TRACE: Temporal Grounding Video LLM via Causal Event Modeling
Yongxin Guo, Jingyu Liu, Mingda Li, Xiaoying Tang, Qingbin Liu, Xi Chen
2024-10-10
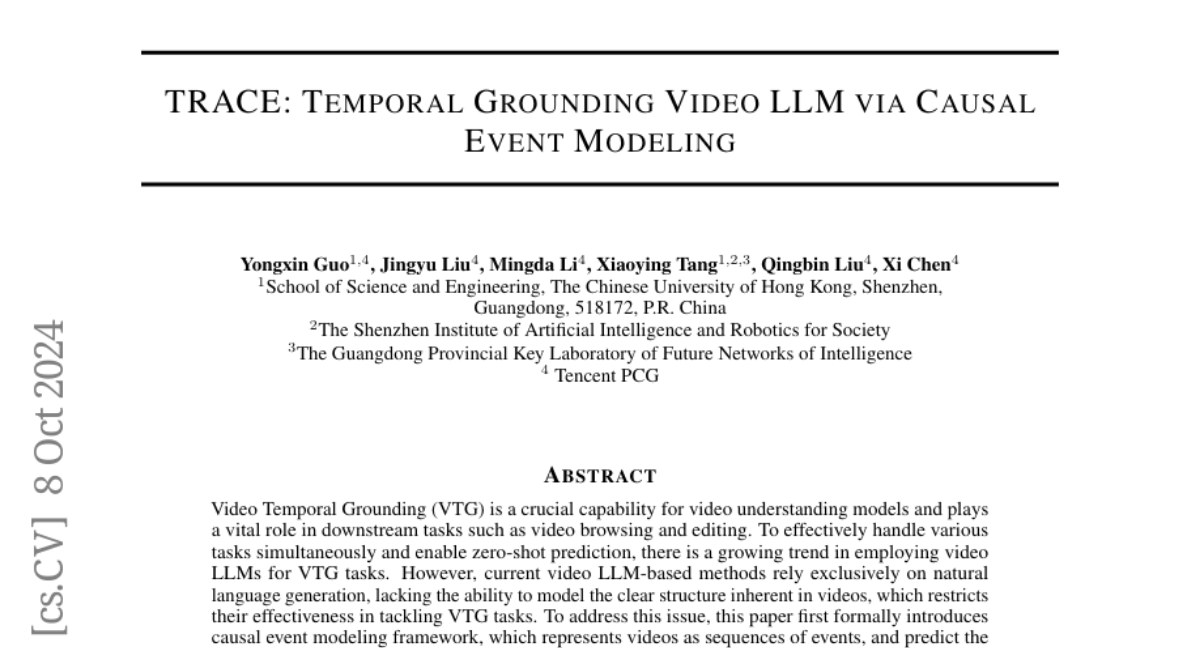
Summary
This paper introduces TRACE, a new method for improving how video understanding models can identify and reason about events in videos over time.
What's the problem?
Video understanding models, especially those that use large language models (LLMs), struggle to accurately track and understand the sequence of events in videos. Current methods mainly focus on generating text descriptions without effectively capturing the structure and timing of events, which limits their ability to perform tasks like video browsing and editing.
What's the solution?
To tackle this issue, the authors developed a framework called causal event modeling, which breaks down videos into sequences of events. Each event is defined by its timing, importance, and a text description. They then created TRACE, a task-interleaved video LLM that processes visual frames, timestamps, and event descriptions as separate tasks. This allows the model to better understand the relationships between events and improve its performance on various video tasks.
Why it matters?
This research is important because it enhances the capabilities of video understanding models, making them more effective for practical applications like video editing and content retrieval. By improving how these models handle temporal reasoning, TRACE can lead to better user experiences in navigating and interacting with video content.
Abstract
Video Temporal Grounding (VTG) is a crucial capability for video understanding models and plays a vital role in downstream tasks such as video browsing and editing. To effectively handle various tasks simultaneously and enable zero-shot prediction, there is a growing trend in employing video LLMs for VTG tasks. However, current video LLM-based methods rely exclusively on natural language generation, lacking the ability to model the clear structure inherent in videos, which restricts their effectiveness in tackling VTG tasks. To address this issue, this paper first formally introduces causal event modeling framework, which represents videos as sequences of events, and predict the current event using previous events, video inputs, and textural instructions. Each event consists of three components: timestamps, salient scores, and textual captions. We then propose a novel task-interleaved video LLM called TRACE to effectively implement the causal event modeling framework in practice. The TRACE processes visual frames, timestamps, salient scores, and text as distinct tasks, employing various encoders and decoding heads for each. Task tokens are arranged in an interleaved sequence according to the causal event modeling framework's formulation. Extensive experiments on various VTG tasks and datasets demonstrate the superior performance of TRACE compared to state-of-the-art video LLMs. Our model and code are available at https://github.com/gyxxyg/TRACE.