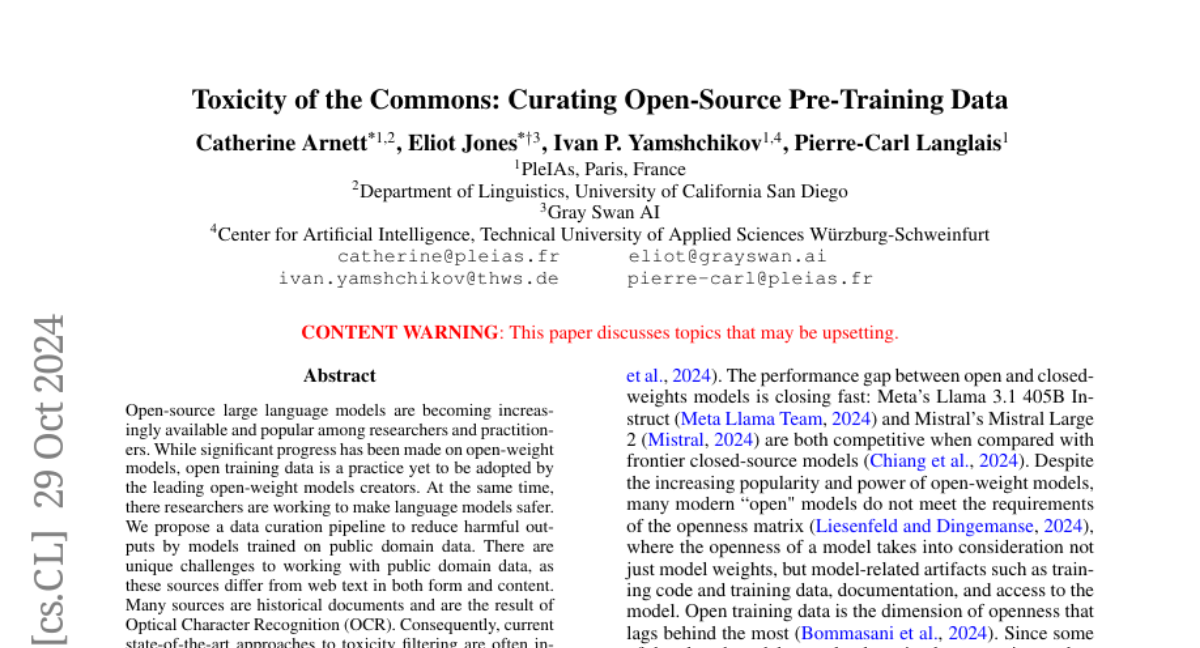
Summary
This paper discusses a new approach to curating open-source training data for language models to reduce harmful outputs by filtering out toxic content.
What's the problem?
As large language models become more popular, there is a growing need for safe and effective training data. However, many existing datasets contain harmful or toxic content, which can lead to these models generating inappropriate or offensive outputs. Current methods for filtering such content often do not work well with public domain data, which can include historical documents and texts that have been scanned and converted using Optical Character Recognition (OCR). This makes it challenging to create a safe training environment for these models.
What's the solution?
The authors propose a new data curation pipeline called ToxicCommons, which includes a custom dataset that classifies texts based on five types of discrimination (like racial or gender-based) and violence. They also developed a classifier named Celadon that can efficiently detect toxic content in open-source datasets. This approach allows for better filtering of harmful material while still providing enough diverse data for training the models effectively.
Why it matters?
This research is important because it helps improve the safety of open-source language models by ensuring they are trained on cleaner, less harmful data. By creating better tools for filtering toxicity, the study contributes to the development of more responsible AI systems that can be used safely in various applications, including education, customer service, and content creation.
Abstract
Open-source large language models are becoming increasingly available and popular among researchers and practitioners. While significant progress has been made on open-weight models, open training data is a practice yet to be adopted by the leading open-weight models creators. At the same time, there researchers are working to make language models safer. We propose a data curation pipeline to reduce harmful outputs by models trained on public domain data. There are unique challenges to working with public domain data, as these sources differ from web text in both form and content. Many sources are historical documents and are the result of Optical Character Recognition (OCR). Consequently, current state-of-the-art approaches to toxicity filtering are often infeasible or inappropriate for open data models. In this paper, we introduce a new fully open-source pipeline for open-data toxicity filtering. Our contributions are threefold. We create a custom training dataset, ToxicCommons, which is composed of texts which have been classified across five different dimensions (racial/origin-based, gender/sex-based, religious, ability-based discrimination, and violence). We use this dataset to train a custom classifier, Celadon, that can be used to detect toxic content in open data more efficiently at a larger scale. Finally, we describe the balanced approach to content filtration that optimizes safety filtering with respect to the filtered data available for training.